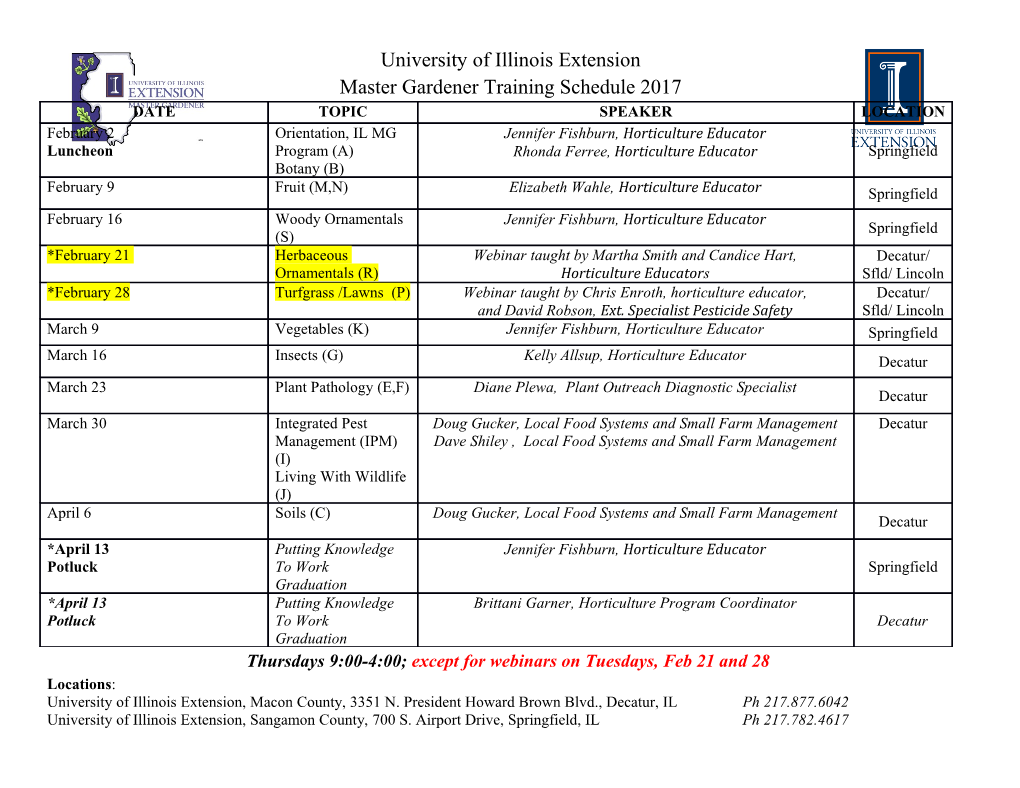
Journal of Statistical Theory and Applications Volume 10, Number 4, 2011, pp. 553-569 ISSN 1538-7887 Comparison of Wald, Score, and Likelihood Ratio Tests for Response Adaptive Designs Yanqing Yi1∗and Xikui Wang2 1 Division of Community Health and Humanities, Faculty of Medicine, Memorial University of Newfoundland, St. Johns, Newfoundland, Canada A1B 3V6 2 Department of Statistics, University of Manitoba, Winnipeg, Manitoba, Canada R3T 2N2 Abstract Data collected from response adaptive designs are dependent. Traditional statistical methods need to be justified for the use in response adaptive designs. This paper gener- alizes the Rao's score test to response adaptive designs and introduces a generalized score statistic. Simulation is conducted to compare the statistical powers of the Wald, the score, the generalized score and the likelihood ratio statistics. The overall statistical power of the Wald statistic is better than the score, the generalized score and the likelihood ratio statistics for small to medium sample sizes. The score statistic does not show good sample properties for adaptive designs and the generalized score statistic is better than the score statistic under the adaptive designs considered. When the sample size becomes large, the statistical power is similar for the Wald, the sore, the generalized score and the likelihood ratio test statistics. MSC: 62L05, 62F03 Keywords and Phrases: Response adaptive design, likelihood ratio test, maximum likelihood estimation, Rao's score test, statistical power, the Wald test ∗Corresponding author. Fax: 1-709-777-7382. E-mail addresses: [email protected] (Yanqing Yi), xikui [email protected] (Xikui Wang) Y. Yi and X. Wang 554 1. Introduction The Wald, Rao's score and likelihood ratio tests are regarded as the Holy Trinity in asymp- totic statistics. These tests are first-order equivalent and asymptotically optimal, however they differ in small samples and in second-order properties under certain conditions. The likelihood ratio test was introduced by Neyman and Pearson (1928), the Wald test by Wald (1943) and the score test by Rao (1948). Aitchison and Silvey (1958) and Silvey (1959) derived the La- grangian Multiplier (LM) test independently of the score test, however the LM and score tests are equivalent. Neyman's C(α) test (Neyman 1959, 1979) may be regarded as a conditional Rao's score test (Bera and Bilias, 2001). Bera and Bilias (2001) provided historical perspectives of the Rao's score test, Silvey's LM test and Neyman's C(α) test. Expository studies of the Wald, score and likelihood ratio tests are given in Buse (1982) and Rayner (1997). Engle (1984) provided review on these tests and Ghosh (1991) reviewed the higher-order statistical power performance of these test statistics. Comparisons of these tests are given in Rao (2005) with respect to their merits and defects, in Molenberghs and Verbeke (2007) in a constrained parameter space, in Sutradhar and Bartlett (1993) by monte carlo simulation, in Li (2001) on the sensitivity to nuisance parameters, and Chandra and Joshi (1983), Chandra and Mukerjee (1985), and Mukerjee (1990a, 1990b) under contiguous alternatives. Furthermore, Rao and Mukerjee (1997) and Taniguchi (1991) compared these tests in a possibly non-iid set- up. Ghosh and Mukerjee (2001) considered the higher-order asymptotic of statistical power for a large class of test statistics including the Wald, score and likelihood ratio statistics based on quasi likelihood. However their assumptions do not apply to data from response adaptive clinical trials. Response adaptive designs of clinical trials use accruing information to improve the efficacy and ethics of the clinical trials without undermining the validity and integrity of the clinical research. In response adaptive designs the randomization probability of treatment allocation is sequentially modified depending on information so far accumulated in the trial. Consequently treatment allocation is deliberately biased in order to assign more patients to the potentially superior treatment while a valid statistical comparison of the alternative treatments is still feasible at the conclusion of the study. However due to the particular dependence structure in data collected from response adaptive trials, the statistical comparison of treatment effectiveness Wald, Score, and Likelihood Ratio Tests 555 is non-traditional. The Wald and likelihood ratio tests have been extended to analyze data from response adaptive designs. Hu and Rosenberger (2003) analyzed the statistical power based on the Wald test and found that the power is a decreasing function of the variance of allocation proportion. Rosenberger et al (2001) used the Wald statistic to analyze power and proposed an optimal adaptive design (namely the RSIHR design) which optimally balances the expected number of failures and the statistical power of the test. Ivanova (2003) introduced the drop-the-loser design (namely the DL design) and used the Wald statistic to compare the statistical power under the DL design with other designs. Yi and Wang (2009) also proposed a design (namely the YW design) and compared the statistical power of the Wald test under different adaptive designs. The likelihood ratio test was applied to the birth and death urn design in Ivanova et al (2000). Yi and Wang (2007) justified the use of the likelihood ratio test for a general class of response adaptive designs. The Wald and likelihood ratio tests are based on the usual likelihood and the maximum likelihood estimators are used in these statistics. The Wald, score and likelihood test statistics have been generalized based on quasi-likelihood functions. Ghosh and Mukerjee (2001) generalized these test statistics to quasi-likelihood set- tings and considered high-order statistical power of these statistics. Taniguchi (1991) compared high-order statistical power of these statistics in a general setting including iid and time series data. Heyde (1997) introduced a general framework to obtain optimal parameter estimation by using quasi-likelihood functions. The assumptions for high-order statistical power of these test statistics are not satisfied by the data collected from response adaptive designs. For the esti- mation for response adaptive designs, Coad and Woodroofe (1998) investigated the bias of the maximum likelihood estimator for sequential clinical trials. Coad and Ivanova (2001) proposed the bias-corrected estimators for response adaptive designs. Yi and Wang (2008) proved that the maximum likelihood estimators are efficient in the Bahadur sense. This paper uses the usual likelihood function and the maximum likelihood estimators in the Wald, score and likelihood ratio statistics. The results in this paper can be generalized to quasi-likelihood settings. With a response adaptive design, the adaptation of the treatment allocation introduces more variation in the data and results in a loss of statistical power. Most of the research on statistical power for response adaptive designs are based on the Wald test statistic. The comparison of the statistical powers of the Wald, score and likelihood ratio tests has not been conducted for Y. Yi and X. Wang 556 response adaptive designs. It is well known that the statistical power performance of the Wald test is not satisfactory and the score test performs well when sample sizes are small for iid data. However it is unclear whether the small sample performance of the score test remains true for data collected from response adaptive designs. This paper generalizes the score test to response adaptive designs and compares the performance of statistical power of the Wald, score and likelihood ratio tests for response adaptive designs. Considering the variability in test statistics added by adaptive designs, the sensitivity of these tests to the type of design is also explored. The paper is organized as follows. Section 2 introduces necessary assumptions and the asymptotic distributions of the Wald, score and likelihood ratio tests under the null hypothesis and under contiguous alternatives. Section 3 presents simulation results to compare statistical powers of these tests under different response adaptive designs. An application of these statistics to real data is included in Section 4. Section 5 concludes the paper. 2. Formulation of the problem Suppose patients arrive sequentially in the trial and each patient receives one and only one of k treatments, k ≥ 2. Patients' responses Y1j;Y2j; ··· ; from treatment j; j = 1; ··· ; k; are independent and identically distributed with a density function fj(y; θj); j = 1; ··· ; k: We T assume that θ = (θ1; θ2; ··· ; θk) 2 Θ is an unknown parameter, where T stands for transpose. th Let δi = (δi1; δi2; ··· ; δik) be the treatment assignment to the i patients such that δij = 1 th if the i patient receives treatment j and δij = 0 otherwise, and yi = (Yi1δi1;Yi2δi2; ··· ;Yikδik) be the corresponding response. Here we use the convention that if treatment j is not applied to patient i, then the response is 0 from treatment j. When the ith patient, i ≥ 2, is to be treated, the information available is given by the σ algebra Fi−1 generated by f(δ1; y1); ··· ; (δi−1; yi−1)g. Pn After n patients have been treated in the trial, let Nj(n) = i=1 δij; j = 1; 2; ··· ; k; be the number of patients receiving treatment j. For simplicity, denote Nj(n) as Nj: For response adaptive designs, assume Nj (A) As n ! 1, we have n ! vj(θ) 2 (0; 1) almost surely for every θ 2 Θ and Nj(n) ! 1 almost surely, where vj(θ) is a continuous function of θ; j = 1; 2; ··· ; k; representing the desired allocation proportion to treatment j. Wald, Score, and Likelihood Ratio Tests 557 This assumption holds true for a number of response adaptive designs, including the ran- domized play-the-winner design, the optimal design proposed by Rosenberger et al. (2001), and the allocation rule in Melfi et al. (2001). A randomized response adaptive allocation rule π = fπi; i = 1; 2; · · · g consists of a sequence Pk of conditional probabilities πij = P (δij = 1jFi−1), j=1 πij = 1, i ≥ 2, and the initial possibly randomized treatment allocation probabilities π1j = P (δ1j = 1) are pre-fixed (such as 1=k), 1 ≤ j ≤ k.
Details
-
File Typepdf
-
Upload Time-
-
Content LanguagesEnglish
-
Upload UserAnonymous/Not logged-in
-
File Pages17 Page
-
File Size-