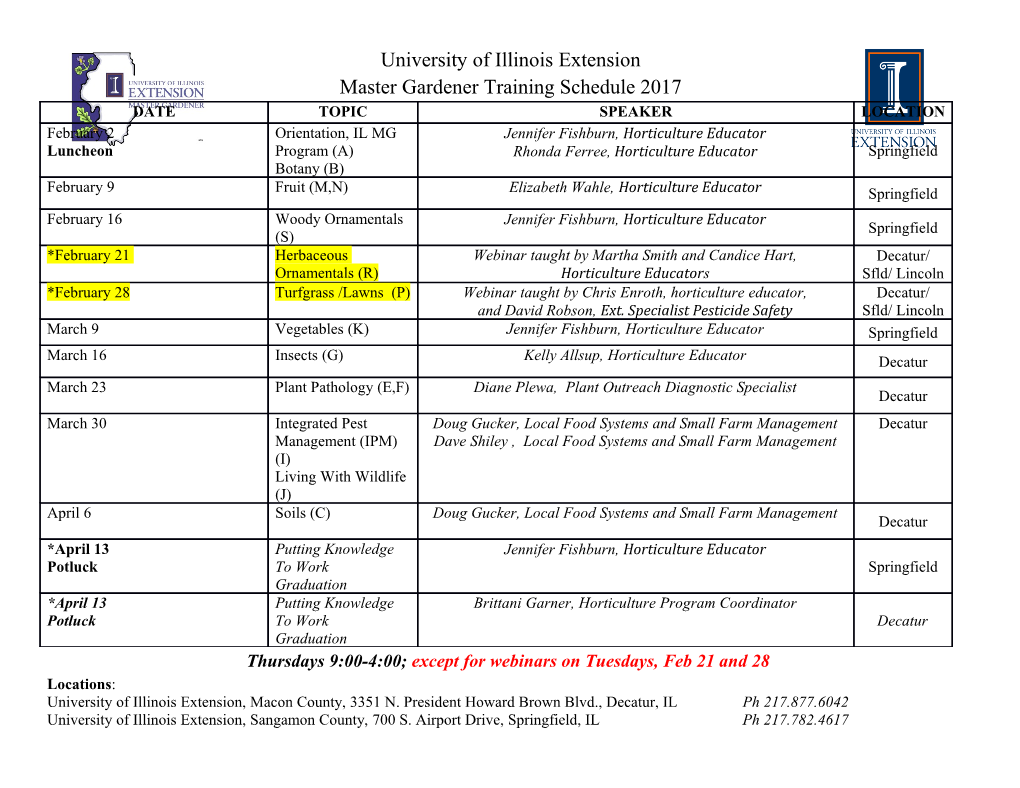
Journal of the American Statistical Association ISSN: 0162-1459 (Print) 1537-274X (Online) Journal homepage: https://www.tandfonline.com/loi/uasa20 Modeling Motor Learning Using Heteroscedastic Functional Principal Components Analysis Daniel Backenroth, Jeff Goldsmith, Michelle D. Harran, Juan C. Cortes, John W. Krakauer & Tomoko Kitago To cite this article: Daniel Backenroth, Jeff Goldsmith, Michelle D. Harran, Juan C. Cortes, John W. Krakauer & Tomoko Kitago (2018) Modeling Motor Learning Using Heteroscedastic Functional Principal Components Analysis, Journal of the American Statistical Association, 113:523, 1003-1015, DOI: 10.1080/01621459.2017.1379403 To link to this article: https://doi.org/10.1080/01621459.2017.1379403 View supplementary material Accepted author version posted online: 29 Sep 2017. Published online: 29 Sep 2017. Submit your article to this journal Article views: 513 View Crossmark data Full Terms & Conditions of access and use can be found at https://www.tandfonline.com/action/journalInformation?journalCode=uasa20 JOURNAL OF THE AMERICAN STATISTICAL ASSOCIATION , VOL. , NO. , –: Applications and Case Studies https://doi.org/./.. Modeling Motor Learning Using Heteroscedastic Functional Principal Components Analysis Daniel Backenrotha, Jeff Goldsmitha, Michelle D. Harranb,c,JuanC.Cortesb,d, John W. Krakauerb,c,d, and Tomoko Kitagob,e aDepartment of Biostatistics, Mailman School of Public Health, Columbia University, New York, NY; bDepartment of Neurology, Columbia University Medical Center, New York, NY; cDepartment of Neuroscience, Johns Hopkins University School of Medicine, Baltimore, MD; dDepartment of Neurology, Johns Hopkins University School of Medicine, Baltimore, MD; eBurke Neurological Institute, White Plains, NY ABSTRACT ARTICLE HISTORY We propose a novel method for estimating population-level and subject-specific effects of covariates on the Received February variability of functional data. We extend the functional principal components analysis framework by mod- Revised August eling the variance of principal component scores as a function of covariates and subject-specific random KEYWORDS effects. In a setting where principal components are largely invariant across subjects and covariate values, Functional data; Kinematic modeling the variance of these scores provides a flexible and interpretable way to explore factors that affect data; Motor control; the variability of functional data. Our work is motivated by a novel dataset from an experiment assessing Probabilistic PCA; Variance upper extremity motor control, and quantifies the reduction in movement variability associated with skill modeling; Variational Bayes learning. The proposed methods can be applied broadly to understand movement variability, in settings that include motor learning, impairment due to injury or disease, and recovery. Supplementary materials for this article are available online. 1. Scientific Motivation variance and recovery (Krakauer 2006). By taking full advantage of high-frequency laboratory recordings, we shift focus from 1.1. Motor Learning particular time points to complete curves. Our approach allows us to model the variability of these curves as they depend on Recent work in motor learning has suggested that change in covariates,likethehandusedortherepetitionnumber,aswell movement variability is an important component of improve- as the estimation of random effects reflecting differences in base- ment in motor skill. It has been suggested that when a motor line variability and learning rates among subjects. task is learned, variance is reduced along dimensions relevant Section 1.2 describes our motivating data in more detail, and to the successful accomplishment of the task, although it may Section 2 introduces our modeling framework. A review of rele- increase in other dimensions (Scholz and Schöner 1999;Yarrow, vantstatisticalworkappearsinSection 3.Detailsofourestima- Brown, and Krakauer 2009). Experimental work, moreover, tion approach are in Section 4. Simulations and the application has shown that learning-induced improvement of movement to our motivating data appear in Sections 5 and 6,respectively, execution, measured through the trade-off between speed and andweclosewithadiscussioninSection 7. accuracy, is accompanied by significant reductions in movement variability. In fact, these reductions in movement variability may be a more important feature of learning than changes in the 1.2. Dataset average movement (Shmuelof, Krakauer, and Mazzoni 2012). These results have typically been based on assessments of vari- Our motivating data were gathered as part of a study of motor ability at a few time points, for example, at the end of the move- learning among healthy subjects. Kinematic data were acquired ment, although high-frequency laboratory recordings of com- in a standard task used to measure control of reaching move- plete movements are often available. ments. In this task, subjects rest their forearm on an air-sled In this article we develop a modeling framework that can be system to reduce effects of friction and gravity. The subjects are used to quantify movement variability based on dense record- presented with a screen showing eight targets arranged in a cir- ings of fingertip position throughout movement execution. This cle around a starting point, and they reach with their arm to a framework can be used to explore many aspects of motor skill target and back when it is illuminated on the screen. Subjects’ and learning: differences in baseline skill among healthy sub- movements are displayed on the screen, and they are rewarded jects, effects of repetition and training to modulate variability with 10 points if they turn their hand around within the target, over time, or the effect of baseline stroke severity on movement and 3 or 1 otherwise, depending on how far their hand is from CONTACT Daniel Backenroth [email protected] Department of Biostatistics, Mailman School of Public Health, Columbia University, West th Street, th Floor, New York, NY . Color versions of one or more of the figures in the article can be found online at www.tandfonline.com/r/JASA. Supplementary materials for this article are available online. Please go to www.tandfonline.com/r/JASA. © American Statistical Association 1004 D. BACKENROTH ET AL. thetargetatthepointofreturn.Subjectsarenotrewardedfor each target by each subject. The reduction in movement vari- movements outside prespecified velocity thresholds. ability after practice is clear. Our dataset consists of 9481 movements by 26 right-handed Prior to our analyses, we rotate curves so that all movements subjects. After becoming familiarized with the experimental extend to the target at 0◦. This rotation preserves shape and apparatus, each subject made 24 or 25 reaching movements to scale, but improves interpretation. After rotation, movement each of the 8 targets, in a semirandom order, with both the left along the X coordinate represents movement parallel to the line and right hand. Movements that did not reach at least 30% of the between origin and target, and movement along the Y coordi- distance to the target and movements with a direction more than nate represents movement perpendicular to this line. We build 90 deg away from the target direction at the point of peak veloc- models for X and Y coordinate curves separately in our primary ity were excluded from the dataset, because of the likelihood analysis. An alternative bivariate analysis appears in Appendix that they were made to the wrong target or not attempted due C. to distraction. Movements made at speeds outside the range of interest, with peak velocity less than 0.04 or greater than 2.0 m/s, 2. Model for Trajectory Variability were also excluded. These exclusion rules and other similar rules We adopt a functional data approach to model position curves have been used previously in similar kinematic experiments, and P (t).HereweomittheX andY superscripts for notational sim- are designed to increase the specificity of these experiments for ij plicity. Our starting point is the functional principal component probingmotorcontrolmechanisms(Huangetal.2012; Tanaka, analysis (FPCA) model of Yao, Müller, and Wang (2005)with Sejnowski, and Krakauer 2009;Kitagoetal.2015). A small num- subject-specific means. In this model, it is assumed that each ber of additional movements were removed from the dataset due curve P (t) can be modeled as to instrumentation and recording errors. The data we consider ij have not been previously reported. ( ) = μ ( ) + δ ( ) For each movement, the X and Y position of the hand move- Pij t ij t ij t ∞ ment is recorded as a function of time from movement onset to = μ ( ) + ξ φ ( ) + ( ). the initiation of return to the starting point, resulting in bivari- ij t ijk k t ij t (1) X ( ), Y ( ) k=1 ate functional observations denoted [Pij t Pij t ]forsubject i and movement j. In practice, observations are recorded not Here μij(t) is the mean function for curve Pij(t),thedevia- as functions but as discrete vectors. There is some variability in tion δij(t) is modeled as a linear combination of eigenfunctions φ ( ) ξ movement duration, which we remove for computational con- k t ,the ijk are uncorrelated random variables with mean 0 venience by linearly registering each movement onto a common and variances λk,where λk < ∞ and λ1 ≥ λ2 ≥··· , and = k grid of length D 50. The structure of the registered kinematic ij(t) iswhitenoise.Hereallthedeviationsδij(t) are assumed data is illustrated
Details
-
File Typepdf
-
Upload Time-
-
Content LanguagesEnglish
-
Upload UserAnonymous/Not logged-in
-
File Pages14 Page
-
File Size-