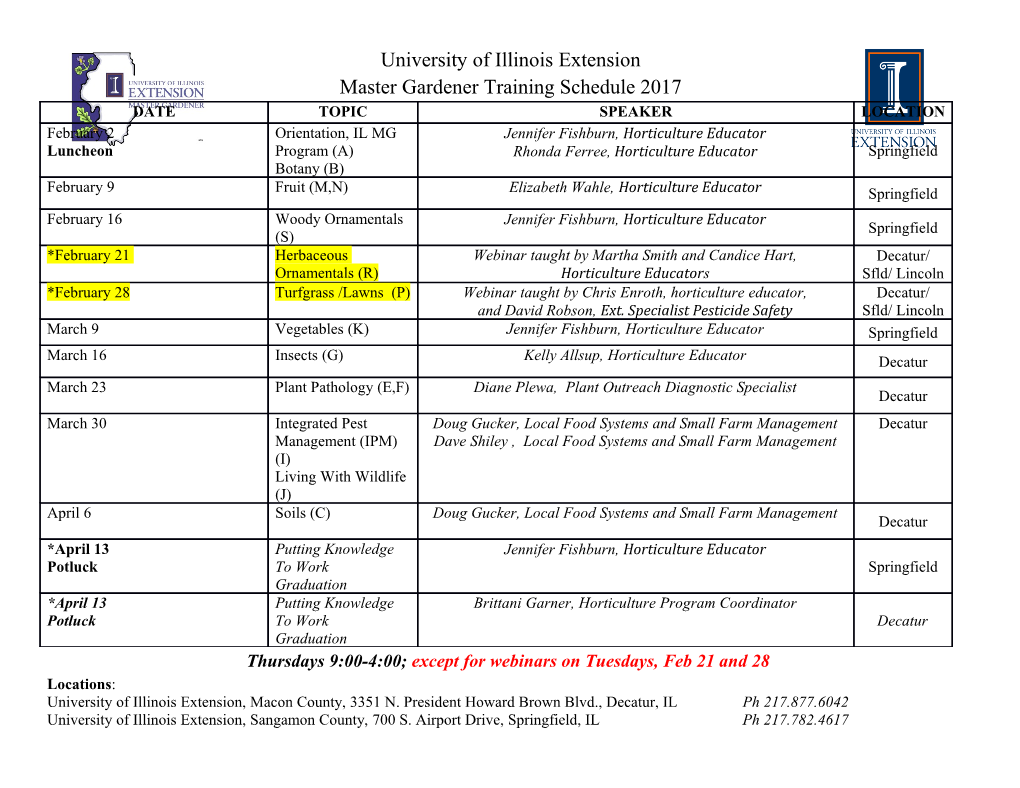
CHAPTER 3 UNDERSTANDING DISASTER RISK: HAZARD RELATED RISK ISSUES - SECTION III 3.8 Meteorological risk: extreme temperatures Glenn McGregor, Angie Bone, Florian Pappenberger 3.8.1 or as a threshold value recorded at a meteorological or climate station. Temperature Temperature extremes, extremes in although rare, are Temperature extremes can be ex- a disaster risk important from a DRM pressed as a probability of occur- rence, or as a return period (e.g. 5 % management context perspective as they probability or 1 in 20 year return pe- can lead to a range of riod). Occasionally, the term ‘return Understanding temperature extremes substantive direct and period’ is misinterpreted to mean an in a DRM context involves getting indirect impacts on event of a particular magnitude, so to know how often temperature ex- human activity and other that an event with a return period of 1 tremes occur, the conditions under systems. in 20 years, having once occurred, will which they occur and establishing occur again only after 20 years have associated direct and indirect societal passed. This is incorrect, as at any one impacts. time the occurrence of a particular temperature will have a specific prob- Knowledge about temperature ex- 3.8.2 ability associated with it. Given this, tremes can inform the development What are it is entirely possible to have two 1 in of strategies for managing the risk 20 year events in successive years or associated with this type of natural temperature indeed in the same year. event. That temperature extremes do extremes? result in disastrous consequences, in A threshold value will be a specific terms of lives lost, is manifest via the Temperature extremes can occur over high or low temperature value, above observed impacts of a range of ex- a range of temporal (e.g. daily, month- or below which there is a discernible treme temperature events over the ly, seasonal, annual, decadal) and geo- impact. These can be described in last few decades (Table 3.3). Note- graphical scales (e.g. local to regional terms of percentiles, for example, the worthy is that all top 10 disasters are to global). They are usually defined in 5th or 95th percentile, meaning that related to extreme high as opposed to terms of their position in a distribu- for all the temperature observations low temperatures. tion of observed temperature values recorded for a location, the highest or 257 lowest set of temperatures are con- are some that refer to the duration of datasets that can be used for risk anal- sidered to fall within the lowest or high or low temperatures over sever- ysis have been constructed based on highest 5 % of values. Percentiles are al days. These are often referred to as available station data (Klok and Tank, a relative measure of extreme values, heat waves or cold waves. Although 2009; Menne et al., 2012). as the value associated with a particu- these terms are applied extensively in lar percentile will vary from location a range of fora, there is no standard to location. For example, the 95th definition of what a heat wave or cold percentile value of temperature for a wave is, despite a number of attempts There is a range of location in southern Europe may be to develop ‘universal’ heat wave and temperature extreme 35°C, while for a northern European cold wave definitions (Allen and Sher- metrics. Statistical location it may be 28°C. idan, 2016; Lhotka and Kysely, 2015; Perkins and Alexander, 2013; Robin- measures including Probabilities, return periods and per- son, 2001; Tong et al., 2010). probabilities, return centiles are just a few of a wide range periods and percentiles of possible measures of temperatures Building a picture of the nature of can be used to describe extremes. For example, Table 3.4 lists temperature extremes for a particu- their occurrence. a set of measures of temperature ex- lar location or region is dependent on Knowledge gaps exist tremes considered relevant to a range measurements from daily weather and of sectors of the economy and soci- climate observing stations. Accord- concerning extreme urban ety (Donat et al., 2013). Among these ingly, a number of daily temperature temperatures. TABLE 3.3 In addition to observational data, Top 10 extreme temperature disasters and associated death toll by coun- other sources are increasingly being try and date. used to develop extreme temperature Source: EM-DAT (2009) climatologies (e.g. assembled via data rescue and reconstruction projects, as well as the analysis of diaries and oth- Country Disaster type Date Total number of deaths er historical documents (McGregor, 2015)). Considerable effort has also Russian Federation Extreme high temperature 01/06/2010 55 736 gone into constructing gridded tem- perature datasets with a variety of spa- Italy Extreme high temperature 16/07/2003 20 089 tial and temporal resolutions (Donat et al., 2013). In the case of data-sparse France Extreme high temperature 01/08/2003 19 490 regions, stochastic weather generators have also been applied to the analysis Spain Extreme high temperature 01/08/2003 15 090 of temperature extremes (Rahmani et al., 2016; Steinschneider and Brown, Germany Extreme high temperature 01/08/2003 9 355 2013; Wilks, 2012). A range of reanal- ysis products such as the 20th century France Extreme high temperature 29/06/2015 3 275 (100-year) reanalysis dataset produced by the ECMWF (ERA-20C, n.d.) also Portugal Extreme high temperature 01/08/2003 2 696 offer considerable potential for ex- treme temperature analyses. Because India Extreme high temperature 26/05/1998 2 541 weather and climate stations were originally located to be representative India Extreme high temperature 20/05/2015 2 248 France 258 Extreme high temperature 15/07/2006 1 388 CHAPTER 3 UNDERSTANDING DISASTER RISK: HAZARD RELATED RISK ISSUES - SECTION III of atmospheric processes over large urban temperature fields and associat- climate networks and information regions, there are very few long-term ed extremes (Chen et al., 2012). systems (Chapman et al., 2015; Choi urban weather stations. This has con- et al., 2013; Honjo et al., 2015; Hu et strained the development of a full Accordingly, attention is now being al., 2016; Muller et al., 2013a, b). Fur- understanding of the complexities of turned to the development of urban thermore, satellite-based high spatial TABLE 3.4 List of the temperature indices recommended by the Expert Team on Climate Change Detection and Indices (ETCCDI) and calculated based on Global Historical Climatology Network (GHCN)-Daily station data. Percentile values used as the threshold for some of the indices are calculated for the base period 1961-90. Source: adopted from Donat et al. (2013) Identifier Indicator name Indicator definition Units TXx Hottest day Monthly maximum value of daily maximum temperature °C TNx Warmest night Monthly maximum value of daily minimum temperature °C TXn Coldest day Monthly minimum value of daily maximum temperature °C TNn Coldest night Monthly minimum value of daily minimum temperature °C TN10p Cool nights Percentage of time when daily minimum temperature < 10th percentile % TX10p Cool days Percentage of time when daily maximum temperature < 10th percentile % TN90p Warm nights Percentage of time when daily minimum temperature > 90th percentile % TX90p Warm days Percentage of time when daily maximum temperature > 90th percentile % DTR Diurnal Monthly mean difference between daily maximum and minimum °C temperature temperature range GSL Growing season Annual (1 January to 31 December in NH, 1 July to 30 June in SH) count Days length between first span of at least 6 days with TG > 5°C and first span after 1 July (1 January in SH) of 6 days with TG < 5°C. (NH stands for Northern Hemisphere, SH for Southern Hemisphere and TG is daily mean temperature) ID Ice days Annual count when daily maximum temperature < 0°C Days FD Frost days Annual count when daily minimum temperature < 0°C Days SU Summer days Annual count when daily maximum temperature > 25°C Days TR Tropical nights Annual count when daily minimum temperature > 20°C Days WSDI Warm spell Annual count when at least 6 consecutive days of maximum temperature Days duration index > 90th percentile CSDI Cold spell Annual count when at least 6 consecutive days of minimum temperature Days duration index < 10th percentile 259 resolution surface temperature obser- above a location or region. Many ex- extreme maximum temperatures can vations are also being applied in the treme temperature events can there- be significantly influenced by ENSO analysis of urban surface temperature fore be explained in terms of unusual for a range of regions across the fields (Azevedo et al., 2016; Hu et al., patterns of atmospheric circulation, world (Arblaster and Alexander, 2012; 2015; Jin, 2012) as well as the output such as ‘blocking’, which the term giv- Kenyon and Hegerl, 2008; Parker et from urban climate numerical models en to a situation in which a high-pres- al., 2014) as well as by Madden–Julian (Best and Grimmond, 2015; Loridan sure system becomes ‘stuck’ and does Oscillation-related anomalies in trop- and Grimmond, 2012). not move for several days. Blocking ical convection (Cassou et al., 2005; results in the flow of either very warm Matsueda and Takaya, 2015). Simi- 3.8.3 or cold air over a region or cloudless larly, the NAO has been found to in- skies that enhance heat gain or heat fluence the occurrence of both high- Climatic variability loss from the Earth’s surface. For ex- and low-temperature extremes across and change and ample, Della-Marta et al. (2007) have Europe (Burgess and Klingman, temperature shown that heat waves over Europe 2015; Hoy et al., 2013; Kenyon and are related to persistent and large- Hegerl, 2008; Moore and Renfrew, extremes scale high-pressure systems.
Details
-
File Typepdf
-
Upload Time-
-
Content LanguagesEnglish
-
Upload UserAnonymous/Not logged-in
-
File Pages32 Page
-
File Size-