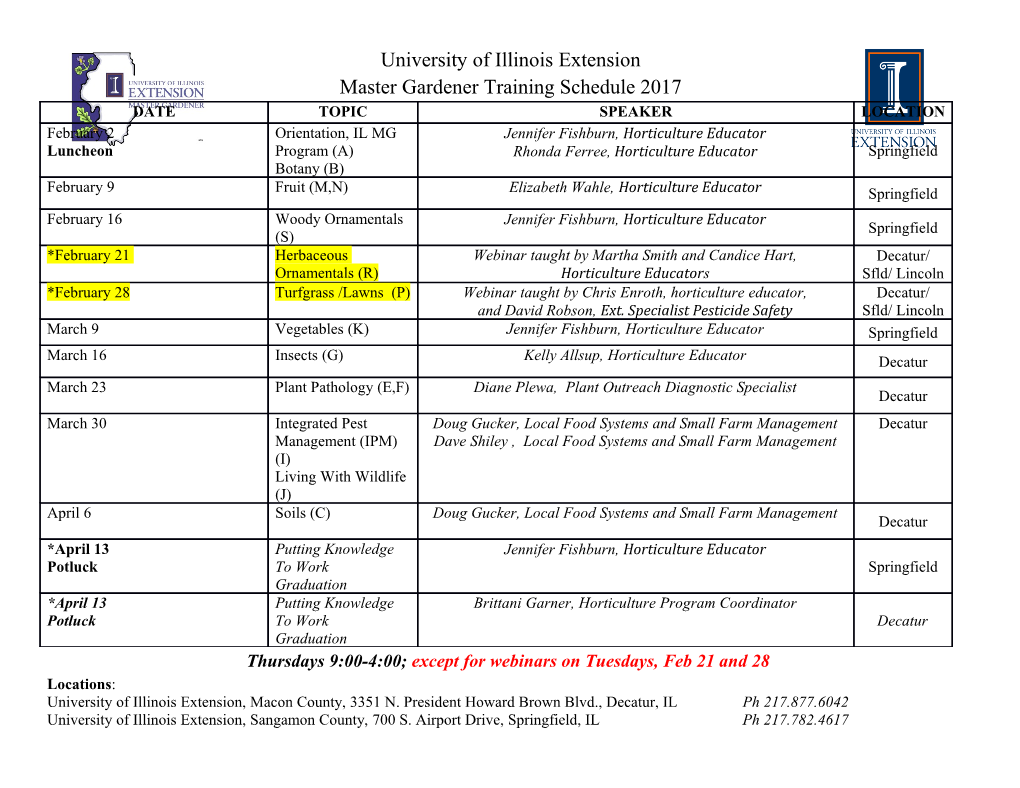
Financial Analysts Journal Volume 69 · Number 4 ©2013 CFA Institute “Sell in May and Go Away” Just Won’t Go Away Sandro C. Andrade, Vidhi Chhaochharia, and Michael E. Fuerst The authors performed an out-of-sample test of the sell-in-May effect documented in previous research. Reducing equity exposure starting in May and levering it up starting in November persists as a profitable market-timing strategy. On average, stock returns are about 10 percentage points higher for November– April half-year periods than for May–October half-year periods. The authors also found that the sell-in-May effect is pervasive in financial markets. multitude of calendar or seasonal anoma- The sell-in-May effect is distinct among sea- lies from the efficient market hypothesis sonal anomalies because it is the least affected by A (EMH) have been identified: for example, transaction costs. This fact is important because the January effect, the holiday effect, the turn-of- even if the anomaly is not a statistical fluke, it need the-month effect, and such day-of-the-week effects not be a challenge to the EMH. To be meaningful, as the Monday effect. However, as a predominantly anomalies from the EMH must be exploitable trad- nonexperimental field, financial economics is vul- ing opportunities net of transaction costs, a point nerable to spurious inferences from data mining. that Jensen (1978), Fama (1991), and Rubinstein In fact, many, if not most, calendar anomalies dissi- (2001) emphasized. pate after they have been identified. Regarding the The requirement of exploitability net of costs is a disappearance of the January effect, Eugene Fama weakness of several seasonal anomalies because the is quoted as saying, “I think it was all chance to full exploitation of such anomalies requires frequent begin with. There are strange things in any body of trading. For example, to fully exploit the turn-of- data” (Smalhout 2000, p. 29). the-month, Monday, and day-and-night effects, an Therefore, an objective test of a calendar effect investor would have to completely turn over a stock must include a test of the effect’s persistence using portfolio 12 times a year, 52 times a year, and 252 out-of-sample data. Sullivan, Timmermann, and times a year, respectively.2 In contrast, the sell-in- White (2001), who addressed the dangers of data min- May anomaly requires only two trades a year. ing for calendar effects, noted, “New data provides ■ Discussion of findings. We found that the sell- an effective remedy against data mining. Use of new in-May effect not only persists but also maintains data ensures that the sample on which a hypothesis the same economic magnitude as in Bouman and was originally based effectively is separated from the Jacobsen’s (2002) sample. On average across mar- sample used to test the hypothesis” (p. 269). kets and over time, stock returns are roughly 10 In this study, we performed the first comprehen- percentage points (pps) higher in November–April sive out-of-sample analysis of the anomaly identified half-year periods than in May–October half-year by the adage “Sell in May and go away,” also known periods. This result is quite remarkable in light as the Halloween effect. In the first academic analysis of the fate of most such calendar anomalies that of this effect, Bouman and Jacobsen (2002) studied 37 are subjected to scrutiny after their discovery (see markets and found higher returns in 35 of these mar- Dimson and Marsh 1999). kets in the November–April half-year period than in We also present novel evidence consistent with the May–October half-year period; November–April seasonal variation in aggregate risk aversion as the returns were significantly higher in 20 of the 37 mar- cause of the sell-in-May effect. We show that the kets. Bouman and Jacobsen’s sample ends in 1998. In sell-in-May effect is pervasive in financial markets; our study, we investigated the out-of-sample 1998– it is present across a wide variety of trading strat- 2012 period for those 37 equity markets.1 egies that plausibly reap returns as compensation for aggregate risk taking. Specifically, we found an Sandro C. Andrade is associate professor of finance, economically large and statistically significant sell- Vidhi Chhaochharia is associate professor of finance, and in-May effect for strategies that exploit the value, Michael E. Fuerst is lecturer of finance at the University size, credit risk, foreign exchange (FX) carry trade, of Miami, Coral Gables, Florida. and volatility risk premiums. 94 www.cfapubs.org ©2013 CFA Institute “Sell in May and Go Away” Just Won’t Go Away Data and Methodology Table 1 displays results in two panels: Panel A shows results for countries whose MSCI data begin We used MSCI stock market index total returns in 1970, and Panel B shows the results for countries in local currencies for the 37 countries in Bouman and Jacobsen’s (2002) sample. MSCI stock mar- whose MSCI data begin in 1988 or later (as described ket index data begin in 1970 for 18 countries and in the table notes). Each panel shows the results for in 1988 (or later) for 19 countries. We computed both the Bouman and Jacobsen (2002) sample period returns for adjacent half-year periods. May– ending in October 1998 and the subsequent out-of- October periods start at the beginning of May sample period beginning in November 1998. and end at the end of October, and November– Strikingly, Table 1 shows that the mean return April periods start at the beginning of November for November–April is larger than the mean return and end at the end of April. The Bouman and for May–October in all 37 countries for the out-of- Jacobsen sample period for each country begins sample period; that is, the sell-in-May effect persists in May of the year its MSCI index starts and ends out of sample. In addition, we confirmed Bouman in October 1998. Our out-of-sample period begins and Jacobsen’s results for their sample period and in November 1998 and ends in April 2012. Note found a sell-in-May effect in 35 of the 37 countries. that because we used half-year holding periods In total, 13 of the 37 countries had significantly from May to October and from November to greater returns for November–April out of sample, April, our sample periods must start in either compared with 19 of 37 countries for Bouman and May or November and end in either October or Jacobsen’s sample period. Note, however, that the April.3 average number of half-year periods in Bouman Our core statistical analysis is based on the fol- and Jacobsen’s sample period is 38 whereas it is lowing regression equation: only 27 in the out-of-sample period. Therefore, it is not surprising to find noisier point estimates in the rSit =+µαit+ εit , (1) out-of-sample period. where One caveat applies to the results in Table 1. For rit = the return on the stock index for country i the out-of-sample data, we calculated the differ- for period t ence between two sample means with a sample size 4 μi = the intercept for country i of only 14. The small sample size potentially led to α = the sell-in-May coefficient to estimate spurious results for individual countries. We miti- St = a dummy variable that takes a value of gated the small-sample problem by pooling data 1 for November–April periods and 0 for across all countries from Table 1 and performing a May–October periods test of normality of residuals to verify whether out- εit = the error term liers unduly influence results (see Table 2). Equation 1 is estimated both country by country and across countries using pooled data. Pooled and Value-Weighted Results Additionally, we estimated Equation 1 using Panel A of Table 2 contains the main results of MSCI World Index returns in local currencies. our study. It shows pooled estimates of the In contrast to using individual country data and sell-in-May coefficient α in Equation 1 for three equally weighting across countries, using MSCI samples. The first column provides the results World returns value weights the returns and for all 37 countries for Bouman and Jacobsen’s assigns larger weights to countries with larger sample period. In light of the results in Table 1, market capitalizations. we removed Brazil and Argentina from the sam- ple; these results are presented in the second col- Country-by-Country Results umn.5 In the third column, we present our novel Table 1 provides country-by-country mean returns out-of-sample results. for May–October and November–April periods For robustness, we present a number of differ- and an ordinary least-squares (OLS) estimate of ent pooled estimates for the sell-in-May coefficient the sell-in-May coefficient α in Equation 1. Note α in Equation 1. The equation is estimated using that OLS estimates of this coefficient are alge- either OLS or Prais–Winsten feasible generalized braically identical to differences in mean returns least squares (FGLS), both with and without fixed between the November–April and May–October effects (i.e., a different intercept for each market).6 periods. Owing to evidence of first-order autocor- Moreover, we report three types of robust standard relation of residuals in most countries, we report errors in the OLS specifications: (1) panel-corrected t-statistics computed using Newey–West standard standard errors (PCSEs), (2) Newey–West stan- errors with one lag.
Details
-
File Typepdf
-
Upload Time-
-
Content LanguagesEnglish
-
Upload UserAnonymous/Not logged-in
-
File Pages13 Page
-
File Size-