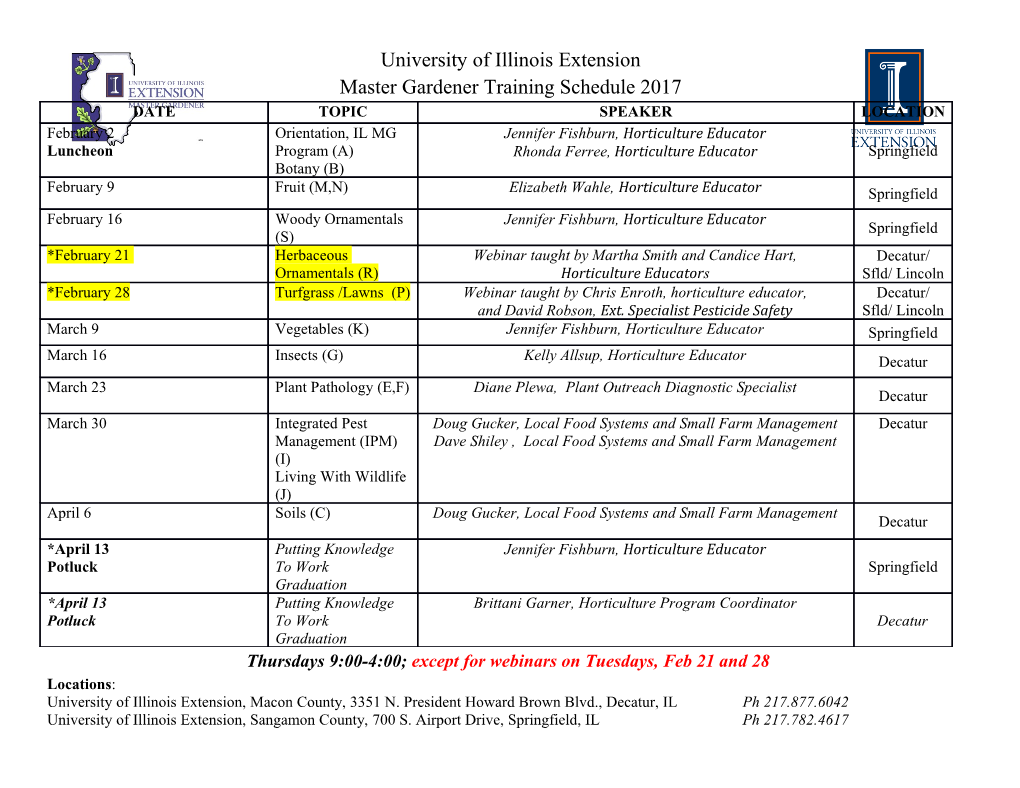
Beyond Ebola: Lessons for Mitigating Future Pandemics Carlos Castillo-Chavez1 Regents Professor, Director Simon A. Levin Mathematical, Computational and Modeling Sciences Center Tempe, AZ 85287-1904, USA Graduate School of Public Health University of Pittsburgh Monday, November 9, 2015 1 Draft [email protected];: https://twitter.com/mcmsc01 Overview Big Data Risk factors and recent outbreaks Modeling disease dynamics using a Lagrangian perspective Spatial spread of infectious diseases Steven Strogatz. The Real Scientific Hero of 1953. New York Times (1923-Current file), page 1, 2003. Three ways of doing science brought by James D. Watson and Francis Crick & the inventors of the computer experiment: Enrico Fermi, John Pasta and Stanislaw Ulam. The computer experiment offered a third way of doing science. Data Science (Big Data) is the fourth way of doingDraft science Beyond Ebola: Lessons learned ... Carlos Castillo-Chavez Simon A. Levin Modeling Center 2 / 65 Overview Big Data Risk factors and recent outbreaks Modeling disease dynamics using a Lagrangian perspective Spatial spread of infectious diseases Enrico Fermi, John Pasta and Stanislaw Ulam – in 1953 invented the concept of a "computer experiment. "... the most important lesson ...is how feeble even the best minds are at grasping the dynamics of large, nonlinear systems. Faced with a thicket of interlocking feedback loops, where everything affects everything else, our familiar ways of thinking fall apart. To solve the most important problems of our time, we’re going to have to change the way we do science." NYT 2003, S. Strogatz Draft Beyond Ebola: Lessons learned ... Carlos Castillo-Chavez Simon A. Levin Modeling Center 3 / 65 Overview Big Data Risk factors and recent outbreaks Modeling disease dynamics using a Lagrangian perspective Spatial spread of infectious diseases Training of Mathematical Scientists for the 21st Century “... As Fermi and his colleagues taught us, a complex system like cancer can’t be understood merely by cataloging its parts and the rules governing their interactions. The nonlinear logic of cancer will be fathomed only through the collaborative efforts of molecular biologists.” (Strogatz, 2003). The world’s ability to train 21st century mathematical scientists must rely on models of learning and thinking embedded within interdisciplinary educational research/mentorship models. Mathematical scientists must become proficient on multiple models of doing science including the systematic use of computer experiments and in data science. Draft Beyond Ebola: Lessons learned ... Carlos Castillo-Chavez Simon A. Levin Modeling Center 4 / 65 Overview Big Data Risk factors and recent outbreaks Modeling disease dynamics using a Lagrangian perspective Spatial spread of infectious diseases Source:%hlp://www.datasciencecentral.comDraft/forum/topics/theh3vshthathdefinehbighdata% Beyond Ebola: Lessons learned ... Carlos Castillo-Chavez Simon A. Levin Modeling Center 5 / 65 Overview Big Data Risk factors and recent outbreaks Modeling disease dynamics using a Lagrangian perspective Spatial spread of infectious diseases OakRidgeNaTonalLaboratory-June6,2013 Cray-madeTitan–thefastestcomputerintheworld Chinaannouncesfastercomputer Milky–Way2onJune17,2013 hl p://www.voanews.com/content/china-boasts-worlds- fastest-computer/1683465.html Draft Beyond Ebola: Lessons learned ... Carlos Castillo-Chavez Simon A. Levin Modeling Center 6 / 65 Overview Big Data Risk factors and recent outbreaks Modeling disease dynamics using a Lagrangian perspective Spatial spread of infectious diseases A Brief History Block of Mathematical Epidemiology The field was the result of the work of medical doctors - mathematical scientists Daniel Bernoulli (1700–1782) Sir Ronald Ross (1857–1932) Anderson G. McKendrick (1876–1943) William O. Kermack (1898–1970) www.fameimages.com/daniel-bernoulli www.nobelprize.org/nobel_prizes/medicine/laureates/1902/ross-bio.html www.york.ac.uk/depts/maths/histstat/people/ Draft Photograph courtesy of Godfrey Argent Studios Beyond Ebola: Lessons learned ... Carlos Castillo-Chavez Simon A. Levin Modeling Center 7 / 65 Overview Big Data Risk factors and recent outbreaks Modeling disease dynamics using a Lagrangian perspective Spatial spread of infectious diseases The mathematical theory of infectious diseases started by medical doctors Sir Ronald Ross (1857–1932) www.nobelprize.org/nobel_prizes/medicine/laureates/1902/ross-bio.html NobelDraft Laureate 1902 Beyond Ebola: Lessons learned ... Carlos Castillo-Chavez Simon A. Levin Modeling Center 8 / 65 Overview Big Data Risk factors and recent outbreaks Modeling disease dynamics using a Lagrangian perspective Spatial spread of infectious diseases Basic Malaria Model The Life–cycle of malaria parasites Ross-Macdonald Model x : Proportion of infected humans y : Proportion of infected mosquitoes dx = ab M y (1 x) rx dt N − − dy = ax (1 y) µy dt − − Parameter Definition Units M N Number of female mosquitoes per human host 1 a Biting rate on a human per mosquito day− b Infected mosquito to human transmission efficiency 1 r Per capita human recovery rate day− 1 µ Per capita mortalityDraft rate of mosquitos day− Beyond Ebola: Lessons learned ... Carlos Castillo-Chavez 9 / 65 Table: The parameters for the VL modelSimon and A. their Levin Modeling dimensions Center Overview Big Data Risk factors and recent outbreaks Modeling disease dynamics using a Lagrangian perspective Spatial spread of infectious diseases Basic Reproductive Number for Malaria 2 Biological interpretation 2 ma b 2 is the number of secondary cases = R0 0 µr of infection on hosts or vectors R generated by a single infective host or infective vector. a – number of bites per unit time b – infected bites that produce an infection M m = N – number of female mosquitoes per human host 1 r – duration of infection in human 1 – lifetime of a mosquito µ Draft Beyond Ebola: Lessons learned ... Carlos Castillo-Chavez Simon A. Levin Modeling Center 10 / 65 Overview Big Data Risk factors and recent outbreaks Modeling disease dynamics using a Lagrangian perspective Spatial spread of infectious diseases Holistic Perspective on Malaria How can we use what we learned from person to vector to person or vector to host to vector transmission at higher levels of organization? Holistic view - Public good: Can malaria be controlled at higher levels of organization? Problem across scales: how do we use knowledge at the individual level to understand phenomena at the population level? Validation of proposed control policies via the existence of a threshold: the prestige of mathematics and mathematical modeling Power of abstraction, can weDraft use this framework elsewhere: STDs Beyond Ebola: Lessons learned ... Carlos Castillo-Chavez Simon A. Levin Modeling Center 11 / 65 Overview Big Data Risk factors and recent outbreaks Modeling disease dynamics using a Lagrangian perspective Spatial spread of infectious diseases Gonorrhea: Transmission Dynamics and Control Herbert Hethcote Kenneth Cooke James A. Yorke University of Iowa Pomona College University of Maryland, College Park Herbert Hethcote and Jim Yorke changed health policy with their work on gonorrhea via their concept of Core Group Ken Cooke and Jim Yorke expanded significantly the work of Ross in 1970s with their work on GonorrheaDraft transmission and control Beyond Ebola: Lessons learned ... Carlos Castillo-Chavez Simon A. Levin Modeling Center 12 / 65 Overview Big Data Risk factors and recent outbreaks Modeling disease dynamics using a Lagrangian perspective Spatial spread of infectious diseases Ross’ "students" Kermack and McKendrick William Ogilvy Kermack Anderson Gray McKendrick (1898–1970) (1876–1943) Photograph courtesy of Godfrey Argent Studios Draftwww.york.ac.uk/depts/maths/histstat/people/ Beyond Ebola: Lessons learned ... Carlos Castillo-Chavez Simon A. Levin Modeling Center 13 / 65 Overview Big Data Risk factors and recent outbreaks Modeling disease dynamics using a Lagrangian perspective Spatial spread of infectious diseases The basic reproduction number for the SIR model without vital dynamics T(4) T(3) T(2) Infected T(1) Index case Susceptible T(0) Infected Index case R0=2 Susceptible No Transmission Transmission The basic reproduction number, , defined as the number of secondary cases R0 generated by a typical infectious individual during its period of infectiousness in an entirely susceptible population. Draft Beyond Ebola: Lessons learned ... Carlos Castillo-Chavez Simon A. Levin Modeling Center 14 / 65 Overview Big Data Risk factors and recent outbreaks Modeling disease dynamics using a Lagrangian perspective Spatial spread of infectious diseases System of equations without vital dynamics - single outbreak S β IS I γ R dS SI States Meaning = β , dt − N S # of Susceptible dI SI = β γI, I # of Infectecd dt N − R # of Recovered dR = γI, dt Parameters Meaning β Transmission coefficient N = S + I + R. Draftγ Per-capita recovery rate Beyond Ebola: Lessons learned ... Carlos Castillo-Chavez Simon A. Levin Modeling Center 15 / 65 Overview Big Data Risk factors and recent outbreaks Modeling disease dynamics using a Lagrangian perspective Spatial spread of infectious diseases On the basic reproduction number R0 = β R0 γ 1 depends on number of contacts and probability of transmission (both R0 quantities captured by β) and the infectious period (1/γ). 2 If < 1 then the infection dies out. R0 3 If > 1 then an epidemic ensues R0 4 Accurate estimation of the value of the reproductive number are central in the planning of control
Details
-
File Typepdf
-
Upload Time-
-
Content LanguagesEnglish
-
Upload UserAnonymous/Not logged-in
-
File Pages65 Page
-
File Size-