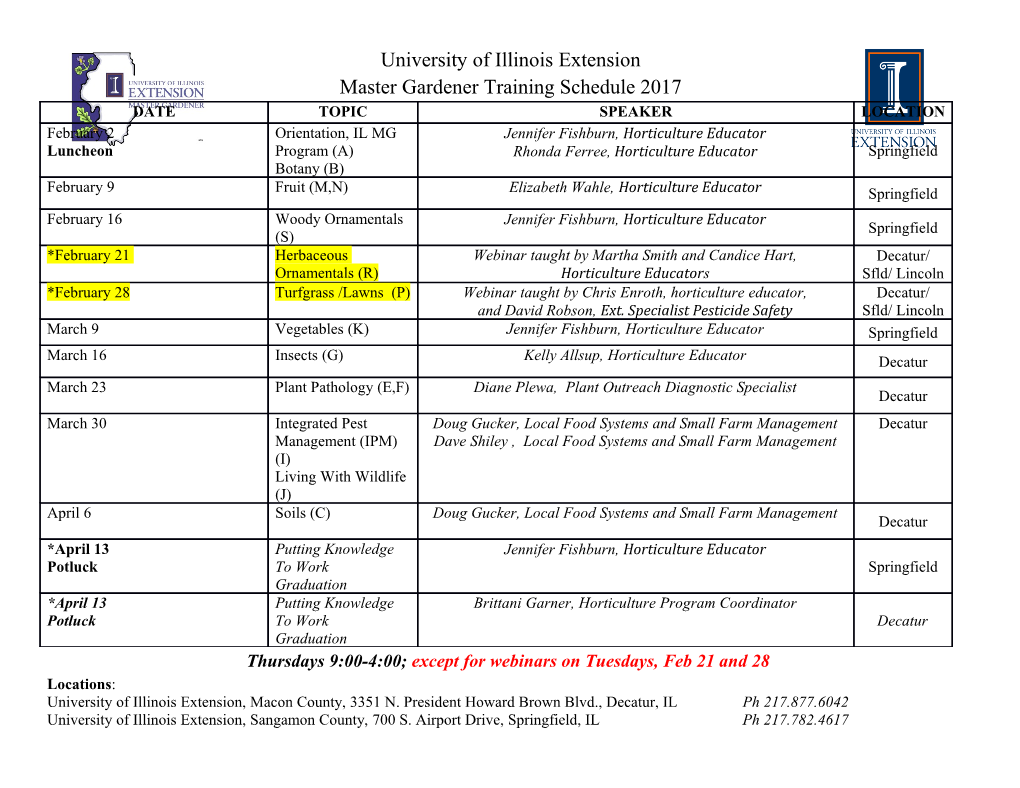
www.nature.com/scientificreports OPEN Metabolomics‑based discrimination of patients with remitted depression from healthy controls using 1H‑NMR spectroscopy Ching‑I. Hung1,2, Gigin Lin2,3,4, Meng‑Han Chiang2,3,4 & Chih‑Yung Chiu2,4,5* The aim of the study was to investigate diferences in metabolic profles between patients with major depressive disorder (MDD) with full remission (FR) and healthy controls (HCs). A total of 119 age‑matched MDD patients with FR (n = 47) and HCs (n = 72) were enrolled and randomly split into training and testing sets. A 1H‑nuclear magnetic resonance (NMR) spectroscopy‑based metabolomics approach was used to identify diferences in expressions of plasma metabolite biomarkers. Eight metabolites, including histidine, succinic acid, proline, acetic acid, creatine, glutamine, glycine, and pyruvic acid, were signifcantly diferentially‑expressed in the MDD patients with FR in comparison with the HCs. Metabolic pathway analysis revealed that pyruvate metabolism via the tricarboxylic acid cycle linked to amino acid metabolism was signifcantly associated with the MDD patients with FR. An algorithm based on these metabolites employing a linear support vector machine diferentiated the MDD patients with FR from the HCs with a predictive accuracy, sensitivity, and specifcity of nearly 0.85. A metabolomics‑based approach could efectively diferentiate MDD patients with FR from HCs. Metabolomic signatures might exist long‑term in MDD patients, with metabolic impacts on physical health even in patients with FR. Major depressive disorder (MDD) is a common mental disorder. However, no robust objective laboratory test is available for the diagnosis of MDD or evaluation of the severity of depression. A metabolomics-based approach can be employed to identify products of a given biochemical system and metabolic substrates, and therefore this approach has emerged as a method by which to increase our understanding of diseases and biological systems in a large-scale manner1. Te technology of metabolomics ofers signifcant potential as a tool to investigate the diagnosis of diseases and responses to medications. Metabolomics has been used in MDD-related research, such as to evaluate the severity of depression 2, identify biomarkers of MDD 3–9, for predictive diagnosis of MDD 10–13, identify metabolic profles post-antidepressant treatment14–17, pinpoint biomarkers of metabolites for drug response phenotypes18,19, and diferentiate MDD from bipolar disorder12,20,21. Full remission (FR) of depression is a treatment goal for patients with MDD. One of the commonly-used def- nitions of FR is a 17-item Hamilton Depression Rating Scale (HAMD) score ≤ ­722. However, MDD with FR does not equate to achieving health23,24. For example, cognitive dysfunction, which may hinder functional recovery, is one of the common residual symptoms of depression, and may persist during the remission phase 25. Tis raises two interesting questions: (1) are there any diferences in the metabolic profles between MDD patients with FR and healthy controls (HCs), and (2) is it possible to establish an algorithm based on metabolites as biomarkers to diferentiate MDD patients with FR from HCs? Te majority of studies of metabolomics in MDD patients, as described above, have been concerned with identifying biomarkers or obtaining a predictive diagnosis of MDD, or predicting the response to antidepres- sants. Few studies have focused on investigating diferences in metabolite expressions between MDD patients with FR and HCs using targeted metabolomics analysis 26,27, and to our knowledge, no study has comprehensively investigated diferences in metabolite levels in peripheral plasma between MDD patients with FR and HCs. An 1Department of Psychiatry, Chang-Gung Memorial Hospital at Linkou, Taoyuan, Taiwan, ROC. 2College of Medicine, Chang Gung University, Taoyuan, Taiwan, ROC. 3Department of Medical Imaging and Intervention, Imaging Core Laboratory, Institute for Radiological Research, Chang Gung Memorial Hospital at Linkou, Taoyuan, Taiwan, ROC. 4Clinical Metabolomics Core Laboratory, Chang Gung Memorial Hospital at Linkou, Taoyuan, Taiwan, ROC. 5Division of Pediatric Pulmonology, Department of Pediatrics, Chang Gung Memorial Hospital at Linkou, 5 Fu-Shing St., Kweishan, Taoyuan 333, Taiwan, ROC. *email: [email protected] Scientifc Reports | (2021) 11:15608 | https://doi.org/10.1038/s41598-021-95221-1 1 Vol.:(0123456789) www.nature.com/scientificreports/ algorithm based on metabolomics analysis to diferentiate MDD patients with FR from HCs is still lacking. However, investigation of the above two issues is important, because MDD has negative impacts on multiple physical systems28–30. Abnormalities in metabolites among MDD patients with FR might be associated with long-term negative impacts on physical health. Furthermore, recurrence is common in MDD, and investigation of these issues may provide clues as to the recurrence of depression and subsequent prevention of depression. Terefore, this study aimed to comprehensively investigate the diferences in metabolomic profles in periph- eral plasma between patients with MDD with FR and HCs, and to then establish an algorithm based on metabo- lomics analysis to diferentiate MDD patients with FR from HCs. We hypothesized that an algorithm based on metabolomics analysis could be efective in diferentiating MDD patients with FR from HCs. Methods Subjects. Te subjects included in this study were nested within a project that examined MDD patients and were recruited at the 10-year follow-up point from August 2014 to December 201629,31,32. At baseline (from Janu- ary 2004 to August 2007), patients diagnosed with MDD in that project were enrolled from outpatient clinics of the Psychiatric Department of Chang Gung Memorial Hospital at Linkou, a medical center in northern Taiwan. Te outpatients fulflled the criteria for MDD, and were diagnosed using the Structured Clinical Interview for DSM-IV-text revision (TR) Axis I Disorders33. At baseline, 229 participants with MDD were enrolled, then were treated by antidepressants. At the 10-year follow-up point, 137 (47.2%) subjects attended follow-up. Te severity of depression was evaluated using the 17-item Hamilton Depression Rating Scale (HAMD)34 administered by a psychiatrist, and among the 137 sub- jects, a total of 47 MDD patients were in FR, which was defned as a HAMD score ≤ ­722, and had been medication- free for at least 6 months and had no history of substance abuse or dependence. Sixty-seven healthy persons were simultaneously enrolled as controls. Te exclusion criteria for the HCs were as follows: (1) any current or previous lifetime history of neurological or DSM-IV-TR axis I/II diagnoses; (2) systemic medical diseases, such as hypertension, diabetes mellitus, and others; and (3) any family history of psychiatric disorders. Te project was approved by the Institutional Review Board of Chang Gung Memorial Hospital (No. 105-5895C). Based on the guidelines regulated in the Declaration of Helsinki, written informed consent was acquired from all subjects. Te enrolled subjects, including 47 MDD patients with FR and 67 HCs, were then randomly split (3/5 for training, 2/5 for testing) into training (30 MDD patients with FR and 42 HCs) and testing sets (17 MDD patients with FR and 30 HCs) for algorithm development. Twelve-hour fasting plasma samples of the 47 MDD patients with FR and 67 HCs were collected and analyzed at the 10-year follow-up point. Fasting plasma parameters including glucose, cholesterol, or triglyceride, alanine aminotransferase (ALT), and aspartate aminotransferase (AST) analyzed by completely automated methods at clinical laboratories were also analyzed. Plasma sample preparation. Fasting blood samples for plasma collection were obtained at 9–10 a.m., aliquoted and stored immediately at − 80 °C until analysis. Tawed plasma samples were centrifuged at 12,000×g at 4 °C for 30 min. 500 μL of plasma supernatant were mixed with 500 μL of 0.075 M phosphate bufer (pH 7.40) in 20% deuterium water containing 0.08% 3-(trimethylsilyl)-propionic-2,2,3,3-d4 acid sodium salt (TSP) as an internal chemical shif reference standard. Te mixed samples were vortexed for 20 s and centrifuged at 12,000×g at 4 °C for 30 min, following which 600 μL of the supernatant were loaded into a standard 5-mm NMR tube (Bruker BioSpin, Billerica, MA, USA) for further analysis. Nuclear magnetic resonance (NMR) spectrum acquisition. NMR experiments were performed at Chang Gung Healthy Aging Research Center, Taiwan. 1H-NMR spectra were acquired on a Bruker Avance 600- MHz spectrometer (Bruker-Biospin GmbH, Karlsruhe, Germany) equipped with a 5-mm CPTCI 1H cryoprobe. Temperature was controlled at 300 K throughout the experiments. Relaxation-edited spectra were acquired using Carr–Purcell–Meiboom–Gill (CPMG)-presat pulse sequence. In CPMG method, a series of 180° pulse was applied with the radio-frequency (RF) pulses in 27.24 μs and the water presaturation bandwidth 25 Hz. Low-power water pre-saturation pulse sequence was used for water signal suppression during the relaxation time of 4 s. For each spectrum, 64 transients were collected into 64 K data points using a spectral window of 20 ppm during a relaxation time of 4 s. Te temperature-controlled Bruker SampleJet automation unit was installed for sample handling and laboratory automation. Prior to Fourier transformation, all 1H-NMR spectra were processed with zero-flling and exponential line-broadening of 0.3 Hz. Te acquired spectra were manu- ally phased, baseline corrected, and the internal TSP signal calibrated to δ 0.0 ppm using TopSpin 3.2 sofware (Bruker BioSpin, Rheinstetten, Germany). NMR data processing and analysis. NMRProcFlow (https:// www. nmrpr ocfow. org), an open-source sofware, provides comprehensive tools for the processing and visualization of 1D NMR data. Te raw 1H-NMR spectra were imported into NMRProcFlow 1.3 for ppm calibration, baseline correction, alignment, spectra bucketing and data normalization 35. Spectra bucketing was performed using the method of intelligent bucket- ing and variable size bucketing with the full range of 10.0–0.00 ppm36.
Details
-
File Typepdf
-
Upload Time-
-
Content LanguagesEnglish
-
Upload UserAnonymous/Not logged-in
-
File Pages8 Page
-
File Size-