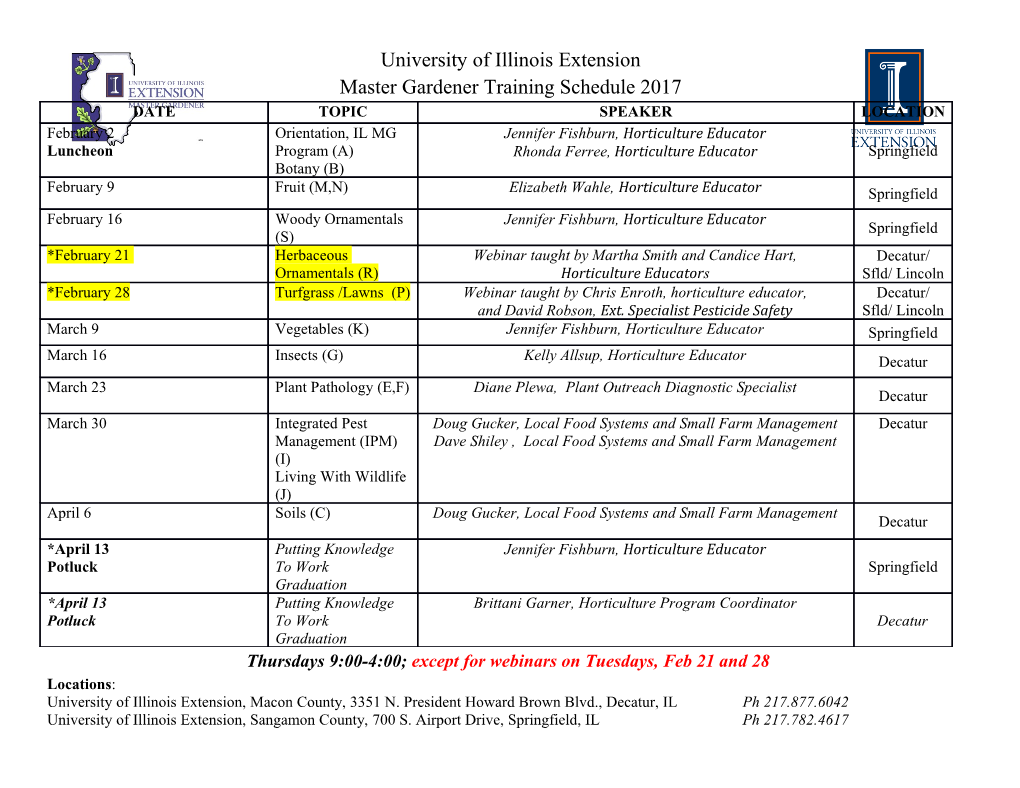
Time Series Feature Extraction for Industrial Big Data (IIoT) Applications [1] Term paper for the subject „Current Topics of Data Engineering” In the course of studies MSc. Data Engineering at the Jacobs University Bremen Markus Sun Born on 07.11.1994 in Achim Student/ Registration number: 30003082 1. Reviewer: Prof. Dr. Adalbert F.X. Wilhelm 2. Reviewer: Prof. Dr. Stefan Kettemann Time Series Feature Extraction for Industrial Big Data (IIoT) Applications __________________________________________________________________________ Table of Content Abstract ...................................................................................................................... 4 1 Introduction ...................................................................................................... 4 2 Main Topic - Terminology ................................................................................. 5 2.1 Sensor Data .............................................................................................. 5 2.2 Feature Selection and Feature Extraction...................................................... 5 2.2.1 Variance Threshold.............................................................................................................. 7 2.2.2 Correlation Threshold .......................................................................................................... 7 2.2.3 Principal Component Analysis (PCA) .................................................................................. 7 2.2.4 Linear Discriminant Analysis (LDA) ..................................................................................... 8 2.2.5 Autoencoders ....................................................................................................................... 8 2.3 Time Series ............................................................................................. 9 2.3.1 Autocorrelation ..................................................................................................................... 9 2.3.2 Seasonality ........................................................................................................................ 10 2.3.3 Stationarity ......................................................................................................................... 11 2.4 Feature Extraction from Time Series Data ............................................ 12 3 Method Description - “TSFRESH” Package for Feature Extraction in Python 12 4 Conclusion & Outlook ..................................................................................... 14 References ............................................................................................................... 15 2 Markus Sun List of Figures Figure 1 - Data Points in different dimensions [9] .................................................................. 6 Figure 2 - Autoencoder's NN Architecture [11] ....................................................................... 9 Figure 3 - Autocorrelation example [12] ................................................................................10 Figure 4 - Example of Seasonality [12] .................................................................................11 Figure 5 - Example of a non-stationary process [12] .............................................................11 Figure 6 - Overview about Data Scientist's working time [13] ...............................................13 Abstract The chosen topic in this term paper for the subject “Current Topics of Data Engineering” is “Time Series Feature Extraction for Industrial Big Data (IIoT) applications.” This report should rather be seen as a project proposal for possible future projects, which shows some insights regarding the topic itself and possible approaches. This term paper has mainly two parts. One of them is the introduction of the terminologies and some of its methods, which are related to the particular topic of this paper. The other is the description of a potential method for solving this problem in a real case, which is the usage of the package “TSFRESH” from python. 1 Introduction Nowadays, the terms “Artificial Intelligence,” “Artificial Neural Networks,” “Machine Learning” and “Deep Learning” are often used in a context with innovations, which has a huge impact potential for today’s world. Even though the mathematical/statistical concepts and ideas behind them already existed since several decades ago, they just became more popular not too long ago. There are mainly two reasons for the popularity gain. One is the advanced processing power of computers, and the other one is big data. The latter describes the vast amount of data, which are produced by the digital equipment and sensors in this digital age. In the past 50 years, the amount of data increased exponentially, furthermore 90% of all data up to date has been created in the last two years. [2] The estimated amount of data we should have by 2020 is 40 trillion gigabytes of data [3]. It’s needless to say that this vast quantity of big data has a significant potential to access new knowledge, but it has no value unless we can analyze it and find the hidden patterns within. That’s precisely where machine learning techniques are needed. Within the area of data analytics, machine learning techniques are known as predictive analytics. The strength of these techniques is to find valuable hidden patterns within a vast amount of complex data. With these newly gained pieces of information, it is possible to predict some future events and helps to find a solution for a specific complex problem. One specific research field, which is referred to as Human Activity Recognition (HAR), yields great interest for medical, military, and security applications. [4] Related to this research field, the “Universität Bremen” in Bremen, Germany, organized a competition “The Bremen Big Data Challenge 2019”. The objective of this particular challenge was the classification of various leg movements. The data was collected through different sensors beforehand, which were positioned on the leg of human subjects. The student group, which is formed by Gari Ciodaro, Diogo Cosin, and Ralph Florent used a variety of feedforward neural networks to take on the challenge. The highest obtained accuracy for the training set was 84.44% and 91%. The accuracy of the challenge data set (test set) was 54% and 64%. [5] Generally spoken, industrial big data, the Internet of Things (IoT), robotics, and other similar information sources are generating a large volume of variety information in huge velocity, variability (inconsistency), and veracity (imprecision). In order to create models with good performances, one needs to carefully merge and integrate these kinds of data by analyzing and extracting the most useful features from different time intervals. This report aims to discuss the time series feature extraction for industrial big data (IIoT) applications and its possible usage for the “The Bremen Big Data Challenge 2019”, in order to optimize the prediction accuracy of the machine learning models. [6] 2 Main Topic - Terminology This chapter briefly introduces some of the terminologies, which are correlated to the topic of time series feature extraction. It is important to know about the terminology in order to have a good overall understanding of the topic. At first, I will introduce the term “sensor data”, which will be followed by “feature extraction”, “feature selection” and “time series”. 2.1 Sensor Data Sensors are indispensable devices in nowadays world. A sensor is a device, which measures certain physical properties by detecting different types of input from its environment. The measured properties can be, for instance, pressure, force, displacement, acceleration, temperature, vibration or even electrochemical potential. Once the property is measured, it will be converted into a standardized control signal. This standardized control signal will then be converted directly at the device (sensor) into a readable display, or it will be electronically transmitted via a network for further processing. [7] The output of these devices is referred to as sensor data. It can be used to provide information or as an input to another system. Sensor data is a crucial component for the Internet of Things (IoT) environment because most of the data, which are transmitted over a network are sensor data. [8] 2.2 Feature Selection and Feature Extraction The world has more data than ever, and the amount is increasing at an exponential rate. This increasing amount of data leads to data sets with confusingly many features. Therefore, it is important to know the difference between interesting data and useful data or in other words, the ability to discriminate between the relevant and irrelevant parts of a given data set. This procedure of selecting some subset of a machine learning algorithm’s input variables, on which the algorithm should put its focus on and ignore the rest, is referred to as “Dimensionality Reduction”. [9] This reduction of dimensionality is needed because of a phenomenon that occurs by classifying, organizing, and analysing data with high dimensions, the curse of dimensionality. “Dimensionality” in the context of machine learning refers to the number of features (variables) in a data set. As it can be seen in figure 1 below, where the data space moves from a one- dimensional perspective to two dimensions and then to three dimensions, the given data fills less of the whole data space, each time
Details
-
File Typepdf
-
Upload Time-
-
Content LanguagesEnglish
-
Upload UserAnonymous/Not logged-in
-
File Pages16 Page
-
File Size-