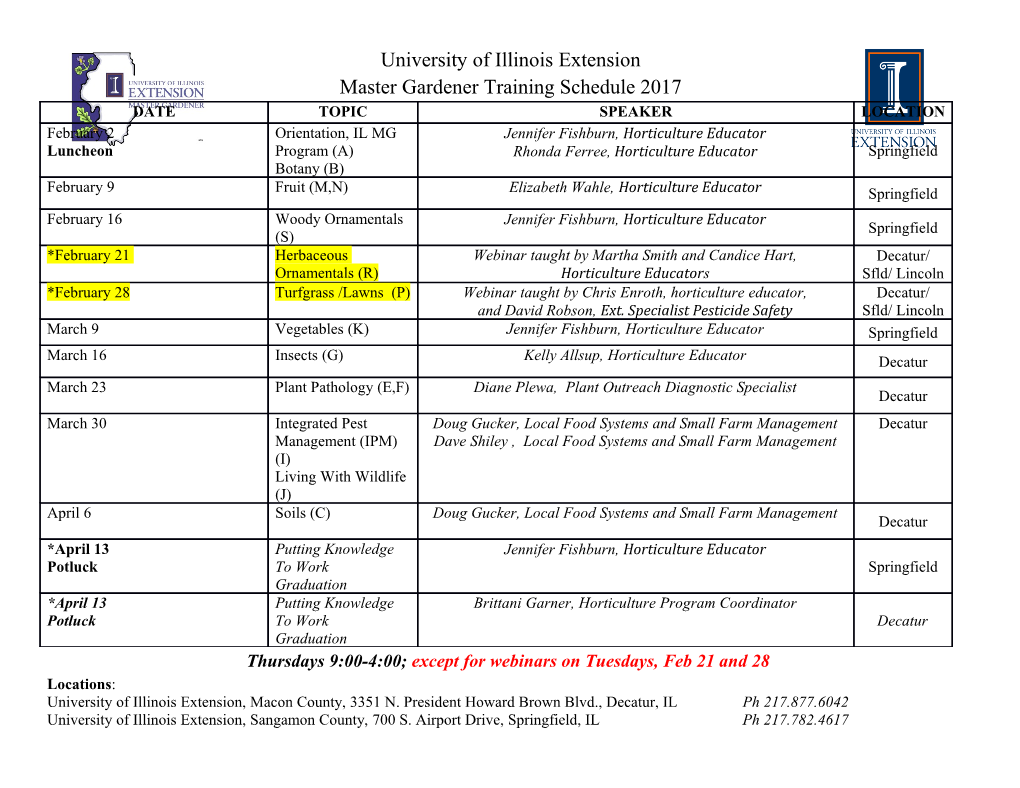
View metadata, citation and similar papers at core.ac.uk brought to you by CORE provided by Scholarship@Western Western University Scholarship@Western Electronic Thesis and Dissertation Repository 4-22-2013 12:00 AM Comparison of option pricing between ARMA-GARCH and GARCH-M models Yi Xi The University of Western Ontario Supervisor Dr. Reginald J. Kulperger The University of Western Ontario Graduate Program in Statistics and Actuarial Sciences A thesis submitted in partial fulfillment of the equirr ements for the degree in Master of Science © Yi Xi 2013 Follow this and additional works at: https://ir.lib.uwo.ca/etd Part of the Other Statistics and Probability Commons Recommended Citation Xi, Yi, "Comparison of option pricing between ARMA-GARCH and GARCH-M models" (2013). Electronic Thesis and Dissertation Repository. 1215. https://ir.lib.uwo.ca/etd/1215 This Dissertation/Thesis is brought to you for free and open access by Scholarship@Western. It has been accepted for inclusion in Electronic Thesis and Dissertation Repository by an authorized administrator of Scholarship@Western. For more information, please contact [email protected]. COMPARISON OF OPTION PRICING BETWEEN ARMA-GARCH AND GARCH-M MODELS (Thesis format: Monograph) by Yi Xi Graduate Program in Statistics and Actuarial Science A thesis submitted in partial fulfillment of the requirements for the degree of Master of Science The School of Graduate and Postdoctoral Studies The University of Western Ontario London, Ontario, Canada ⃝c Yi Xi 2013 Abstract Option pricing is a major area in financial modeling. Option pricing is sometimes based on normal GARCH models. Normal GARCH models fail to capture the skewness and the leptokurtosis in financial data. The variant GARCH-in-mean (GARCH-M) model is widely used in the option pricing literature. It adds a heteroskedasticity term to the mean equation, which is interpreted as a risk premium, and also incorporates a type of asymmetry. Our goal is to compare option valuation between GARCH-M and ARMA-GARCH models with normal and non-normal, z-distributed innovations. The models are fitted to the historical return data, and risk neutral measures are based on the conditional Esscher transform and the extended Girsanov principle. We compare European Calls on the S&P 500 with the model predictions. The TGARCH is best for ARMA-GARCH/GARCH-M models. Neither normal nor z dominates the other, but overall z-TGARCH-M (z-innovations) seems to be best, ARMA- TGARCH is surprisingly good. Keywords: option pricing, ARMA-GARCH, GARCH-in-Mean, z-distribution, Esscher transform, Extended Girsanov principle ii Acknowlegements First and foremost, I would like to express my deepest gratitude to my program supervisor Dr. Reginald J. Kulperger for his generous support, encouragement, patience and valuable guidance during my time at University of Western Ontario. During these two years spent at Western he was not only my academic supervisor but also my mentor. I deeply indebted to you for all that you have done for me during all these years. I was one of recipients of Richard Konrad Ontario Graduate Scholarship. I really appreci- ated the generous support from Mr. Konrad and the Provincial Government. I also wish to express my heart-felt thank you to all the faculty, staff, and fellow graduate students in the Department of Statistical and Actuarial Science who have made my stay here both enjoyable and memorable. My sincere thanks to Dr. Mark Resser, Dr. Matt Davison and Dr. Rogemar Mamon for agreeing to act as examiners. I am appreciated the selfless love, devotion, and encouragement from my parents. In addi- tion, I am grateful to my husband Lijun. Her love, support and words of encouragement have been a constant that I have been able to count on throughout this experience. iii Contents Abstract ii Acknowlegements iii List of Figures vii List of Tables viii List of Appendices x 1 Introduction 1 2 Financial Series and Models 4 2.1 Financial Time Series . 4 2.2 ARMA Model . 5 2.3 GARCH Model . 6 2.3.1 Properties of the GARCH(1,1) Models . 7 2.3.2 Extensions of GARCH Model . 8 3 Estimation of the GARCH Models 11 3.1 Estimation Methods . 11 3.1.1 Maximum Likelihood Estimation . 11 3.1.2 Quasi-Maximum Likelihood . 12 3.2 Estimating ARMA-GARCH and GARCH-M Models . 14 3.3 Model Fitting Analysis . 16 4 Risk Neutral Measures Under GARCH Models 22 4.1 Definitions and Notations . 22 4.2 Risk Neutral Measures . 24 4.2.1 Conditional Esscher Transform . 24 4.2.2 Extended Girsanov Principle . 26 4.3 Applications for GARCH Models . 27 4.3.1 GARCH with Normal Innovation . 27 4.3.2 GARCH with Z-distributed Innovation . 28 5 Numerical Experiments 32 5.1 Simulation Steps . 32 iv 5.2 Option Data Description . 34 5.3 Empirical Analysis . 37 5.3.1 Option Data Set 1 . 37 5.3.2 Option Data Set 2 . 46 6 Conclusion and Future research 52 6.1 Conclusion . 52 6.2 Future Research . 53 Bibliography 55 A Tables of Pricing Errors for Example 2 59 Curriculum Vitae 68 v List of Figures 3.1 Daily closing prices and returns of S&P 500 Index from January 02, 1988 to January 06, 2004 . 17 3.2 Log-density of observed residuals versus their theoretical density for Normal distribution . 20 3.3 Log-density of observed residuals versus their theoretical density for z-distribution 21 5.1 European Call option price evaluated on April 18, 2002 for different models with the maturities T = 22, 46, 109, 173, and 234 days; the closing stock price on that day was S 0 = $1124:47. 40 5.1 Continued Table: European Call option price evaluated on April 18, 2002 for different models with the maturities T = 22, 46, 109, 173, and 234 days; the closing stock price on that day was S 0 = $1124:47. 41 5.2 Model pricing errors for European Call options on April 18, 2002. 42 5.2 Continued Table: Model pricing errors for European Call options on April 18, 2002. 43 5.3 Implied volatility smiles based on MLE estimates using returns from January 07, 2004 to December 29, 2004 . 49 vi List of Tables 2 3.1ϵ ˜t(#) andσ ˜t ( ) in GARCH models . 15 3.2 GARCH and TGARCH parameters estimated by MLE with normal innovations using daily closing prices of S&P 500 from January 02,1988 to January 06, 2004 18 3.3 GARCH and TGARCH parameters estimated by MLE, which under the as- sumption on z-distributed noise, using daily closing prices of S&P 500 from January 02, 1988 to January 06, 2004 . 19 5.1 S&P 500 call option prices from Schoutens (2003) . 35 5.2 Number of Call option contracts (S&P 500 Index, January 07, 2004 to Decem- ber 29, 2004 ) . 36 5.3 Average price of Call option contracts (S&P 500 Index, January 07, 2004 to December 29, 2004 ) . 36 5.4 Overall pricing errors for European Call options on April 18, 2002 . 38 5.5 GARCH and TGARCH parameters estimated by MLE with normal distribution using daily closing prices of S&P 500 from January 02, 1988 to April 17, 2002 44 5.6 GARCH and TGARCH parameters estimated by MLE, which under the as- sumption on z-distributed noise, using daily closing prices of S&P 500 from January 02, 1988 to April 17, 2002 . 45 5.7 Overall pricing errors for European Call options in data set 2 . 46 5.8 Overall pricing errors regarding to moneyness(Call option contracts of S&P 500 Index, January 07, 2004 to December 29, 2004) . 50 5.9 Overall pricing errors regarding to maturity (Call option contracts of S&P 500 Index, January 07, 2004 to December 29, 2004) . 51 A.1 Pricing errors for GARCH-M (Call option contracts of S&P 500 Index, January 07, 2004 to December 29, 2004) . 60 A.2 Pricing errors for TGARCH-M (Call option contracts of S&P 500 Index, Jan- uary 07, 2004 to December 29, 2004) . 61 A.3 Pricing errors for ARMA-GARCH (Call option contracts of S&P 500 Index, January 07, 2004 to December 29, 2004) . 62 A.4 Pricing errors for ARMA-TGARCH (Call option contracts of S&P 500 Index, January 07, 2004 to December 29, 2004) . 63 A.5 Pricing errors for z-GARCH-M (Call option contracts of S&P 500 Index, Jan- uary 07, 2004 to December 29, 2004) . 64 A.6 Pricing errors for z-TGARCH-M (Call option contracts of S&P 500 Index, January 07, 2004 to December 29, 2004) . 65 vii A.7 Pricing errors for z-ARMA-GARCH (Call option contracts of S&P 500 Index, January 07, 2004 to December 29, 2004) . 66 A.8 Pricing errors for z-ARMA-TGARCH (Call option contracts of S&P 500 In- dex, January 07, 2004 to December 29, 2004) . 67 viii List of Appendices Appendix A Tables of Pricing Errors for Example 2 . 59 ix Chapter 1 Introduction The theory of option pricing is an important topic in the financial literature. The seminal works of Black and Scholes and Merton were the starting point for European option pricing. Follow- ing the finding that these model prices systematically differ from market prices, the literature on option valuation has formulated a number of theoretical models designed to capture these empirical biases. Many empirical studies on asset price dynamics have demonstrated that char- acteristics such as time-varying volatility, volatility clustering, non-normality, and leverage effect etc. should be taken into account when modelling financial data.
Details
-
File Typepdf
-
Upload Time-
-
Content LanguagesEnglish
-
Upload UserAnonymous/Not logged-in
-
File Pages78 Page
-
File Size-