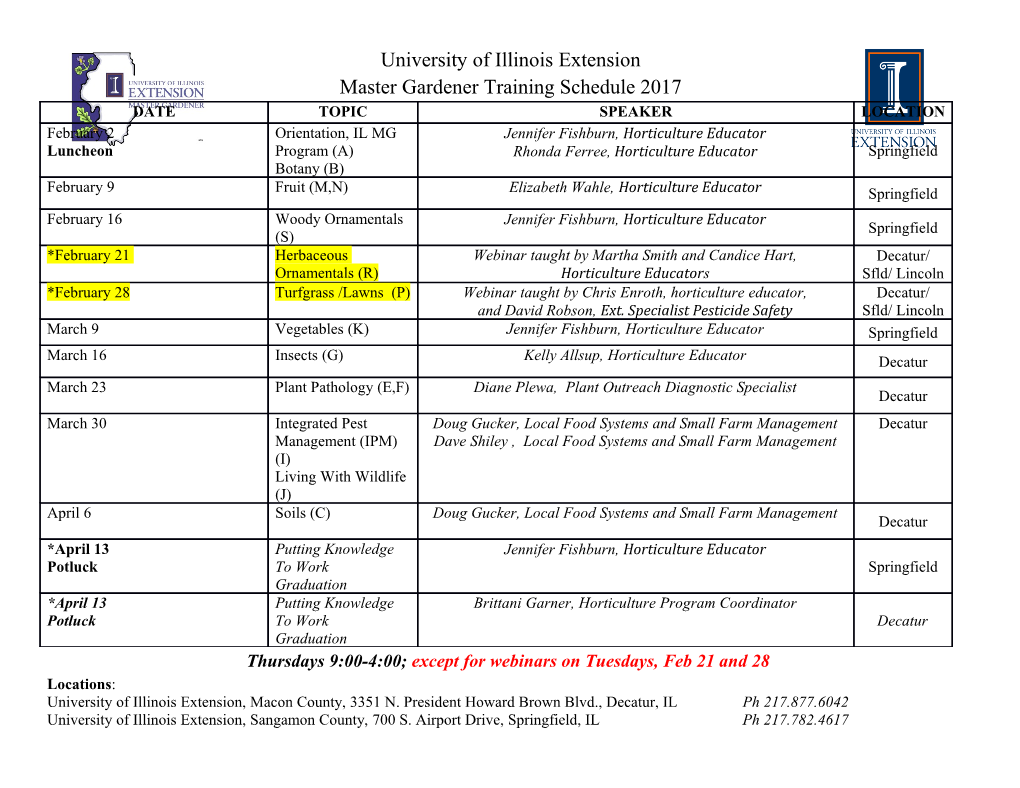
Lagrange Multipliers in the Calculus of Variations Francis J. Narcowich, January 2020 The problem1that we wish to address is the following: Consider the func- R b 0 R b 0 tionals J(y) = a f(x; y; y )dx and K(y) = a g(x; y; y )dx. Extremize (max- imize/minimize) J over all y such that y 2 C1[a; b], y(a) = A, y(b) = B, and K(y) = L, where L is a constant. Theorem 0.1. If y = y extremizes J(y), subject to the conditions above, then there exists a constant λ such that y is an unconstrained extremal for R b D(y) = a (f − λg)dx, y(a) = A; y(b) = B. @f 0 R x @f 0 Proof. Let F (x) = @y0 (x; y;¯ y¯ ) − a @y (t; y¯(t); y¯ (t))dt − c1, where c1 is de- R b @g R x @g termined by a F (x)dx = 0. Similarly, let G(x) = @y0 − a @y dt − c2, where R b a G(x)dx = 0. (Both F and G are evaluated with y = y, so they are fixed functions of x.) Next, consider the function Z x Z x ye := y + " F (t)dt + δ G(t)dt: a a This function satisfies the boundary conditions at x = a and x = b, because ye(a) = y(a) + · 0 + δ · 0 = y(a) = A; and Z b Z b ye(b) = y(b) + " F (x)dx +δ G(x)dx = B: a a | {z } | {z } 0 0 We can require it to satisfy the constraint, too. The quantity K(ye) = R b g(x; y; y0)dx depends only on the parameters and δ. The reason is that a e e y~ depends on known functions, y, F (x) and G(x). These are integrated out when K(ye) is computed. The only variables left are " and δ. The same is true when J(ye) is computed. Thus, we may let φ("; δ) := J(ye) and ("; δ) := K(ye). The constraint is satisfied by requiring ("; δ) = L, which implicitly defines a curve relating δ and ". 1The proof presented here may be found in Akhiezer's book on the Calculus of Varia- tions. See the list of references for a full citation. 1 The extremum for J occurs at " = δ = 0, because φ(0; 0) = J(y), subject to the constraint ("; δ) = L. This is equivalent to a 2D Lagrange multiplier problem: Extremize φ("; δ) − λ( ("; δ) − L); with no constraint. Making use of the extremum occurring at (0; 0) gives us @φ @ @φ @ (0; 0) = λ (0; 0) and (0; 0) = λ (0; 0): (0.1) @" @" @δ @δ We need to compute the various derivatives in the equation above. For @φ @" (0; 0), we have @φ @ Z b @f Z x @f (0; 0) = J(y)j = F (t)dt + F (x) dx: e (0;0) 0 @" @" a @y a @y Integrating the first term on the right by parts then yields @φ Z b @f Z x @f (0; 0) = 0 − dt F (x)dx @" a @y a @y | {z } F (x)+c1 Z b Z b 2 = F (x) dx + c1 F (x)dx = hF; F i a a | {z } 0 Similar calculations result in @ @ @φ (0; 0) = hF; Gi; (0; 0) = hG; Gi; and (0; 0) = hF; Gi: @" @δ @δ Using these in (0.1) and putting the resulting equations in matrix form, we see that hF; F i hF; Gi 1 0 = hF; Gi hG; Gi −λ 0 By multiplying on the left by (1 − λ) and collecting terms, we arrive at kF − λGk2 = 0, which implies that F − λG = 0; consequently, @(f − λg) Z x @(f − λg) 0 − dt = c; @y a @y which is the du Bois-Reymond form of the Euler-Lagrange equations for D(y). These hold for y = y and do not involve the constraint, as required. 2.
Details
-
File Typepdf
-
Upload Time-
-
Content LanguagesEnglish
-
Upload UserAnonymous/Not logged-in
-
File Pages2 Page
-
File Size-