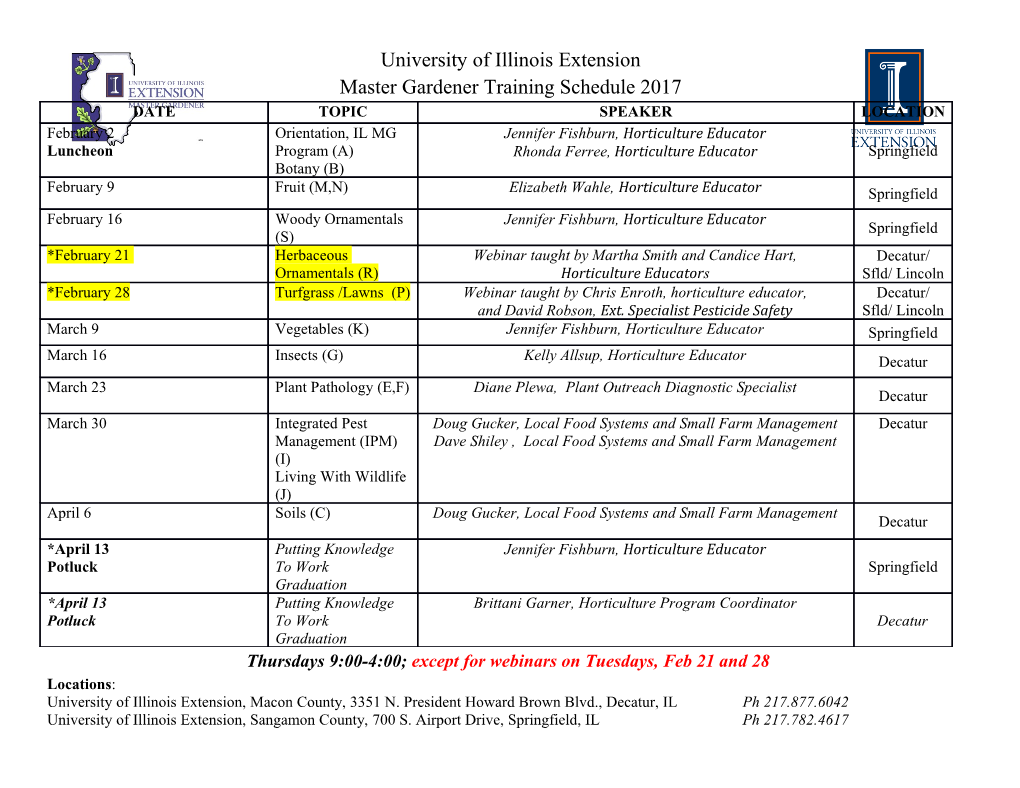
MIT Case Studies in Social and Ethical Responsibilities of Computing The Bias in the Machine: Facial Recognition Technology and Racial Disparities Sidney Perkowitz1 1Charles Howard Candler Emeritus Professor of Physics, Emory University Published on: Feb 05, 2021 License: Creative Commons Attribution-NonCommercial 4.0 International License (CC-BY- NC 4.0) MIT Case Studies in Social and Ethical Responsibilities of Computing The Bias in the Machine: Facial Recognition Technology and Racial Disparities ABSTRACT Facial recognition technology (FRT) appears in uses from providing secure access to smartphones, to identifying criminal suspects from surveillance images as a tool of the justice system. Citizen’s rights and social justice groups, alongside the research community, have identified undesirable societal consequences arising from the uncritical use of FRT algorithms, including false arrest and excessive government surveillance. Within the United States, these consequences have disproportionately affected people of color, both because algorithms have typically been less accurate when applied to nonwhite people, and because—like any new forensic technology—FRT systems are being incorporated into systems and institutions with their own histories of disparities. As research continues to address racial biases in the performance of FRTs, examples from older forensic technologies, such as fingerprint identification, might offer insights into improving real-world deployment. Sidney Perkowitz Charles Howard Candler Emeritus Professor of Physics, Emory University Keywords: facial recognition, justice system, racial equity, false arrest Author Disclosure(s): Portions of this case study originally appeared in Sidney Perkowitz, “The Bias in the Machine: Why Facial Recognition Has Led to False Arrests,” Nautilus, August 19, 2020, and are reproduced with permission. In January 2020, Robert Williams, a Black man, was wrongfully arrested due to an inaccurate facial recognition algorithm, a computerized approach that analyzes human faces and identifies them by comparison to database images of known people. Williams was handcuffed and arrested in front of his family by Detroit police without being told why, then jailed overnight after the police took mugshots, fingerprints, and a DNA sample. The next day, detectives showed Williams a surveillance video image of a Black man standing in Shinola, a store that sells expensive watches. It immediately became clear that Williams was not the person shown in the video. Detailing his arrest in the Washington Post, Williams wrote, “The cops looked at each other. I heard one say that ‘the computer must have gotten it wrong.’”1 Williams learned 2 MIT Case Studies in Social and Ethical Responsibilities of Computing The Bias in the Machine: Facial Recognition Technology and Racial Disparities that in investigating a theft from the store, a facial recognition system had tagged his driver’s license photo as matching an image from the surveillance video. The error from the algorithm was then compounded when police relied upon the assertion of a private investigator that the algorithm had correctly matched the photos. Rather than following appropriate procedures—in which police must first confirm a machine match, then seek additional evidence sufficient for an arrest—they simply arrested Williams. Only after detectives saw Williams in person and compared him to the surveillance image did they see the differences, and the case against him collapsed. But those steps came after Williams had spent thirty hours in jail and posted a $1,000 bond.2 The Williams arrest was briefly unique because it received public attention. As the American Civil Liberties Union (ACLU) observed, over four thousand police departments across the United States use facial recognition systems, making it highly likely that other people have been wrongly implicated in crimes.3 Indeed, soon several similar cases came to light. In February 2019, another Black man, Nijeer Parks, was accused of shoplifting and attacking a police officer in Woodbridge, New Jersey. Facial recognition software had identified him as a suspect from a driver’s license photo. After he spent ten days in jail and paid $5,000 in legal fees to defend himself, unassailable evidence showed that he had been miles away at the time the crime occurred.4 Meanwhile, in July 2019, a facial recognition match sent Detroit police to arrest a third Black man, Michael Oliver. The charge, a felony count of larceny, was dropped when a judge agreed that he had been misidentified, as shown by mismatched tattoos.5 These cases highlight the significant problem with facial recognition technology in criminal investigations: if the algorithm wrongly selects someone as a suspect, that can harm an innocent person. Moreover, new technologies, such as facial recognition algorithms, are being incorporated within systems of policing and surveillance (within the United States and elsewhere) that already have histories of racial disparity and inequality. These preexisting patterns heighten the stakes surrounding the adoption of any new technology, especially for people of color, as emphasized by scholars such as Ruha Benjamin in her ground-breaking study Race After Technology and Brian Jefferson in Digitize and Punish.6 Consider a concrete example: after Nijeer Parks was arrested, he seriously considered admitting guilt and accepting a plea bargain—even though he had been arrested based on a faulty image match and he had been thirty miles away when the crime was committed—because he had prior criminal convictions (from about a decade earlier), which could have led to a severe sentence if he had been convicted at trial.7 As recently as 2018 and 2019, detailed studies by researchers at MIT and Microsoft Research and at the US National Institute of Standards and Technology (NIST) identified persistent inaccuracies in algorithms that were designed to detect and/or identify faces when applied to people of color.8 The algorithms were also less accurate for women than for men, with the largest errors, up to 35 percent, arising for female faces of color, according to the MIT/Microsoft study.9 This report and its authors, Joy 3 MIT Case Studies in Social and Ethical Responsibilities of Computing The Bias in the Machine: Facial Recognition Technology and Racial Disparities Buolamwini and Timnit Gebru, have been instrumental in raising the issue of bias in facial analysis software. Buolamwini, who founded the Algorithmic Justice League to support her efforts, explains the origins of her work in the documentary film Coded Bias (2020).10 These recent efforts highlight at least two major challenges: constructing algorithms that have comparably high accuracy for identifying individuals across racial, ethnic, gender, and age groups; and incorporating such technologies into real-world systems—such as policing and government surveillance—that already have long histories of systematic inequalities. Early Challenges One reason for the racial disparities in the performance of facial recognition technologies is the relative lack of nonwhite faces in the sample data sets that have been used to develop the algorithms. The poor representation of people of color from around the world, and their range of facial features and skin shades, creates what researchers call a “demographic bias” built into the technology. On the other hand, the criminal databases used by police in the United States oversample people of color, leading to further racial disparities. In Digitize and Punish, Jefferson notes, “In some cities, 80 percent of the black male population is listed in [criminal justice] databases.”11 Likewise, in Race After Technology, Benjamin describes a gang database in California in which people of color constitute 87 percent of the entries; some entrants in the database were infants, less than a year old.12 Disparities in the data with which facial recognition technologies have been developed stretch back to the origins of the field. Consider, for example, the efforts of American mathematician and artificial intelligence (AI) pioneer Woodrow Wilson “Woody” Bledsoe. Beginning in 1959, he produced several innovations in pattern recognition and computer vision that made him a major figure in the development of AI.13 Although his pioneering efforts in facial recognition have since been surpassed, they underscore the long-standing challenges of addressing bias in data collection, sampling, and analysis. Working at the Sandia Corporation in Albuquerque, New Mexico, Bledsoe and a colleague first invented a way for a machine to recognize alphanumeric characters. They projected a character, say the letter “A,” onto a rectangular 10 x 15 array of photocells. Each photocell represented a discrete picture element or pixel and received a binary 1 or 0 depending on whether or not it contained part of the image. The pixels were sampled in adjacent groups of two or more called “n-tuples” to account for the spatial relations among them. Further manipulation produced a set of binary digits embodying the letter “A.” This process found and stored the bits and a resulting unique score for every character; then an unknown character was identified by comparing its score to the values in memory. The method worked well, correctly recognizing up to 95 percent of handwritten or printed numerals. 4 MIT Case Studies in Social and Ethical Responsibilities of Computing The Bias in the Machine: Facial Recognition Technology and Racial Disparities But when
Details
-
File Typepdf
-
Upload Time-
-
Content LanguagesEnglish
-
Upload UserAnonymous/Not logged-in
-
File Pages16 Page
-
File Size-