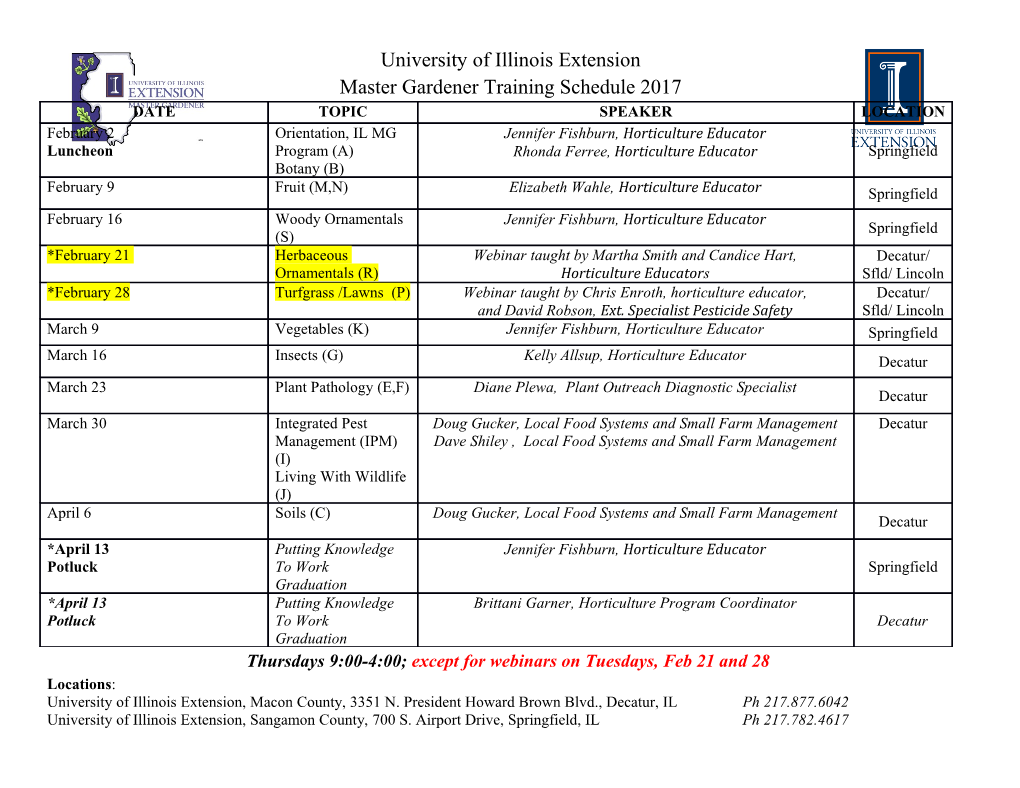
J. Virtamo 38.3143 Queueing Theory / Discrete Distributions 1 DISCRETE DISTRIBUTIONS Generating function (z-transform) Definition Let X be a discrete r.v., which takes non-negative integer values, X 0, 1, 2,... ∈{ } Denote the point probabilities by pi p = P X = i i { } The generating function of X denoted by (z) (or (z); also X(z) or Xˆ(z)) is defined by G GX ∞ i X (z)= piz = E[z ] G iX=0 Rationale: A handy way to record all the values p , p ,... ; z is a ‘bookkeeping variable’ • { 0 1 } Often (z) can be explicitly calculated (a simple analytical expression) • G When (z) is given, one can conversely deduce the values p , p ,... • G { 0 1 } Some operations on distributions correspond to much simpler operations on the generating • functions Often simplifies the solution of recursive equations • J. Virtamo 38.3143 Queueing Theory / Discrete Distributions 2 Inverse transformation The problem is to infer the probabilities p , when (z) is given. i G Three methods 1. Develop (z) in a power series, from which the p can be identified as the coefficients of G i the zi. The coefficients can also be calculated by derivation i 1 d (z) 1 (i) pi = G = (0) i z=0 i! dz i!G 2. By inspection: decompose (z) in parts the inverse transforms of which are known; G e.g. the partial fractions 3. By a (path) integral on the complex plane 1 (z) path encircling the origin (must be chosen so p = G dz i 2πi I zi+1 that the poles of (z) are outside the path) G J. Virtamo 38.3143 Queueing Theory / Discrete Distributions 3 Example 1 1 (z)= =1+ z2 + z4 + G 1 z2 ··· − 1 for i even pi = ⇒ 0 for i odd Example 2 2 2 2 2 1 (z)= = = G (1 z)(2 z) 1 z − 2 z 1 z − 1 z/2 − − − − − − A Since corresponds to sequence A ai we deduce 1 az · − p =2 (1)i 1 (1)i =2 (1)i i · − · 2 − 2 J. Virtamo 38.3143 Queueing Theory / Discrete Distributions 4 Calculating the moments of the distribution with the aid of G(z) Since the pi represent a probability distribution their sum equals 1 and (0) ∞ i (1) = (1) = pi 1 =1 G G iX=1 · By derivation one sees (1)(z) = d E[zX ] G dz X 1 = E[Xz − ] (1)(1) = E[X] G By continuing in the same way one gets (i)(1) = E[X(X 1) (X i +1)] = F G − ··· − i th where Fi is the i factorial moment. J. Virtamo 38.3143 Queueing Theory / Discrete Distributions 5 The relation between factorial moments and ordinary moments (with respect to the origin) The factorial moments Fi = E[X(X 1) (X i + 1)] and ordinary moments (with resect i − ··· − to the origin) Mi = E[X ] are related by the linear equations: F1 = M1 M1 = F1 F2 = M2 M1 M2 = F2 + F1 − F3 = M3 3M2 +2M1 M3 = F3 +3F2 + F1 . − . . . . . For instance, F = (2)(1) = E[X(X 1)] = E[X2] E[X] 2 G − − M = E[X2]= F + F = (2)(1) + (1)(1) ⇒ 2 2 1 G G V[X]= M M 2 = (2)(1) + (1)(1) ( (1)(1))2 = (2)(1) + (1)(1)(1 (1)(1)) ⇒ 2 − 1 G G − G G G −G J. Virtamo 38.3143 Queueing Theory / Discrete Distributions 6 Direct calculation of the moments The moments can also be derived from the generating function directly, without recourse to the factorial moments, as follows: d X 1 (z) = E[Xz − ]z=1 = E[X] dz z=1 G d d 2 X 1 2 z (z) = E[X z − ]z=1 = E[X ] dz dz z=1 G Generally, i d d i 1 d i E[X ]= (z ) − (z) = (z ) (z) dz dz z=1 dz z=1 G G J. Virtamo 38.3143 Queueing Theory / Discrete Distributions 7 Generating function of the sum of independent random variables Let X and Y be independent random variables. Then (z) = E[zX+Y ] = E[zXzY ] GX+Y = E[zX]E[zY ] independence = (z) (z) GX GY (z)= (z) (z) GX+Y GX GY In terms of the original discrete distributions p = P X = i i { } qj = P Y = j { } the distribution of the sum is obtained by convolution p q ⊗ k P X + Y = k = (p q)k = piqk i { } ⊗ iX=0 − Thus, the generating function of a distribution obtained by convolving two distributions is the product of the generating functions of the respective original distributions. J. Virtamo 38.3143 Queueing Theory / Discrete Distributions 8 Compound distribution and its generating function Let Y be the sum of independent, identically distributed (i.i.d.) random variables Xi, Y = X + X + X 1 2 ··· N where N is a non-negative integer-valued random variable. Denote (z) the common generating function of the X X i G N (z) the generating functionof N G We wish to calculate (z) GY Y Y (z) = E[z ] G Y = E[E z N ] X1|+ XN = E[E z ··· N ] | = E[E zX1 zXN N ] ··· | = E[ (z)N ] GX = ( (z)) GN GX (z)= ( (z)) GY GN GX J. Virtamo 38.3143 Queueing Theory / Discrete Distributions 9 Bernoulli distribution X Bernoulli(p) ∼ A simple experiment with two possible outcomes: ‘success’ and ‘failure’. We define the random variable X as follows 1 when the experiment is successful; probability p X = 0 when the experiment fails; probability q =1 p − Example 1. X describes the bit stream from a traffic source, which is either on or off. The generating function (z) = p z0 + p z1 = q + pz G 0 1 E[X] = (1)(1) = p G V[X] = (2)(1) + (1)(1)(1 (1)(1)) = p(1 p) = pq G G −G − Example 2. The cell stream arriving at an input port of an ATM switch: in a time slot (cell slot) there is a cell with probability p or the slot is empty with probability q. J. Virtamo 38.3143 Queueing Theory / Discrete Distributions 10 Binomial distribution X Bin(n, p) ∼ X is the number of successes in a sequence of n independent Bernoulli trials. n X = Yi where Yi Bernoulli(p) and the Yi are independent (i =1,...,n) iX=1 ∼ The generating function is obtained directly from the generating function q+pz of a Bernoulli variable n n n i n i i (z) = (q + pz) = p (1 p) − z G iX=1 i − By identifying the coefficient of zi we have n i n i pi = P X = i = p (1 p) − { } i − E[X] = nE[Y ]= np i V[X] = nV[Yi]= np(1 p) − A limiting form when λ = E[X]= np is fixed and n : →∞ n n (z 1)λ (z)=(1 (1 z)p) = (1 (1 z)λ/n) e − G − − − − → which is the generating function of a Poisson random variable. J. Virtamo 38.3143 Queueing Theory / Discrete Distributions 11 The sum of binomially distributed random variables Let the Xi (i = 1,...,k) be binomially distributed with the same parameter p (but with different ni). Then the distribution of their sum is distributed as X + + X Bin(n + + n , p) 1 ··· k ∼ 1 ··· k because the sum represents the number of successes in a sequence of n + + n identical 1 ··· k Bernoulli trials. J. Virtamo 38.3143 Queueing Theory / Discrete Distributions 12 Multinomial distribution Consider a sequence of n identical trials but now each trial has k (k 2) different outcomes. ≥ k Let the probabilities of the outcomes in a single experiment be p1, p2,...,pk ( i=1 pi = 1). P Denote the number of occurrences of outcome i in the sequence by Ni. The problem is to calculate the probability p(n ,...,n ) = P N = n ,...,N = n of the joint event N = 1 k { 1 1 k k} { 1 n ,...,N = n . 1 k k} Define the generating function of the joint distribution of several random variables N1,...,Nk by N1 Nk ∞ ∞ n1 nk (z1,...,zk)=E[z1 zk ]= ... p(n1,...,nk)z1 zk G ··· nX1=0 nkX=0 ··· After one trial one of the Ni is 1 and the others are 0. Thus the generating function corre- sponding one trial is (p z + + p z ). 1 1 ··· k k The generating function of n independent trials is the product of the generating functions of a single trial, i.e. (p z + + p z )n. 1 1 ··· k k From the coefficients of different powers of the zi variables one identifies n! 1 k when n + ... + n = n, p(n ,...,n )= pn pn 1 k 1 k n ! n ! 1 ··· k 0 otherwise 1 ··· k J. Virtamo 38.3143 Queueing Theory / Discrete Distributions 13 Geometric distribution X Geom(p) ∼ X represents the number of trials in a sequence of independent Bernoulli trials (with the probability of success p) needed until the first success occurs i =1, 2,... i 1 Note that sometimes the distribution of X 1 is p = P X = i = (1 p) − p − i { } − defined to be the geometric distribution (starts from 0) Generating function ∞ i 1 i pz (z)= p (1 p) − z = G iX=1 − 1 (1 p)z − − This can be used to calculate the expectation and the variance: p(1 (1 p)z)+ p(1 p)z 1 E[X] = 0(1) = − − − = 2 z=1 G (1 (1 p)z) p − − 2 1 2(1 p) E[X ] = 0(1) + 00(1) = + − G G p p2 1 p V[X] = E[X2] E[X]2 = − − p2 J.
Details
-
File Typepdf
-
Upload Time-
-
Content LanguagesEnglish
-
Upload UserAnonymous/Not logged-in
-
File Pages25 Page
-
File Size-