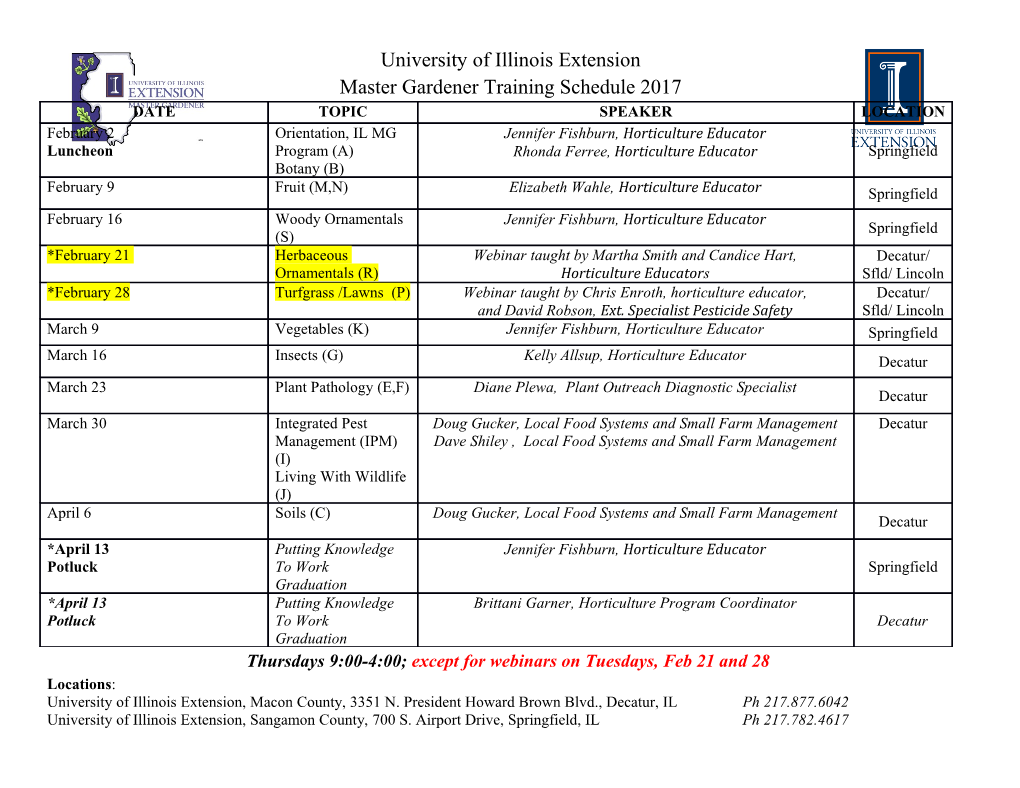
Downloaded from orbit.dtu.dk on: Sep 28, 2021 Handwritten Digit Classication using 8-bit Floating Point based Convolutional Neural Networks Gallus, Michal; Nannarelli, Alberto Publication date: 2018 Document Version Publisher's PDF, also known as Version of record Link back to DTU Orbit Citation (APA): Gallus, M., & Nannarelli, A. (2018). Handwritten Digit Classication using 8-bit Floating Point based Convolutional Neural Networks. DTU Compute. DTU Compute Technical Report-2018 Vol. 01 General rights Copyright and moral rights for the publications made accessible in the public portal are retained by the authors and/or other copyright owners and it is a condition of accessing publications that users recognise and abide by the legal requirements associated with these rights. Users may download and print one copy of any publication from the public portal for the purpose of private study or research. You may not further distribute the material or use it for any profit-making activity or commercial gain You may freely distribute the URL identifying the publication in the public portal If you believe that this document breaches copyright please contact us providing details, and we will remove access to the work immediately and investigate your claim. Handwritten Digit Classification using 8-bit Floating Point based Convolutional Neural Networks Michal Gallus and Alberto Nannarelli (supervisor) Danmarks Tekniske Universitet Lyngby, Denmark [email protected] Abstract—Training of deep neural networks is often con- In order to address this problem, this paper proposes usage strained by the available memory and computational power. of 8-bit floating point instead of single precision floating point This often causes it to run for weeks even when the underlying which allows to save 75% space for all trainable parameters, platform is employed with multiple GPUs. In order to speed up and possibly reduce power and computation time. To test the the training and reduce space complexity the paper presents an proposed solution the computations are ran on LeNeT-5 [10] approach of using reduced precision (8-bit) floating points for network using MNIST hand-written images dataset [11]. training hand-written characters classifier LeNeT-5 which allows for achieving 97.10% (Top-1 and Top-5) accuracy while reducing The paper is organised in the following manner: Section the overall space complexity by 75% in comparison to a model II presents the current advancements in the field. Section III using single precision floating points. concerns the main topic of the paper where the structure of 8- Keywords—approximate computing, deep learning and 12-bit float will be presented along with the architecture known as LeNeT-5. In section IV reader can find the results of training and validation of the aforementioned network using I. INTRODUCTION floats of different bit-widths. Finally the section V concludes Image classification has recently been dominated by Con- the paper and proposes further improvements. volutional Neural Networks (CNNs) that provide state of the art accuracy, sometimes reaching human level capabilities. In II. RELATED WORK order to achieve better results by means of accuracy, the deep neural network models rapidly grow in size, resulting in long During the design of special purpose hardware intended training times, high demand for memory and computational for deep neural networks processing, one of the key factors to power. One of the most commonly seen solutions to this prob- consider is the used precision and format of numbers used for lem concerns employing large CPU Clusters [1], or Graphical storing the results and performing the computations. Most of Processing Units (GPUs) [2] which recent models of started the literature so far has focused on using limited precision dur- including deep learning specific hardware units, such as Tensor ing the forward pass (also known as inference), while training Cores in Nvidia Volta [3]. Application Specific Integrated the network on single precision. Some of the recent works have Circuits (ASICs) as well as FPGA solutions have also been presented usage of custom hardware implementations [12], proposed [4]. while others have employed the usage of FPGAs [4] for the sake of reconfigurability or using SSE3 vector instructions to What the majority of aforementioned solutions fail to perform multiple (up to 16) 8-bit operations in parallel [13]. exploit is the inherent resilience to errors associated with deep Other studies concern usage of different number format. learning applications. Because the neural networks are exposed In [14] 24-bit floating point processing units are used, while to work with real-life noisy data while maintaining successful [15] uses 16-bit fixed point with stochastic rounding, achieving results [5], they do not require full precision range provided errors of 0.70% on modified LeNeT-5 architecture. This result by the hardware in a form of 64-, 32- and even 16-bit floating has later been reproduced along with introduction of dynamic point numbers. Limiting the precision of underlying primitives fixed point by [16]. Hammerstrom [17] shows successful train- which build up the network can result in power, time and ing of the network using 16- and 8-bit fixed-point arithmetic. memory savings. This work presents that both training and inference is possi- Nearly all of the state of the art convolutional neural net- ble using not only fixed- but also low precision (down to 8-bits) work models spend most of the processing time on convolution floating point arithmetic. This includes direct application to not and max pooling layer [6], [7], [8]. Timespan of performing only simple perceptron networks, but also models of higher all convolution operations on the whole network amounts to complexity, such as LeNeT-5 architecture which can be used 80% of total time spent on training [9]. At the same time, as fully functional module for Optical Character Recognition nearly 20% of remaining time is spent on subsampling, also (OCR) program. known as max pooling operations. These on the other hand are memory bound, and their speed is limited by the CPU’s cache size and bandwidth of the bus. III. TRAINING LOW PRECISION DEEP NEURAL NETWORK This work was done as part of the coursework in ”Design of Arithmetic Typical implementations of deep neural networks using Processors” under the supervision of A. Nannarelli. gradient descent training method use 32-bit floating point conversions are needed, so that all the arithmetic operations can be performed on primitives that can benefit from hardware acceleration. The following subsections cover how the conversions are being handled. Please note, that from now on, the notion of minifloat will be used to denote a non-standard low precision floating point number such as 8- or 12-bit float. Fig. 1. Representation of low precision floating points used C. Float ! minifloat conversion In ordert to convert 32-bit floating point number to mini- representation of real numbers. In order to prove that such float, the following steps need to be performed: high precision is not necessary for successful training and inference, the complete from-scratch C++ implementation of 1) Determine and set the sign bit LeNeT-5 has been created that would easily allow for switch- 2) Compute rounding of mantissa ing between underlying data type that is used for storing a) Determine guard, last, round and sticky bits image data, weights, and all intermediaries. All the operations b) Compute G(L + R + T ) and save result to r performed on the data use single-precision floating point 3) Shift float mantissa right by (23 − S) and save to m arithmetic. Therefore the type representing floating point of 4) Save the minifloat mantissa mm as a sum of obtained variable precision had to have its conversion operators from- shift m and the rounding r and to-32-bit float defined. These, along with other details on 5) Determine if overflow has occured using equation implementation have been presented in the following section. o = r · m 6) Extract the float exponent ef and subtract the float 7 A. Low-precision floating point bias er = ef − (2 − 1) to obtain the raw exponent er Floating point format is an industry standard of repre- 7) Compute minifloat exponent em by adding the mini- senting real numbers. A single number consists of a sign, float bias and an mantissa overflow flag em = er + exponent and finally a mantissa, all portrayed in the Figure (2E−1 − 1) + o 1. Exponent is used to allow for representing a wide range of 8) If em < 0: the real numbers, while mantissa serves for assuring adequate a) Determine the value of the guard bit (the precision. A real number computed from its floating point most significant bit that will be truncated) format can be computed based on the following formula: and store it in Gu sign mantissa exponent−bias value = (−1) × (1 + 2M ) × 2 b) Compute the new mantissa using equation S em S (mm +2 )·2 +Gu where 2 is the hidden where M is the amount of bits used to represent mantissa, em E−1 bit, 2 shifts the value right by an absolute while bias is equal to 2 − 1, E being the number of bits value of minifloat exponent, and addition of used for exponent. Gu approximates rounding e Bit-width Exponent bits Mantisa bits c) Set minifloat exponent m to zero E 32 8 23 Else if em > 2 − 1: 16 5 10 12 5 6 a) Based on the sign, set the number to positive 8 4 3 or negative infinity, by setting exponent to (2E − 1) and mantissa to 0 TABLE I. PRECISION USED DURING TRAINING AND INFERENCE Where S and E are the bit lengths of minifloat significant and exponent respectively. Table I shows all four representations that have been subject to neural network training and testing during the D.
Details
-
File Typepdf
-
Upload Time-
-
Content LanguagesEnglish
-
Upload UserAnonymous/Not logged-in
-
File Pages5 Page
-
File Size-