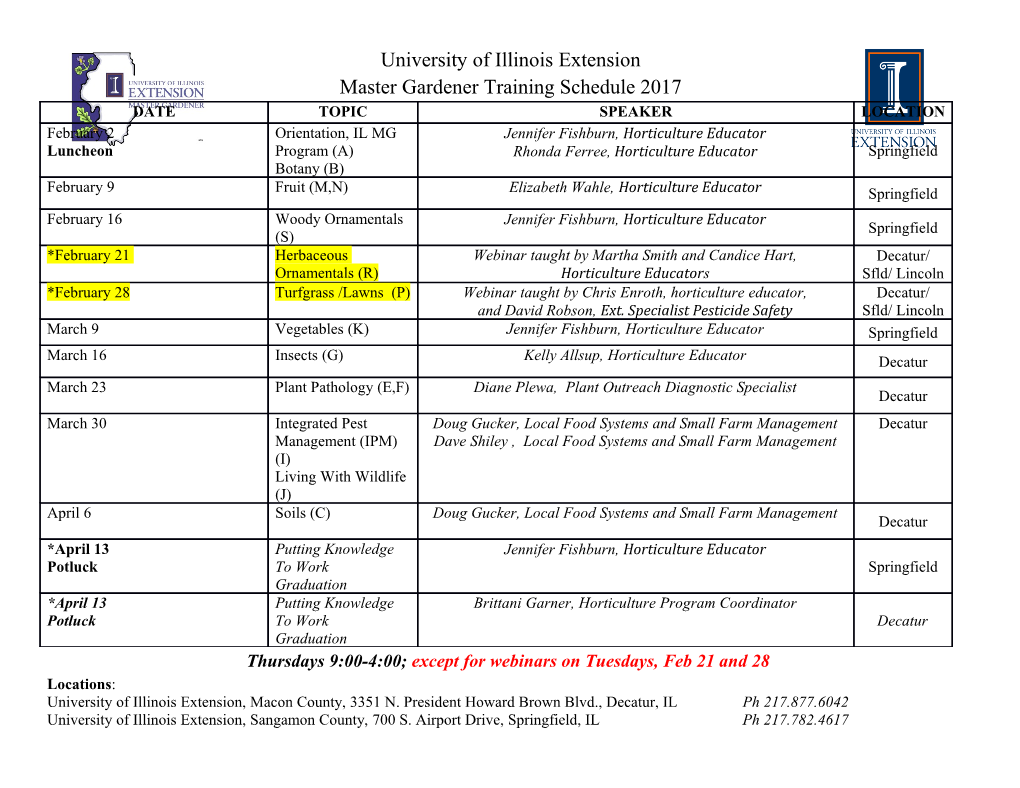
SHA532: Forecasting and Availability Controls in Hotel Revenue Management Copyright © 2012 eCornell. All rights reserved. All other copyrights, trademarks, trade names, and logos are the sole property of their respective owners. 1 This course includes Three self-check quizzes Multiple discussions; you must participate in two One final action plan assignment One video transcript file Completing all of the coursework should take about five to seven hours. What You'll Learn To explain the role of forecasting in hotel revenue management To create a forecast and measure its accuracy To recommend room rates To apply length-of-stay controls to your hotel Course Description Successful revenue management strategies hinge on the ability to forecast demand and to control room availability and length of stay. This course, produced in partnership with the Cornell School of Hotel Administration, explores the role of the forecast in a revenue management strategy and the positive impact that forecasting can also have on staff scheduling and purchasing. This course presents a step-by-step approach to creating an accurate forecast. You'll learn how to build booking curves; account for "pick-up"; segment demand by market, group, and channel; and calculate error and account for its impact. Sheryl Kimes Copyright © 2012 eCornell. All rights reserved. All other copyrights, trademarks, trade names, and logos are the sole property of their respective owners. 2 Professor of Operations Management, School of Hotel Administration, Cornell University Sheryl E. Kimes is a professor of operations management at the School of Hotel Administration. From 2005-2006, she served as interim dean of the School and from 2001-2005, she served as the school's director of graduate studies. Kimes teaches restaurant revenue management, yield management and food and beverage management. She has been named the school's graduate teacher of the year three times. Her research interests include revenue management and forecasting in the restaurant, hotel and golf industries. She has published over 50 articles in leading journals such as Interfaces, Journal of Operations Management , Journal of Service Research , Decision Sciences , and the Cornell Hospitality Quarterly . She has served as a consultant to many Click Play to Listen hospitality enterprises around the world, including Chevy's FreshMex Restaurants, Walt Disney World Resorts, Ruby's Diners, Starwood Asia-Pacific and Troon Golf. Kimes earned her doctorate in Operations Management in 1987 from the University of Texas at Austin. Start Your Course Copyright © 2012 eCornell. All rights reserved. All other copyrights, trademarks, trade names, and logos are the sole property of their respective owners. 3 Module Introduction: Creating a Forecast Your ability to manage demand successfully and maximize revenue will depend on the accuracy of your forecasts. In this module, find out why forecasts are so important to revenue management. Learn to create targeted, accurate forecasts that provide the information you need to manage revenue. When you have completed this module, you will be able to: Relate accurate forecasting to revenue management Define unconstrained demand Describe two different types of forecasts Build a booking curve Create a pickup forecast Explain why forecasting is done by group Create a forecast by group Discuss the impact of errors on forecasts Calculate error using the MAD and MAPE methods Copyright © 2012 eCornell. All rights reserved. All other copyrights, trademarks, trade names, and logos are the sole property of their respective owners. 4 Read: Ambiguity at the Random Stays Hotel In revenue management, your goal is to work with demand and the resources you have to maximize your revenue. To do this effectively, you need to understand the subtleties of constrained and unconstrained demand and why accurate forecasting is a necessary part of any revenue management system. The management team at the Random Stays Hotel is not sure how many guests might arrive on a given day, when they might arrive, or when they might leave. Team members are beginning to suspect that their lack of information is compromising their ability to make good management decisions and may even be contributing to their inability to reach their revenue goals. Their monthly reports indicate problems with labor scheduling, food purchasing, and guest-room occupancy levels. The team is wondering what to do next. One team member suggests they start forecasting arrivals. Though some nod in agreement, others protest. Forecasting seems like a lot of bother! And besides, managers who have been there awhile know how many arrivals to expect, more or less. Why would it matter if their estimates were a little too high or too low? Why should they go to the trouble of creating a written forecast? Explore the activities in the following pages to find out. Copyright © 2012 eCornell. All rights reserved. All other copyrights, trademarks, trade names, and logos are the sole property of their respective owners. 5 Watch: Forecasting Accuracy A presentation containing audio appears below. Use this resource to develop an understanding of the importance of accurate forecasting. Copyright © 2012 eCornell. All rights reserved. All other copyrights, trademarks, trade names, and logos are the sole property of their respective owners. 6 Read: A Forecast Overview Accurate forecasts are essential to revenue management. Without a forecast, it's not possible to set availability controls wisely or to use these controls effectively to grow revenue. A good forecast is a first and necessary step in revenue management. Good forecasts are based on data. Let's take a look at some typical booking data and see how past performance provides a basis for forecast estimates. Figure 1 displays guest-stays data for one hundred Thursdays at a particular hotel. From this chart, you can see how actual numbers of stays vary week by week, though usually keeping close to an average value. This average value can be estimated by inspection or calculated mathematically. It can be used, along with other characteristics of the data, to forecast the number of room-nights on a Thursday in the future. Table 1 Historical Average Weekly Occupancies Forecast Week 1 2 3 4 5 6 7 9 Sun 75% 48% 81% 82% 82% 81% 82% 82% Mon 76% 79% 81% 81% 83% 84% 82% 84% Tue 71% 66% 44% 61% 80% 60% 61% 61% Wed 72% 68% 68% 65% 95% 68% 65% 68% Thu 70% 58% 68% 61% 59% 71% 64% 66% Fri 71% 78% 70% 74% 75% 89% 100% 95% Sat 80% 84% 87% 90% 91% 90% 97% 94% Table 1 above contains occupancy data aggregated by day for seven weeks. The manager of the hotel can use historical data like these to estimate room-nights for days and weeks in the future. The data provided here suggest something about future occupancy levels, but they also demonstrate how much these values can vary. If occupancies vary as much as they do here, how is it possible to create an accurate forecast? Copyright © 2012 eCornell. All rights reserved. All other copyrights, trademarks, trade names, and logos are the sole property of their respective owners. 7 Forecasts are estimates. You shouldn't expect them to be one hundred percent accurate. They tend to be more accurate when more data are available and when those data can be appropriately aggregated, or grouped. For the best results, occupancy data should always be grouped by day, but they can also be grouped by length of stay, by rate, and even by distribution channel or market segment. In addition, forecasts created for dates in the future can be made increasingly accurate as information is acquired closer to the target date. Forecast Variations Types of Forecasts Arrivals Forecast-An arrivals forecast includes guests arriving on the forecast day, but does not include guests arriving prior to the forecast day for multi-night stays. Room-Nights Forecast-A room-night forecast includes guests arriving on the forecast day and guests arriving prior to the forecast day for multi-night stays. Levels of Forecast Detail Day of week-Most forecasting is done by the day of the week, that is, a Monday forecast would be built using data from previous Mondays, a Tuesday forecast would be built using data from previous Tuesdays, and so on. Demand varies reliably by day of the week, with certain obvious exceptions (holidays, emergencies). Length of stay-For length-of-stay forecasting, individual forecasts are created for each stay length (one-night, two-night, three-night, etc.). Rate class-For a hotel that offers more than one rate class (for instance, rack rate, discount, or super discount), forecasts can be created for each rate class individually. Inventory type-In the case of hotel-room forecasting, a forecast by inventory type might be characterized by king- or queen-sized beds, private bath, or view. Aggregate-Arrivals or room-nights are forecasted as one group, without regard to individual subgroups such as length of stay, rate class, or inventory type. Copyright © 2012 eCornell. All rights reserved. All other copyrights, trademarks, trade names, and logos are the sole property of their respective owners. 8 Read: Constrained and Unconstrained How many customers will come to your hotel two weeks from tomorrow? How about three weeks from tomorrow? How about two months? Your forecasts attempt to answer those questions and many others. You base your forecasts on data, using a picture of past demand to estimate demand in the future. But how accurate is your picture of past demand? Here we take a look at some of the issues involved in documenting and estimating demand. To a certain extent, past demand is reflected in the numbers of rooms sold. A large number of bookings indicates demand was high. Few bookings indicates demand was low. These data provide a rough estimate.
Details
-
File Typepdf
-
Upload Time-
-
Content LanguagesEnglish
-
Upload UserAnonymous/Not logged-in
-
File Pages40 Page
-
File Size-