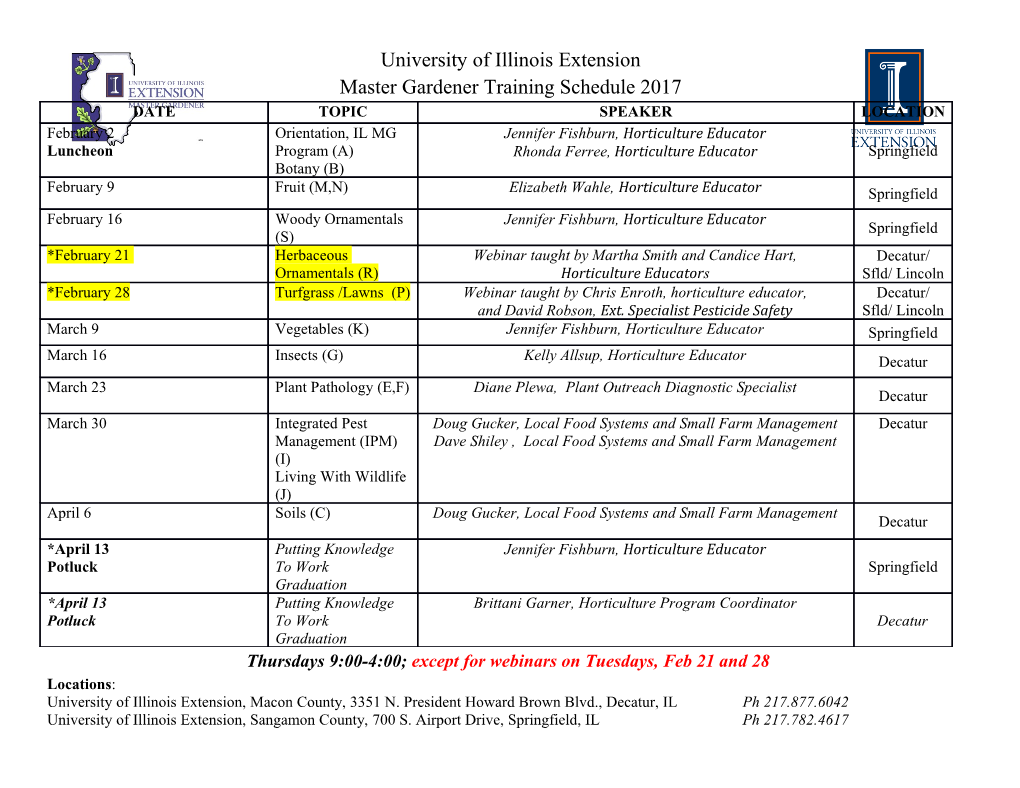
Julia The Tesla of Programing Languages The Sort Version "Flexible as Python Easy as Matlab Fast as Fortran Deep as Lisp" using Dates tonext(today()) do d dayofweek(d) == Fri && day(d) == 13 && month(d) == Feb end 2026-02-13 2 Two Language Problem Matlab Julia R Python Scala Interactive Java Rust, C Fortan Speed 3 Python is Slow But ... Pandas, NumPy and SciPy are really fast Because the computations are done in C Which can be faster than Julia code 4 Notable Uses Federal Reserve Bank Model of US Economy 10 times faster than MATLAB model Celeste Peak performance of 1.54 petaFLOPS using 1.3 million threads Only Julia, C, C++ and Fortran have achieved petaFLOPS computations Climate Modeling Alliance Caltech, MIT, Naval Postgraduate School, JPL coalition Using Julia to implement next generation global climate model 5 Why Julia Designed by and for people doing computational programming using current technology Interactive Fast Simple syntax Can call C/Fortran/Java/R/Python/Matlab code Libraries Statistics ML Web Graphics, etc Lisp-like macros Open Source - Free Paid support available 6 Will Look like Python Dynamic Typing a = 5 a = "cat" function foo(x) function bar(x::Int64)::Int64 y = 2 y::Int64 = 2 return x + y x + y end end foo(3) bar(3) foo(2.3) bar(2.3) Error MethodError: no method matching bar(::Float64) Closest candidates are: bar(!Matched::Int64) 7 Julia Superpower #1 Julia Jit compiler generates efficient code without type type annotations There are situations to avoid function foo(x) y = 2 return x + y end 8 function inc(x) Σ(x, y) = x + y return x + 1 Use LaTeX for symbol Type \ select from suggestions end Σ(1,5) function inc(x) δ = 0.003 x + 1 end inc(x) = x + 1 f(x, y) = 2(x + 3) - 3y 9 Superpower #2 Multiple Dynamic Dispatch f(x) = "general" f("cat") # "general" f(x::Number) = "general number" f(12) # "int" f(x::Int64) = "int" f(12.3) # "Large float" f(x::Float32) = "Small float" f(2//3) # "general number" (2//3 rational) f(x::Float64) = "Large float" 1//3 + 1//7 == 10//21 methods(f) # 6 methods for generic function "f": [1] f(x::Float64) in Main at /Users/whitney/Courses/696/Spring20/JuliaExamples/intro.jl:86 [2] f(x::Float32) in Main at /Users/whitney/Courses/696/Spring20/JuliaExamples/intro.jl:85 [3] f(x::Int64) in Main at /Users/whitney/Courses/696/Spring20/JuliaExamples/intro.jl:84 [4] f(x::Number) in Main at /Users/whitney/Courses/696/Spring20/JuliaExamples/intro.jl:83 [5] f(x) in Main at /Users/whitney/Courses/696/Spring20/JuliaExamples/intro.jl:82 [6] f(x, y) in Main at /Users/whitney/Courses/696/Spring20/JuliaExamples/intro.jl:76 10 Showing Multiple Dispatch g(x::Int64,y::Float64) = "int first" g(x::Float64,y::Int64) = "int last" a = 4 b = 3.2 g(a,b) # "int first" a = 1.2 b = 6 g(a,b) #"int last" 11 Broadcast function foo(x) bar(x) = x + (iseven(x) ? 2 : -2) if iseven(x) x + 2 else bar.(1:10) x - 2 end end 10-element Array{Int64,1}: -1 4 foo(2) # 4 1 6 foo(3) #1 3 8 foo.([1 2 3 4]) # [1 4 1 6] 5 10 7 12 12 Some History 1984 Matlab 1991 Python 2000 R - 1.0 2014 Julia 0.3 2018 Julia 1.0 2019 Dec 30 Julia 1.3.1 13 Julia Calling Python Code using Pkg Pkg.add("Conda") Pkg.add("PyCall") #installing - done once using Conda using PyCall math = pyimport("math") #Using Python math module math.sin(math.pi / 4) Conda.add("matplotlib") plt = pyimport("matplotlib.pyplot") # Using Python matplotlib x = range(0; stop=2*pi, length=1000) y = sin.(3*x + 4*cos.(2*x)); plt.plot(x, y, color="red", linewidth=2.0, linestyle="--") plt.show() 14 Julia Calling Python Code Conda.add("matplotlib") plt = pyimport("matplotlib.pyplot") # Using Python matplotlib x = range(0; stop=2*pi, length=1000) y = sin.(3*x + 4*cos.(2*x)); plt.plot(x, y, color="red", linewidth=2.0, linestyle="--") plt.show() 15 Julia and R Pkg.add("RCall") ENV["R_HOME"]="*" Pkg.build("RCall") # Done once y = 1 R"""f <- function(x, y) x + y using RCall ret <- f(1, $y) """ r_result = R"rnorm(10)" julia_result = rcopy(r_result) RObject{VecSxp} One Sample t-test julia_data = randn(10) r_result = R"t.test($julia_data)" data: `#JL`$julia_data t = -0.34784, df = 9, p-value = 0.736 alternative hypothesis: true mean is not equal to 0 R"optim(0, $(x -> x-cos(x)), method='BFGS')" 95 percent confidence interval: -0.9621269 0.7056758 sample estimates: mean of x -0.1282256 16 Julia and R julia> using RCall Using REPL julia> foo = 1 Toggle between Julia and R 1 Type $ to activate R R> x <- $foo R> x [1] 1 R> y = $(rand(10)) R> sum(y) [1] 3.273645 R> backspace to leave R julia> 17 Data Science JuliaML – Machine Learning (Gitter) JuliaStats – Statistics JuliaImages – Image Processing JuliaText – Natural Language Processing (NLP) JuliaDatabases – Various database drivers for Julia JuliaData – Data manipulation, storage, and I/O in Julia Scientific Domains BioJulia – Biology (Gitter) EcoJulia – Ecology JuliaAstro – Astronomy (Gitter) JuliaDSP – Digital signal processing JuliaQuant – Finance JuliaPhysics – Physics JuliaDynamics - Dynamical systems, nonlinear dynamics and chaos. JuliaGeo – Earth science, geospatial data processing JuliaMolSim – Molecular Simulation in Materials Science and Chemistry JuliaReach - Reachability Computations for Dynamical Systems (Gitter) 18 Stats Packages StatsBase StatsModels DataFrames Distributions MultivariateStats HypothesisTests ML Distances KernelDensity Clustering Generalized linear models Nonnegative matrix factorization TimeSeries BayesNets 19 Julia Observer https://juliaobserver.com Find Julia packages 20 Should You Learn Julia? Researcher Just use existing libraries? Satisfied with current language/system? Student What are you interested in? 21 Getting Started Using Julia https://julialang.org 22 Installing Julia & IDE Easiest Way Julia Pro - free https://juliacomputing.com/products/juliapro More Involved Download Julia https://julialang.org/downloads/ Install Juno (Atom) https://junolab.org 23 Demo 24 Julia vs Python Python Everywhere Easy to use Libraries for everything Slow 3D finite difference time domain simulation of electromagnetic wave propagation EE graduate student - little programing experience in Python or Julia Naive Naive Optimized C Julia Python Julia Ave 0.426 22.6 145.2 0.620 Ratio to C 1.00 52.9 341.1 1.5 25 First Run Compiles Code function sumer(n) localsum = 0 for k in 1:n localsum = k + localsum end localsum end @time sumer(1_000_000) 0.002106 seconds (769 allocations: 44.500 KB) @time sumer(1_000_000) 0.000003 seconds (5 allocations: 176 bytes) 26 Use Functions - Top level treated differently const N = 1_000_000 sum = 0 Time Memory @time for k = 1:N 0.05 sec 30.5 MB sum = sum + k end function sumer(n) localsum = 0 for k in 1:n localsum = k + localsum Time Memory end 0.000003 sec 176 bytes localsum end @time sumer(N) 27 Avoid changing the type of a variable function sumerbad(n) @time sumerbad(N) localsum = 0 # Int for k in 1:0.5:n÷2 Time Memory localsum = k + localsum # now float 0.058 sec 30.5 MB end localsum end function sumergood(n) @time sumergood(N) localsum = 0.0 # float for k in 1:0.5:n÷2 Time Memory localsum = k + localsum # float 0.004 sec 176 bytes end localsum end 28 Type Stability Return type of a function should only depend on the type of its arguments Compiler can generate efficient code for type stable functions pos(x) = x < 0 ? 0 : x pos(2.3) returns a float pos(-2.3) returns an integer pos(x) = x < x ? zero(x) : x pos(2.3) returns a float pos(-2.3) returns a float Type stable 25% faster 29 Julia Parallel Processing Low level constructs High level constructs Runs on Multicore processors Clusters Cluster management Experimental Julia to C/C++ compilers from Intel Labs Run Julia code 20 to 100 times faster than Spark 30 Low Level Parallel Constructs addprocs(2) remote = @spawn rand(2,2) fetch(remote) addprocs(10) Each worker will sum 10_000 @parallel (+) for k = 1:100_000 random numbers rand(1) end Master will sum up the 10 results Assuming you have 10 processors 31 High Level Parallel using DistributedArrays onmaster = rand(100,100) distributed = distribute(onmaster) #distribute onmaster to the workers sum(distributed) # compute sum locally on workers # combine the result on master heads = map(x -> x > 0.5, distributed) # apply map on workers # return result on master 32.
Details
-
File Typepdf
-
Upload Time-
-
Content LanguagesEnglish
-
Upload UserAnonymous/Not logged-in
-
File Pages32 Page
-
File Size-