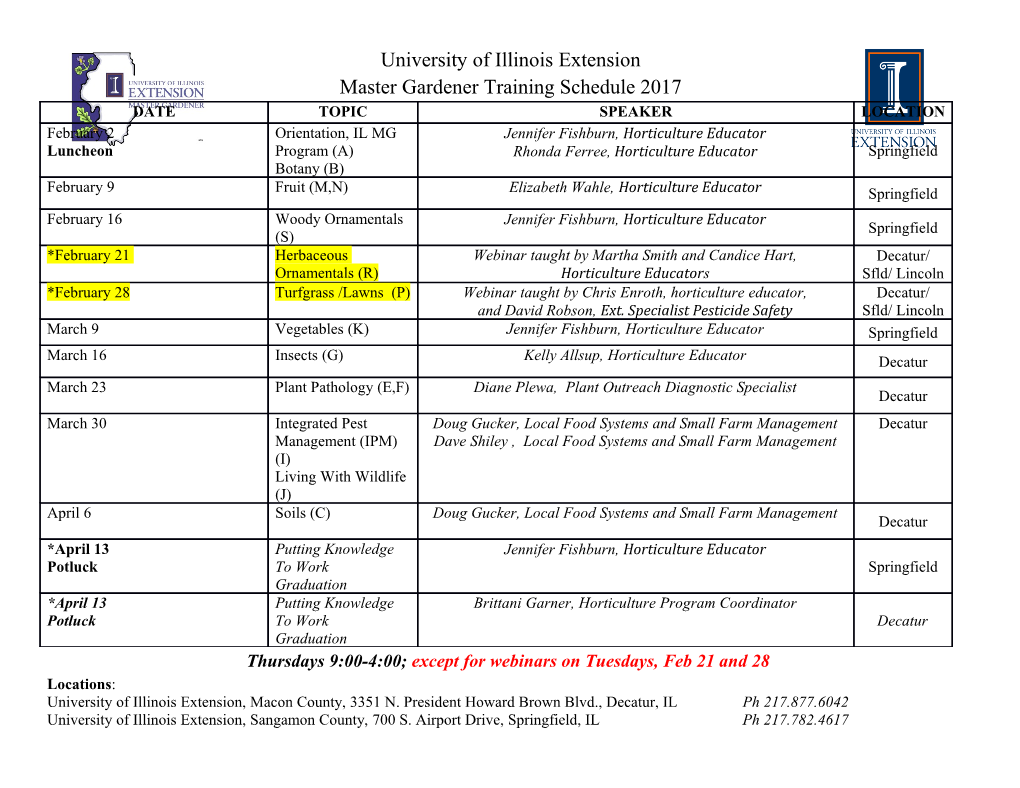
Shrinkage Econ 2148, fall 2017 Shrinkage in the Normal means model Maximilian Kasy Department of Economics, Harvard University 1 / 47 Shrinkage Agenda I Setup: the Normal means model X ∼ N(q;Ik ) and the canonical estimation problem with loss kqb − qk2. I The James-Stein (JS) shrinkage estimator. I Three ways to arrive at the JS estimator (almost): 1. Reverse regression of qi on Xi . 2. Empirical Bayes: random effects model for qi . 3. Shrinkage factor minimizing Stein’s Unbiased Risk Estimate. I Proof that JS uniformly dominates X as estimator of q. I The Normal means model as asymptotic approximation. 2 / 47 Shrinkage Takeaways for this part of class I Shrinkage estimators trade off variance and bias. I In multi-dimensional problems, we can estimate the optimal degree of shrinkage. I Three intuitions that lead to the JS-estimator: 1. Predict qi given Xi ) reverse regression. 2. Estimate distribution of the qi ) empirical Bayes. 3. Find shrinkage factor that minimizes estimated risk. I Some calculus allows us to derive the risk of JS-shrinkage ) better than MLE, no matter what the true q is. I The Normal means model is more general than it seems: large sample approximation to any parametric estimation problem. 3 / 47 Shrinkage The Normal means model The Normal means model Setup k I q 2 R I e ∼ N(0;Ik ) I X = q + e ∼ N(q;Ik ) I Estimator: qb = qb(X) I Loss: squared error 2 L(qb;q) = ∑(qbi − qi ) i I Risk: mean squared error h i h 2i R(qb;q) = Eq L(qb;q) = ∑Eq (qbi − qi ) : i 4 / 47 Shrinkage The Normal means model Two estimators I Canonical estimator: maximum likelihood, ML qb = X I Risk function ML 2 R(qb ;q) = ∑Eq ei = k: i I James-Stein shrinkage estimator JS (k − 2)=k qb = 1 − · X: X 2 I Celebrated result: uniform risk dominance; for all q JS ML R(qb ;q) < R(qb ;q) = k: 5 / 47 Shrinkage Regression perspective First motivation of JS: Regression perspective I We will discuss three ways to motivate the JS-estimator (up to degrees of freedom correction). I Consider estimators of the form qbi = c · Xi or qbi = a + b · Xi : I How to choose c or (a;b)? I Two particular possibilities: 1. Maximum likelihood: c = 1 (k−2)=k 2. James-Stein: c = 1 − X 2 6 / 47 Shrinkage Regression perspective Practice problem (Infeasible estimator) I Suppose you knew X1;:::;Xk as well as q1;:::;qk , I but are constrained to use an estimator of the form qbi = c · Xi . 1. Find the value of c that minimizes loss. 2. For estimators of the form qbi = a + b · Xi , find the values of a and b that minimize loss. 7 / 47 Shrinkage Regression perspective Solution I First problem: ∗ 2 c = argmin ∑(c · Xi − qi ) c i I Least squares problem! I First order condition: ∗ 0 = ∑(c · Xi − qi ) · Xi : i I Solution ∗ ∑Xi qi c = 2 : ∑i Xi 8 / 47 Shrinkage Regression perspective Solution continued I Second problem: ∗ ∗ 2 (a ;b ) = argmin ∑(a + b · Xi − qi ) a;b i I Least squares problem again! I First order conditions: ∗ ∗ 0 = ∑(a + b · Xi − qi ) i ∗ ∗ 0 = ∑(a + b · Xi − qi ) · Xi : i I Solution ∑(Xi − X) · (qi − q) sXq b∗ = = ; a∗ + b∗ · X = q 2 2 ∑i (Xi − X) sX 9 / 47 Shrinkage Regression perspective Regression and reverse regression I Recall Xi = qi + ei , E[ei jqi ] = 0, Var(ei ) = 1. I Regression of X on q: Slope sXq seq 2 = 1 + 2 ≈ 1: sq sq I For optimal shrinkage, we want to predict q given X, not the other way around! I Reverse regression of q on X: Slope s s2 + s s2 Xq = q eq ≈ q : 2 2 2 2 sX sq + 2seq + se sq + 1 I Interpretation: “signal to (signal plus noise) ratio” < 1. 10 / 47 Shrinkage Regression perspective Illustration 148 S. M. STIGLER Floridabe usedto improvean estimateof the price of Frenchwine, when it is assumedthat they are unre- lated? The best heuristicexplanation that has been offeredis a Bayesianargument: If the 0i are a priori independentN(0, r2), thenthe posterior mean of Oi is ofthe sameform as OJ, and henceO'J can be viewed as an empiricalBayes estimator(Efron and Morris, (9i,X,) I@ * -' 1973;Lehmann, 1983, page 299). Anotherexplanation 8~~~~~~(iX,)- thathas been offeredis that0JS can be viewedas a relativeof a "pre-test"estimator; if one performsa preliminarytest of the null hypothesis that 0 = 0, and one then uses 0 = 0 or 0i = Xi dependingon the outcomeof the test, the resultingestimator is a o~~~ x weightedaverage of 0 and00 ofwhich 0Js is a smoothed version(Lehmann, 1983, pages 295-296). But neither of these explanationsis fullysatisfactory (although bothhelp render the resultmore plausible); the first becauseit requiresspecial a prioriassumptions where Stein did not,the secondbecause it correspondsto the resultonly in the loosestqualitative way. The FIG. 1. Hypotheticalbivariate plot of Oi vs. Xi, fori =1, * ,k. difficultyof understandingthe Steinparadox is com- 11 / 47 poundedby the fact that its proof usually depends on the of means (, )to lie nearthe 45? explicitcomputation of the risk function or the theory expect point line. of completesufficient statistics, by a processthat all convincesus of its truthwithout really illuminating Now our goal is to estimate of the Oi's given all the reasonsthat it works.(The best presentationI of the Xi's, with no assumptions about a possible knowis thatin Lehmann(1983, pages 300-302)of a dfistributionalstructure for the Oi's-they are simply proofdue to Efronand Morris(1973); Berger(1980, to be viewed as unknown constants. Nonetheless, to page 165,example 54) outlinesa shortbut unintuitive 3eewhy we should expect that the ordinaryestimator O' proof;the one shorterproof I have encounteredin a can be improvedupon, it helps to thinkabout what if textbookis vitiatedby a majornoncorrectable error.) we would do this were not the case. If the Oi's,and The purposeof thispaper is to showhow a different hencethe pairs (Xi, Oi), had a knownjoint distribution, a natural (and in some settingseven optimal) method perspective,one developedby FrancisGalton over a setig hreth -fdono ---0aditibt -n of With no di~stiuinlasmtosaothe',wouldbe to calculate6 = E centuryago (Stigler,1986, chapter 8), can renderthe proceeding (X) (O I X) resulttransparent, as' well as lead to a simple,full and use this, the theoreticalregression function of 0 on to estimatesof the it proof.This perspectiveis perhapscloser to thatof the X, generate Oi'sby evaluating foreach We think of this as an unattainable periodbefore 1950 than to subsequentapproaches, but Xi. may it has points in commonwith more recentworks, ideal, unattainable because we do not know the con- ditional distributionof 0 X. we will not particularlythose of Efronand Morris(1973), Rubin given Indeed, assume that our about the unknowncon- (1980),Dempster (1980) and Robbins(1983). uncertainty stants Oican be describedby a probabilitydistribution 2. STEIN ESTIMATIONAS A REGRESSION at all; our view is not that of eitherthe Bayesian or We do know the condi- PROBLEM empiricalBayesian approach. tional distributionof X given 0, namely N(O, 1), and The estimationproblem involves pairs of values we can calculate E (X I 0) = 0. Indeed this, the theo- (Xi, Os), i = 1, ** , k,where one element of each pair retical regressionline of X on 0, correspondsto the (Xi) is knownand one (Oi) is unknown.Since the Oi's line 0 = X in Figure 1, and it is this line which gives are unknown,the pairs cannot in factbe plotted,but the ordinaryestimators 09? = Xi. Thus the ordinary it will help our understandingof the problemand estimator may be viewed as being based on the suggesta meansof approaching it ifwe imaginewhat "4wrong"regression line, on E (XI l ) rather than sucha plot wouldlook like.Figure 1 is hypothetical, E(O I X). Since, as Francis Galton alreadyknew in the butsome aspects of it accuratelyreflect the situation. 1880's, the regressionsof X on 0 and of 0 on X can be Since X is N(O, 1), we can thinkof the X's as being markedlydifferent, this suggests that the ordinary generatedby addingN(O, 1) "errors"to the givenO's. estimatorcan be improvedupon and even suggests Thus thehorizontal deviations of the points from the how this mightbe done-by attemptingto approxi- 450 line 0 = X are independentN(O, 1), and in that mate "E(O I X) "-or whateverthat mightmean in a respectthey should cluster around the line as indi- cated.Also, E(X) = Wand Var(X) = 1/k, so we should This content downloaded from 128.103.149.52 on Thu, 2 Oct 2014 09:21:26 AM All use subject to JSTOR Terms and Conditions Shrinkage Regression perspective Expectations Practice problem 1. Calculate the expectations of 1 2 1 2 X = k ∑Xi ; X = k ∑Xi ; i i and 2 1 2 2 2 sX = k ∑(Xi − X) = X − X i 2. Calculate the expected numerator and denominator of c∗ and b∗. 12 / 47 Shrinkage Regression perspective Solution I E[X] = q I E[X 2] = q 2 + 1 2 2 2 2 I E[sX ] = q − q + 1 = sq + 1 ∗ I c = (Xq)=(X 2), and E[Xq] = q 2. Thus q 2 c∗ ≈ : q 2 + 1 ∗ 2 2 I b = sXq =sX , and E[sXq ] = sq . Thus 2 ∗ sq b ≈ 2 : sq + 1 13 / 47 Shrinkage Regression perspective Feasible analog estimators Practice problem Propose feasible estimators of c∗ and b∗. 14 / 47 Shrinkage Regression perspective A solution I Recall: ∗ Xq I c = X 2 2 I qe ≈ 0, e ≈ 1. I Since Xi = qi + ei , Xq = X 2 − Xe = X 2 − qe − e2 ≈ X 2 − 1 I Thus: 2 2 2 ∗ X − qe − e X − 1 1 c = ≈ = 1 − =: bc: X 2 X 2 X 2 15 / 47 Shrinkage Regression perspective Solution continued I Similarly: ∗ sXq I b = 2 sX 2 I sqe ≈ 0, se ≈ 1.
Details
-
File Typepdf
-
Upload Time-
-
Content LanguagesEnglish
-
Upload UserAnonymous/Not logged-in
-
File Pages47 Page
-
File Size-