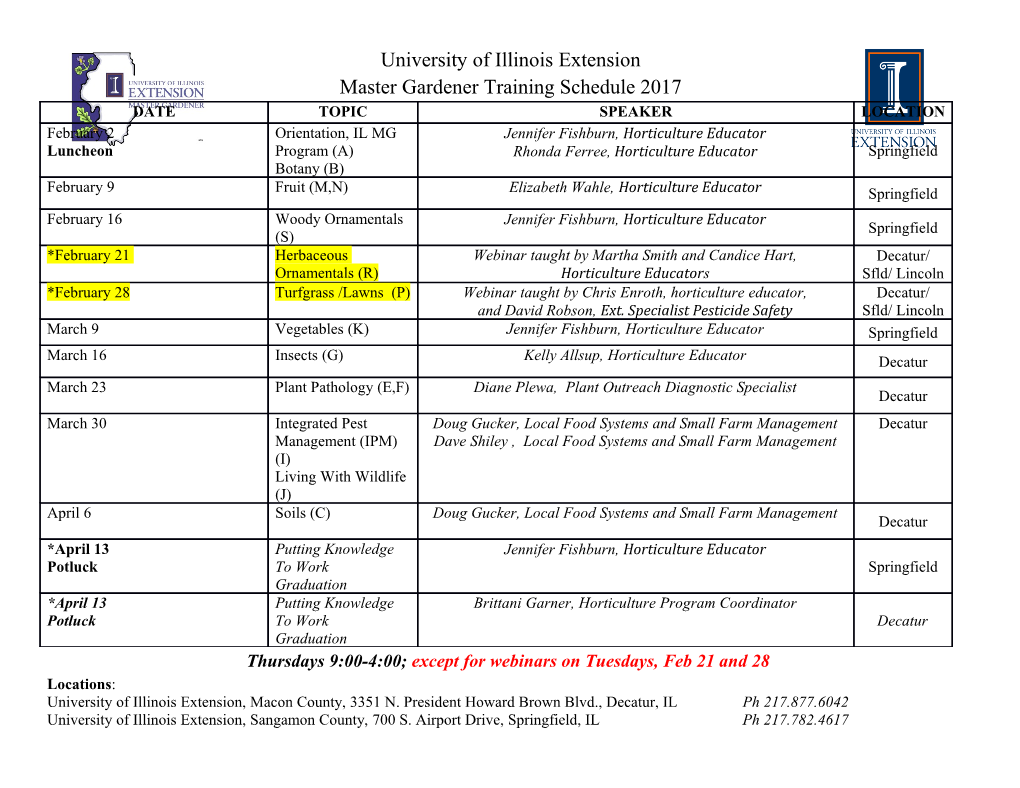
Maximum Likelihood Estimators February 22, 2016 Debdeep Pati 1 Maximum Likelihood Estimation Assume X ∼ Pθ; θ 2 Θ, with joint pdf (or pmf) f(x j θ). Suppose we observe X = x. The Likelihood function is L(θ j x) = f(x j θ) as a function of θ (with the data x held fixed). The likelihood function L(θ j x) and joint pdf f(x j θ) are the same except that f(x j θ) is generally viewed as a function of x with θ held fixed, and L(θ j x) as a function of θ with x held fixed. f(x j θ) is a density in x for each fixed θ. But L(θ j x) is not a density (or mass function) in θ for fixed x (except by coincidence). 1.1 The Maximum Likelihood Estimator (MLE) A point estimator θ^ = θ^(x) is a MLE for θ if L(θ^ j x) = sup L(θ j x); θ that is, θ^ maximizes the likelihood. In most cases, the maximum is achieved at a unique value, and we can refer to \the" MLE, and write ^ θ(x) = argmaxθL(θjx): (But there are cases where the likelihood has flat spots and the MLE is not unique.) 1.2 Motivation for MLE's Note: We often write L(θ j x) = L(θ), suppressing x, which is kept fixed at the observed n data. Suppose x 2 R . Discrete Case: If f(· j θ) is a mass function (X is discrete), then L(θ) = f(x j θ) = Pθ(X = x): L(θ) is the probability of getting the observed data x when the parameter value is θ. n Continuous Case: When f(· j θ) is a continuous density Pθ(X = x) = 0, but if B ⊂ R is a very, very small ball (or cube) centered at the observed data x, then Pθ(X 2 B) ≈ f(x j θ) × Volume(B) / L(θ): 1 L(θ) is proportional to the probability the random data X will be close to the observed data x when the parameter value is θ. Thus, the MLE θ^ is the value of θ which makes the observed data x \most probable". To find θ^, we maximize L(θ). This is usually done by calculus (finding a stationary point), but not always. If the parameter space Θ contains endpoints or boundary points, the maximum can be achieved at a boundary point without being a stationary point. If L(θ) is not \smooth" (continuous and everywhere differentiable), the maximum does not have to be achieved at a stationary point. Cautionary Example: Suppose X1;:::;Xn are iid Uniform(0; θ) and Θ = (0; 1). Given data x = (x1; : : : ; xn), find the MLE for θ. n Y −1 −n L(θ) = θ I(0 < xi < θ) = θ I(0 ≤ x(1))I(x(n) ≤ θ) i=1 ( θ−n for θ ≥ x = (n) 0 for 0 < θ < x(n) which is maximized at θ = x(n), which is a point of discontinuity and certainly not a ^ stationary point. Thus, the MLE is θ = x(n). Notes: L(θ) = 0 for θ < x(n) is just saying that these values of θ are absolutely ruled out by the data (which is obvious). A strange property of the MLE in this example (not typical): ^ Pθ(θ < θ) = 1 The MLE is biased; it is always less than the true value. A Similar Example: Let X1;:::;Xn be iid Uniform(α; β) and Θ = f(α; β): α < βg. Given data x = (x1; : : : ; xn), find the MLE for θ = (α; β). n Y −1 −n L(α; β) = (β − α) I(α < xi < β) = (β − α) I(α ≤ x(1))I(x(n) ≤ β) i=1 ( (β − α)−n for α ≤ x ; x ≤ β = (1) (n) 0 otherwise which is maximized by making β − α as small as possible without entering \0 otherwise" region. Clearly, the maximum is achieved at (α; β) = (x(1); x(n)). Thus the MLE is ^ ^ θ = (^α; β) = (x(1); x(n)). Again, Pα,β(α < α;^ β < β) = 1. 2 2 Maximizing the Likelihood (one parameter) 2.1 General Remarks Basic Result: A continuous function g(θ) defined on a closed, bounded interval J attains its supremum (but might do so at one of the endpoints). (That is, there exists a point θ0 2 J such that g(θ0) = supθ2J g(θ). ) Consequence: Suppose g(θ) is a continuous, non-negative function defined on an open interval J = (c; d) (where perhaps c = −∞ or d = 1). If lim g(θ) = lim g(θ) = 0; θ!c θ!d then g attains its supremum. Thus, MLEs usually exist when the likelihood function is continuous. 3 Maxima at Stationary Points Suppose the function g(θ) is defined on an interval Θ (which may be open or closed, infinite or finite). If g is differentiable and attains its supremum at a point θ0 in the interior of Θ, 0 that point must be a stationary point (that is, g (θ0) = 0). 0 00 1. If g (θ0) = 0 and g (θ0) < 0, then θ0 is a local maximum (but might not be the global maximum). 0 00 2. If g (θ0) = 0 and g (θ) < 0 for all θ 2 Θ, then θ0 is a global maximum (that is, it attains the supremum). The condition in (1) is necessary (but not sufficient) for θ0 to be a global maximum. Condition (2) is sufficient (but not necessary). A function satisfying g00(θ) < 0 for all θ 2 Θ is called strictly concave. It lies below any tangent line. Another useful condition (sufficient, but not necessary) is: 0 0 3. If g (θ) > 0 for θ < θ0 and g (θ) < 0 for θ > θ0, then θ0 is a global maximum. 4 Maximizing the Likelihood (multi-parameter) 4.1 Basic Result: k A continuous function g(θ) defined on a closed, bounded set J ⊂ R attains its supremum (but might do so on the boundary). 3 4.2 Consequence: k Suppose g(θ) is a continuous, non-negative function defined for all θ 2 R . If g(θ) ! 0 as jjθjj ! 1, then g attains its supremum. Thus, MLEs usually exist when the likelihood function is continuous. k Suppose the function g(θ) is defined on a convex set Θ ⊂ R (that is, the line segment joining any two points in Θ lies entirely inside Θ). If g is differentiable and attains its supremum at a point θ0 in the interior of Θ, that point must be a stationary point: @g(θ ) 0 = 0; i = 1; 2; : : : ; k: @θi Define the vector D and Hessian matrix H: @g(θ)k D(θ) = (a k × 1 vector): @θi i=1 @2g(θ)k H(θ) = (a k × k matrix) @θi@θj i;j=1 4.3 Maxima at Stationary Points 1. If D(θ0) = 0 and H(θ0) is negative definite, then θ0 is a local maximum (but might not be the global maximum). 2. If D(θ0) = 0 and H(θ) is negative definite for all θ 2 Θ, then θ0 is a global maximum (that is, it attains the supremum). (1) is necessary (but not sufficient) for θ0 to be a global maximum. (2) is sufficient (but not necessary). A function for which H(θ) is negative definite for all θ 2 Θ is called strictly concave. It lies below any tangent plane. 4.4 Positive and Negative Definite Matrices Suppose M is a k × k symmetric matrix. Note: Hessian matrices and covariance matrices are symmetric. Definitions: 0 k 1. M is positive definite if x Mx > 0 for all x 6= 0 (x 2 R ). 2. M is negative definite if x0Mx < 0 for all x 6= 0. 4 0 k 3. M is non-negative definite (or positive semi-definite) if x Mx ≥ 0 for all x 2 R . Facts: 1. M is p.d. iff all its eigenvalues are positive. 2. M is n.d. iff all its eigenvalues are negative. 3. M is n.n.d. iff all its eigenvalues are non-negative. 4. M is p.d. iff −M is n.d. 5. If M is p.d., all its diagonal elements must be positive. 6. If M is n.d., all its diagonal elements must be negative. 7. The determinant of a symmetric matrix is equal to the product of its eigenvalues. 2 × 2 Symmetric Matrices: m11 m12 M = (mij) = ; m12 = m21 m21 m22 2 jMj = m11m22 − m12m21 = m11m22 − m12: A 2×2 matrix is p.d. when the determinant is positive and the diagonal elements are positive. A 2 × 2 matrix is n.d. when the determinant is positive and the diagonal elements are negative. The bare minimum you need to check: M is p.d. if m11 > 0 (or m22 > 0) and jMj > 0. M is n.d. if m11 < 0 (or m22 < 0) and jMj > 0. Example: Observe X1;X2;:::;Xn be iid Gamma(α; β). Preliminaries: n Y xα−1e−xi/β L(α; β) = i βαΓ(α) i=1 Maximizing L is same as maximzing l = log L given by l(α; β) = (α − 1)T1 − T2/β − nα log β − n log Γ(α) 5 P P where T1 = i log xi;T2 = i xi. Note that T = (T1;T2) is the natural sufficient statistic of this 2pef. @l d Γ0(α) = T − n log β − n (α); (α) ≡ log Γ(α) = @α 1 dα Γ(α) @l T nα 1 = 2 − = (T − nαβ) @β β2 β β2 2 @2l = −n 0(α) @α2 @2l −2T nα −1 = 2 + = (2T − nαβ) @β2 β3 β2 β3 2 @2l −n = @α@β β Situation #1: Suppose α = α0 is known.
Details
-
File Typepdf
-
Upload Time-
-
Content LanguagesEnglish
-
Upload UserAnonymous/Not logged-in
-
File Pages15 Page
-
File Size-