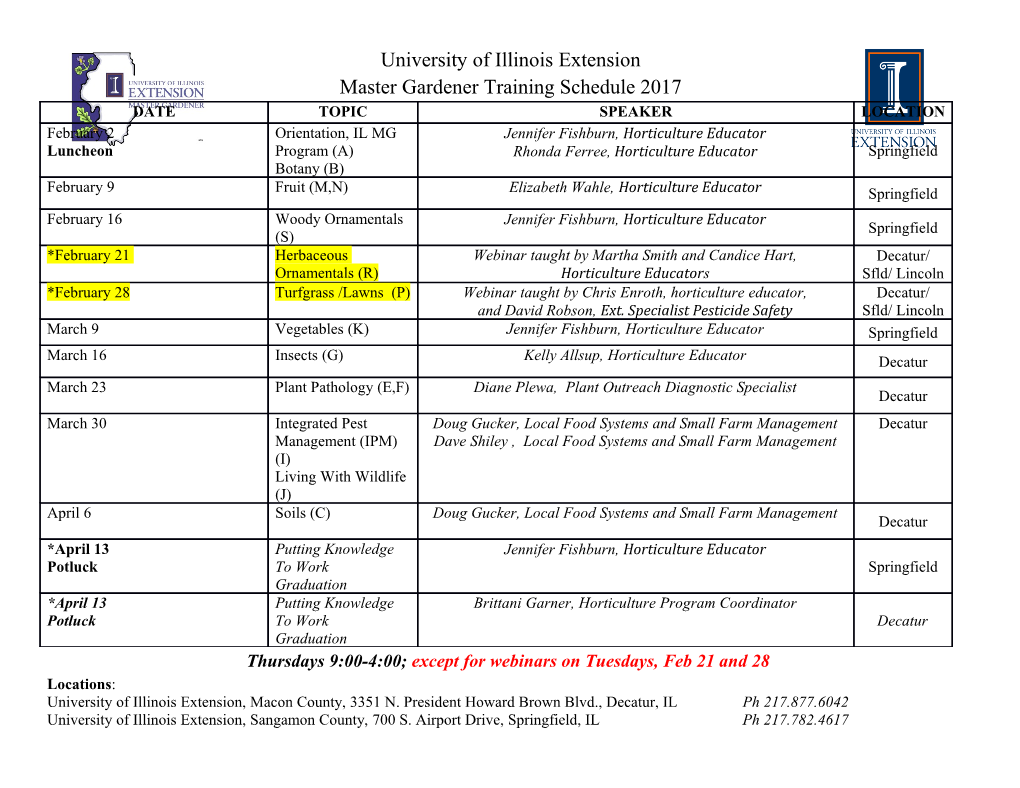
Extended abstract AMS 89 th Annual Meeting 11-15 January 2009, Phoenix, AZ 14.3 EVALUATION OF A NEW MULTIPLE-DOPPLER TORNADO DETECTION AND CHARACTERIZATION TECHNIQUE USING REAL RADAR OBSERVATIONS Corey K. Potvin* ,1 , Alan Shapiro 1, Tian-You Yu 2, and Jidong Gao 3 1School of Meteorology, University of Oklahoma, Norman, OK 2School of Electrical and Computer Engineering, University of Oklahoma, Norman, OK 3Center for Analysis and Prediction of Storms, University of Oklahoma, Norman, OK 1. INTRODUCTION tive Adaptive Sensing of the Atmosphere; Mclaughlin et al. 2005; Brotzge et al. 2007) radar Since the implementation of the WSR-88D network to detect small-scale vortices and also to network, several algorithms have been developed provide vortex characteristic estimates which may to aid forecasters in real-time identification of in- improve tornado nowcasting. The vortex para- tense small and mesoscale vortices. The National meters are obtained by minimizing a cost function Severe Storms Laboratory (NSSL) Mesocyclone which measures the discrepancy between the ob- Detection Algorithm (MDA; Stumpf et al. 1998) served and model radial wind fields. By taking the was designed to alert forecasters to the presence translation of the system into account, the radar of supercell thunderstorms, which produce a large data can be used at their actual locations and portion of tornadoes in the United States. The times of acquisition. NSSL Tornado Detection Algorithm (TDA; Mitchell Tests of the technique using analytically- et al. 1998) calculates azimuthal shear of radial generated and numerically-simulated tornadic wind using adjacent radar resolution volumes, and wind fields can be found in Potvin et al. (2008). In identifies regions where shear exceeds a thre- this paper, the technique is applied to real dual- shold. Unfortunately, the success of the TDA al- Doppler observations of tornadoes. The low-order gorithm and others of its kind [e.g., Tornado Vor- model is introduced in section 2. The computation tex Signature (TVS) algorithm, Crum and Alberty and minimization of the cost function is described 1993] depends upon the chosen detection thre- in section 3. Section 4 describes tests using sholds, the suitability of which is largely range- WSR-88D observations of an F4 tornado which and storm-dependent. Thus, this approach may struck central Oklahoma on 8 May 2003. Section be subject to high false alarm rate or low probabili- 5 describes tests using high-resolution Doppler- ty of detection values. on-Wheels observations of a relatively small tor- The Velocity Track Display (VTD) technique nado. A summary and plans for future work follow and its variants (Lee et al. 1994; Roux and Marks in section 6. 1996; Lee et al. 1999; Liou et al. 2006) were de- veloped to retrieve the three-dimensional velocity field of a specific class of meteorologically signifi- 2. DESCRIPTION OF LOW-ORDER MODEL cant flows: intense vortices. These techniques fit radial velocity data to a vortex model in order to The low-order model used in this study is recover key characteristics of the vortex flow. This comprised of four idealized flow fields: a uniform capability distinguishes this approach from tradi- flow, linear shear flow, linear divergence flow, and tional dual-Doppler analysis, which does not con- modified combined Rankine vortex (MCRV; strain the retrieved wind field with a spatial vortex representing the tornado). The vortex and its envi- model and thus is not designed to retrieve vortex ronment are allowed to translate. Our use of the characteristics. Our method also adopts a vortex- MCRV model is supported qualitatively by high- fitting approach. More specifically, radial wind ob- resolution mobile radar observations of tornadoes servations from two or more close-proximity Dopp- whose azimuthally-averaged tangential winds ler radars with overlapping domains are fit to an roughly followed this profile (Wurman and Gill analytical low-order model of a vortex and near- 2000; Bluestein et al. 2003; Lee and Wurman environment. The model control parameters in- 2005). clude vortex location, size, intensity, and transla- The Cartesian components of the linear flow tion velocity. Our method is designed to capitalize fields (broadscale flow) are given by upon the increased observational density and overlapping coverage of a CASA-like (Collabora- 1 Extended abstract AMS 89 th Annual Meeting 11-15 January 2009, Phoenix, AZ Vx=+ a by( − vt b ) + cx-ut), ( b To facilitate calculation of the radial (with re- (1) spect to a radar) component of the model wind V=+ d ex( − ut ) + fy-vt ( ), y b b fields, the Cartesian components of the model wind fields are first obtained and then the radial where a, d are constant flow components, b, e are component is extracted. Toward that end, the ve- shear parameters, c, f are divergence parameters, locity V of the MCRV can be expressed in vortex- ub, vb are the translational velocity components of centered cylindrical coordinates (not radar coordi- the broadscale fields, and t is time. It can be nates) as the sum of its radial and tangential com- noted that (1) implicitly makes provision for a ponents, V ˆ ˆ , where ˆ and ˆ are the broadscale vortex since the Cartesian representa- =vr r + v θθ r θ tion of a solid body vortex is u=−Ω y,v =Ω x, unit vectors in the radial and azimuthal directions in the vortex cylindrical coordinate system, respec- where Ω is the (constant) vortex angular velocity. tively. Figure 1 depicts the relationship between This broadscale vortex description is independent the Cartesian and vortex coordinate systems. The of the small-scale vortex model to be described Cartesian components of V are computed as: next. In a local cylindrical coordinate system cen- ˆ V tered on and translating with the modified com- u=⋅= i vcosr θ − vsinθ θ , ˆ V bined Rankine vortex, the azimuthal velocity field v=⋅= j vsinr θ + vcosθ θ . (4) vθ and radial velocity field vr are given by: Formulae for cosθ and sinθ at arbitrary time t fol- r r low immediately from Fig. 1: V,T r< R, V,R r< R, R R vθ = vr = (2) Rα Rβ x− x0 − utv V, r≥ R, V, r≥ R, cos θ = , α T β R r r r y− y − vt where sin θ = 0 v . r 2 2 r=( xxut −−0v )( +−− yy 0 vt v ) , (3) Substituting these into (4) yields is the distance of a given ( x, y) coordinate from the xx−− ut yy −− vt u=0v v − 0 v v , center of the vortex at time t. The vortex is de- rr r θ scribed by seven parameters: initial vortex center yy−−0 vtv xx −− 0 ut v location ( x0, y0), radius of maximum wind R, max- v= v + v . rr r θ imum tangential velocity VT, maximum radial ve- Substituting for vr, vθ from (2) and adding the linear locity VR, the radial decay rates α, β of the tangen- tial and radial wind components, and the transla- flow fields (1) produces the Cartesian representa- tion of the full model wind field: tional velocity components uv , v v. The model pa- rameters are listed in Table 1. __________________________________________________________________________________ VR V T ab(yvt)c(xut)+−+−+bb (x −−− x0 ut) v (y −− y 0 vt), v r < R, R R u = β α RV(xR−− x0 ut) v RV(y T −− y 0 vt) v a+−+−+ b(y vt)b c(x ut) b −, r ≥ R, rβ+1 r α + 1 VR V T v=+−+−+ d e(xut)bb f(yvt) (y −−+ y0 vt) v (xx −− 0 ut), v r < R, R R v = β α RV(yR−− y0 vt) v RV(x T −− x 0 ut) v =+−+−+d e(x ut)b f(y vt) b +, r ≥ R. rβ+1 r α + 1 mod Finally, solving for the radial component of the total velocity yields the model Doppler radar velocity, Vr : 2 Extended abstract AMS 89 th Annual Meeting 11-15 January 2009, Phoenix, AZ mod VR V T Vrnnbb= cosφ sin θ a +−+−+ b(y vt)c(xut) (x −−− x0 ut) v (y −−+ y 0 vt) v R R VR V T cosφnnbb cos θ d+−+−+ e(x ut) f(y vt) (y −−+ y0 vt) v (x −− x 0 ut) v R R r< R, (5) RV(xβ−− x ut) RV(y α −− y vt ) =cosφ sin θ a +−+−+ b(y vt) c(x ut) R0 v − T 0 v + nn b b β +1 α +1 r r RV(yβ−− y vt) RV(x α −− x ut) cosφ cos θ d+−+−+ e(x ut) f(y vt) R0 v + T 0 v nn b b β+1 α + 1 r r r≥ R. __________________________________________________________________________________ where θn and φn are the azimuth and elevation an- normalized, can be used to calculate the mean th gles, respectively, of the n radar ( θn is measured model error per radar grid point. clockwise from the north). The cost function J is minimized to retrieve the set of parameter values producing the least 3. COST FUNCTION COMPUTATION AND squares error in the model wind (best fit between MINIMIZATION model and observed winds). In view of (6) and the location of the model parameters in (5), our mini- The (squared) discrepancies between the ob- mization problem is highly non-linear. Conjugate served and model-predicted radial wind fields are gradient minimization methods have proven useful summed over the spatial-temporal domains of N for such problems. The minimization algorithm radars, each scanning in range rn, azimuth θ and used in this technique is the Polak-Ribiere (1969) elevation angle φ. By taking the translation of the method, a robust and efficient variant of the broadscale flow and vortex into account, discre- Fletcher and Reeves (1964) algorithm.
Details
-
File Typepdf
-
Upload Time-
-
Content LanguagesEnglish
-
Upload UserAnonymous/Not logged-in
-
File Pages15 Page
-
File Size-