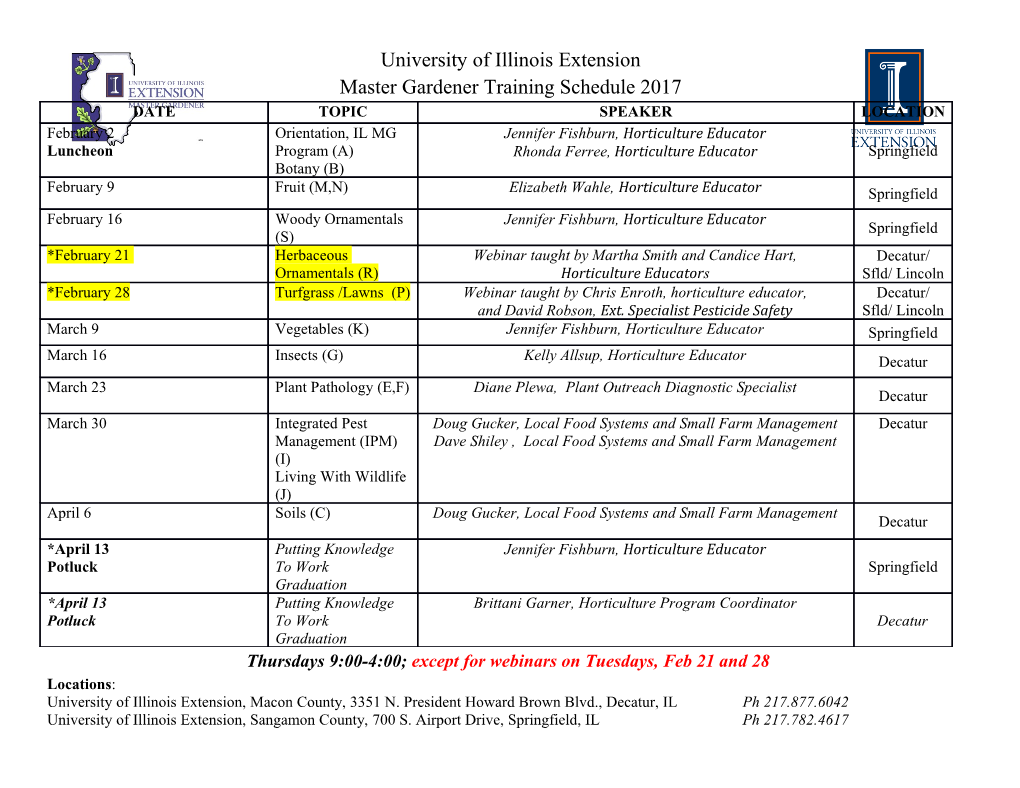
Combining molecular modelling with experiments: Sulfonylureas and glinides as new PPARγ agonists Marco Scarsi Biozentrum - Swiss Institute of Bioinformatics Drug discovery and development 12-15 years of work 5-6 years 5-6 years 1-2 years Registration Preclinical Candidate Clinical tests Drug and research drugs marketing Pre-clinical drug research: Real and virtual Optimization Target Molecular DB Candidate toxicology identification screening drugs metabolism Optimization Target Molecular DB Candidate toxicology identification screening drugs Optimizationmetabolism Target Molecular DB Candidate toxicology identification screening drugs 1-2 years 1 year 3-4metabolism years Virtual screening ? DB Does the molecule bind to the protein? Virtual screening • Principles of molecular mechanics: – Atoms as basic units – Chemical bonds cannot be broken: They can be stretched, bent, torqued. – Good to simulate non-covalent binding AutoDock • The potential generated by the protein is calculated on a grid • Each ligand is flexibly sampled on the grid (conformational search) AutoDock: algorithm • Lamarckian Genetic Algorithm • The searcher modifies the phenotype, which is allowed to update the genotype • Lamarck: “an adaptation of an individual to its environment can be inherited by its offsprings” Our work… Virtual Target screening of Experimental Drug selection: known drugs validation redirection PPARγ • Identify new PPARγ ligands among known drugs • TheraSTrat was interested in side-effects of known drugs Nuclear receptors Ligands: Nuclear receptor Response Element PPARγ • Pharmacological target for type-II diabetes (several drugs on the market) • It controls lipid metabolism and glucose homeostasis • Lots of experimental data available PPARγ agonists Fatty acid (endogenous) Thiazolidinedione Tyrosine-based (drug) agonist (candidate drug) X-ray: PPARγ bound to farglitazar HIS 323 TYR 473 SER 289 HIS 449 Farglitazar: potent synthetic agonist (nM) Mutations is SER289, HIS323, TYR473, HIS449 strongly reduce activity Agonists aligned (X-ray) • Is there anything else binding to PPARγ ? • Virtual screening Compound libraries • TheraSTrat AG database: ~8000 compounds – most marketed drugs – proprietary • Chembank database: ~6000 compounds – bioactive compounds – freely available Virtual screening on a grid Docking a ligand to the receptor CSCS [BC]2 PC Desktop Grid (PBS) (UD MP, Win32) Grid Manager Ticino Basel [BC]2 HPC cluster Vital-IT HPC cluster (SGE, x86-32) (LSF, Itanium 64) Basel Lausanne Docking results • Sulfonylureas and glinides bind to PPARγ Gliquidone Repaglinide Farglitazar (x-ray) Glipizide Nateglinide Glimepiride Mitiglinide • Why are sulfonylureas and glinides so interesting as putative PPARγ agonists? Type II Diabetes: drug therapies PPARγ Sulfonylureas, agonists Glinides ? Bind to sulfonylurea Bind to PPARγ receptor Reduce insulin Improve insulin resistance secretion Type II diabetes treatment Experimental validation 3 experiments: biochemistry • Binding to receptor – displacement of labeled ligand • Activation of receptor – transactivation assays • Activation of metabolic cell biology pathways – expression of PPARγ-regulated genes PPARγ Binding Assays • PPARγ + fluorescent labeled high-affinity ligand • Competitor assay Results of Binding Assays Sulfonylureas Glinides Gliquidone IC50 = 8μM Nateglinide IC50 = 316μM 120 120 100 100 80 80 60 60 40 40 Ar(%) 20 Ar(%) 20 0 0 -20 -20 0.001 0.1 10 1000 0.001 0.1 10 1000 Compound (µM) Compound (µM) ...and ...and Glimepiride IC50 = 125μM Repaglinide IC50 > 1.5mM IC50 ? Glipizide IC50 ? Mitiglinide Activation of receptor • Transactivation Ligand assay Expression PPAR Vector • Measure ligand effect on synthetic PPAR RXR PPARE target gene light emitted by luciferin/luciferase LUC reaction measured by photometer Reporter vector Results of transactivation assays Sulfonylureas Glinides 16 14 4 12 3 10 8 2 6 Fold activation Fold activation 4 Gliquidone 1 Repaglinide 2 Glipizide Nateglinide Mitiglinide 0 Glimepiride 0 0.001 0.01 0.1 1 10 100 1000 0.001 0.01 0.1 1 10 100 1000 Concentration (µM)m Concentration (µM) ÎSulfonylureas and glinides activate PPARγ in the 10-100 mM range PPARγ-dependent gene activation • Mouse pre-adipocyte cells • Measure expression of selected genes induced by PPARγ signaling: – Adiponectin –aP2 –GLUT4 PPARγ-dependent gene activation 100 90 80 70 adiponectin 60 50 aP2 40 GLUT4 30 20 10 0 % of induction rosiglitazone induction % of glipizide 100 nateglinide 50 gliquidone 10 pioglitazone 10 microM microM microM microM Sulfonylureas and Glinides Known PPARγ activator Experiments: Summary biochemistry • Sulfonylureas and glinides: – bind to PPARγ – activate PPARγ and cell biology enhance transcription Clinically relevant? •PPARγ activation observed at concentrations of 10-100 μM • Do these drugs ever reach these plasma concentrations? • Yes (gliquidone, glipizide, nateglinide) Type II Diabetes: drug therapies Glitazones, Sulfonylureas, TZDs Glinides Bind to sulfonylurea Activate PPARγ receptor Reduce insulin Improve insulin resistance secretion type II diabetes treatment Conclusion: Chemical – carboxylic acids known PPARγ agonists – thiazolidinediones – sulfonylureas new • Same acidity: – carboxylic acids pKa ~4.8 – thiazolidinediones pKa ~6.5 – sulfonylureas pKa ~5.3 • Same network of H-bonds Conclusions: Pharmacological • Sulfonylurea and glinide drugs can: – Enhance insulin secretion (SU receptor) – Reduce insulin resistance (PPARγ) • Possible to design new drugs targeting PPARγ and the SU receptor • A "favorable side effect"? A broad sinergy Michael Podvinec Adrian Roth Renate Looser Urs A. Meyer Torsten Schwede Christoph Ruecker TheraSTrat Hubert Hug Hugo Albrecht Sander Kersten Patent? Hard to patent: Patentable: Disease 1 Disease 1 Disease 2 Pre-clinical research: real and virtual High- Target Lead Candidate throughput Leads Toxicology, identification optimization drugs screening metabolism High- Target Lead Candidate throughput Leads Toxicology, identification optimization drugs screening metabolism High- Target Lead Candidate throughput Leads Toxicology, 1-2identification years 1 year 2-3optimization years 1-2 years drugs screening metabolism.
Details
-
File Typepdf
-
Upload Time-
-
Content LanguagesEnglish
-
Upload UserAnonymous/Not logged-in
-
File Pages36 Page
-
File Size-