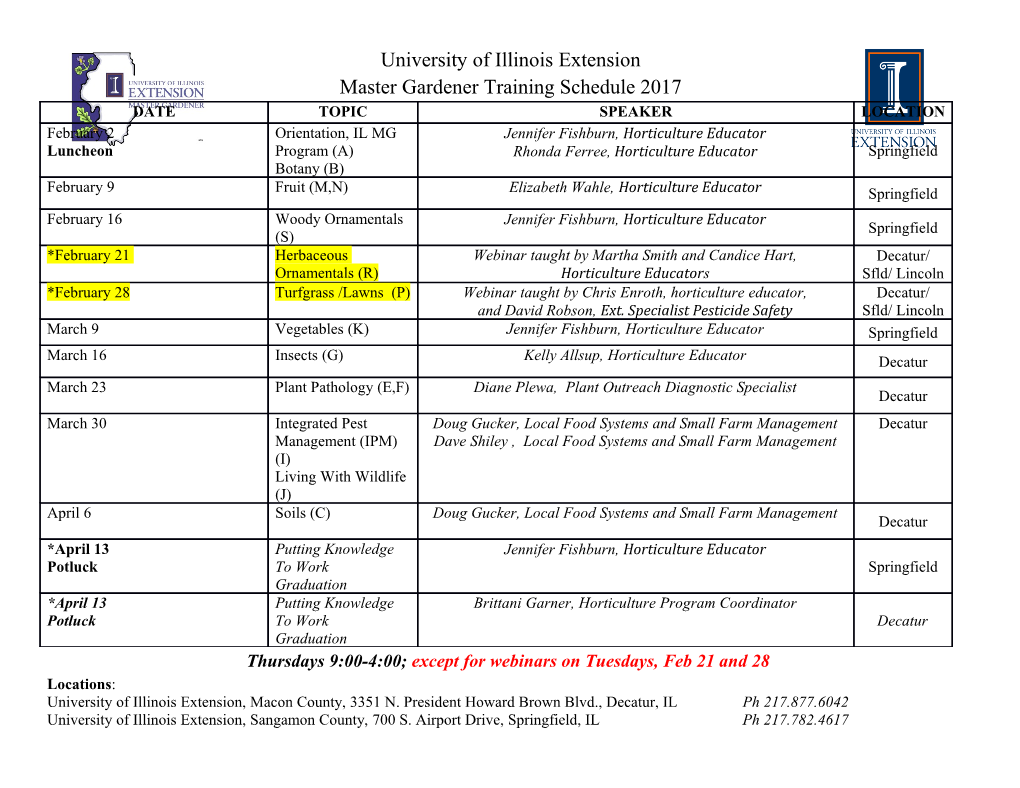
Bioe 144/244 Introduction to ProteinMultiple Informatics sequence alignment (MSA) algorithms, uses and benchmarking Subset (to avoid Bioe 190 redundancy with GFKLP previous lectures GYKLP Fall 2016 and Pevsner text) GFRVP GF-LP 1 Bioe 144/244 Introduction to Protein Informatics Topics Covered • Uses of multiple sequence alignments (MSAs) • Global, glocal and local alignments • Structural vs sequence alignments • Progressive, iterative, consistency-based and master-slave alignment methods • MAFFT and SATCHMO-JS • Benchmark datasets & scoring functions • MSA formats (aligned FASTA, UCSC a2m) 2 Bioe 144/244 What is an MSA? Introduction to Protein Informatics • An MSA is an assertion of homology – > 2 nucleotide or amino acid sequences – Characters in the columns have descended from an ancestral character except for indel characters that represent insertions and deletions • An MSA is a matrix – Mi,j = the character for sequence i at column j. – Lower-case characters may have different meanings from upper- case characters – Dot (.) and dash (-) are indel characters (understand UCSC a2m format) 3 Bioe 144/244 Uses of sequence alignment Introduction to Protein Informatics Phylogenetic tree Active and binding GFKLP site prediction GYKLP Homology Models GFRVP GF-LP Profiles/HMM construction And more… Domain Prediction Substitution Matrices Function prediction by phylogenomic analysis Secondary structure prediction Subfamily identification 4 … Bioe 144/244The relationship of trees and MSAs Introduction to Protein Informatics • An MSA is typically used as input to estimate a phylogenetic tree • Some progressive MSA algorithms start by estimating a hierarchical tree based on pairwise similarity (or distance) scores, from which a guide tree can be constructed; the guide tree is then used to determine the order in which sequences (or groups of sequences are aligned to each other. • Simulataneous sequence alignment and phylogenetic tree estimation methods also exist (e.g., SATCHMO); – Note that although SATCHMO is a progressive alignment method it does not use a guide tree 5 Major techniques used in MSA Bioe 144/244 Introduction to Protein Informatics estimation • Optimizing a scoring function (e.g., sum of pairs) – note sensitivity to the substitution/match/indel scores – ClustalW set indel costs higher in hydrophobic regions • Dynamic Programming (to align sequences/groups to each other) • Divide-and-conquer techniques – Tree-based partitioning (MUSCLE, MAFFT) – Similar idea used in SATCHMO-JS • Guide trees (in some progressive methods) – Estimated initially without an MSA (based on pairwise sequence comparison scores) – Subsequent iterations use the current MSA as the basis for estimating a tree (for tree-dependent partitioning) 6 Bioe 144/244 Introduction to Protein Informatics Some alignments are easy Note: Belvu MSA coloring (based on BLOSUM62 average pairwise score Light blue: highest conservation, Dark blue: moderate conservation. Grey: few residues conserved Uncolored: mixed distributions 7 SATCHMO simultaneous MSA & Tree Bioe 144/244 Introduction to Protein Informatics estimation 8 Bioe 144/244 Introduction to Protein InformaticsLocal, glocal and global-global • Local-local Best for boosting remote homolog detection, identification of evolutionary domains. Default protocol of BLAST and PSI-BLAST. • Global-local (aka glocal) Global to the query, potentially local to the hit. Best for gathering homologs to a structural or functional domain. • Global-global Restrict sequences to those appearing to have the same domain architecture. Default protocol of FlowerPower. 9 As proteins diverge from their common Bioe 144/244 Introduction to Proteinancestor, Informatics structurally superposable positions decrease Restricted to the “common core” “The relation between the divergence of sequence and structure in proteins”, Chothia and Lesk. EMBO Journal 1986 Structural alignment is the gold standard Bioe 144/244 Introduction to Protein forInformatics evaluating sequence alignment • Structural superposition of two PDB structures provides correspondences/equivalences between residues • Since primary sequence diverges more rapidly than 3D structure, structural alignment is the gold standard against which sequence alignment is assessed • Not all structural aligners agree on all pairs • However, clearly superposable pairs (within 2.5 Angstroms) are normally agreed upon by structural aligners • Example structural aligners include: JFAT-CAT, CE, DALI, VAST, Structal 11 RCSB/PDB Structure superposition: Bioe 144/244 Introduction to Proteinhelices Informatics and strands superpose better than loops 12 Bioe 144/244 Introduction to Protein Informatics VAST multiple alignment based on structure superposition: Non-equivalent positions are in lower-case 1SN4 Scorpion neurotoxin (blue positions are not superposable) 13 Bioe 144/244 Basic MSA method classes Introduction to Protein Informatics • Progressive: – Key feature: once two sequences are aligned to each other, their respective alignment will not change (errors cannot be fixed) – Note: Sometimes (not always) a guide tree (based on pairwise sequence similarity) is used to determine the join order of the sequences (e.g., ClustalW) – Pros: output alignments generally good within clusters of closely related sequences – Cons: cannot fix errors – Examples: ClustalW, SATCHMO • Iterative – Key feature: alignments of sequences may be adjusted based on some objective function – Pros: improves accuracy; Cons: additional computational complexity over progressive alignment – Note that many iterative alignment algorithms include progressive alignment – Examples: MUSCLE, MAFFT • Master-slave – One sequence (or profile/HMM) is the master; other sequences are aligned to it – Pros: fast. Cons: low accuracy (especially in regions where the profile/HMM is noisy, or sequences being aligned diverge in sequence) – Examples: BLAST, PSI-BLAST, aligning sequences to an HMM • Consistency-based – Key feature: the algorithm attempts to enforce that if in the set of pairwise alignments to be integrated into the MSA, residue i is aligned to residue j, and residue j is aligned to residue k, that in the output MSA, residues i, j and k all align to each other. – Pros: makes biological sense. Cons: computationally expensive (slow, problem on large inputs) – Examples: ProbCons, T-Coffee MAFFT Iterative Alignment Bioe 144/244 Introduction to Protein(includes Informatics an initial progressive step) MAFFT: a novel method for rapid multiple sequence alignment based on fast Fourier transform, Katoh, Misawa, Kuma, and Miyataa, Nucleic Acids Res. 2002 Jul 15;30(14):3059-66. The basic steps of the MAFFT algorithm 1. A guide tree is estimated based on pairwise sequence comparison distance matrix 2. Input sequences are progressively aligned following the branching order of sequences in the guide tree. 3. The alignment is subjected to further improvement, in which the alignment is divided into two groups and realigned using a technique called tree-dependent restricted partitioning. 4. This process is iterated until no better scoring alignment is obtained http://nar.oxfordjournals.org/content/30/14/3059.full 15 Tree-dependant partitioning used in the Bioe 144/244 Introduction to Protein Informatics MAFFT iterative step 16 http://nar.oxfordjournals.org/content/30/14/3059.full Bioe 144/244 Introduction to Protein Informatics Benchmark datasets • BAliBase (Benchmark Alignment Database) • One of the oldest benchmark datasets. Numerous small test sets evaluating different types of data (high vs low %ID, uneven or similar lengths, etc.). Limitations: many sequences have no known structure (reference alignments manually refined); most reference/ test datasets are small in size (do not evaluate how methods perform on large inputs). • PREFAB (Protein Reference Alignment database) • Pairwise structural alignments at different levels of divergence • SABmark (Sequence and structure Alignment Benchmark) • Recommended reading: “SABmark—a benchmark for sequence alignment that covers the entire known fold space”, Ivo Van Walle,, Ignace Laster and Lode Wyns, Bioinformatics (2005) 21 (7): 1267-1268. 17 SATCHMO: Simultaneous Alignment and Tree Construction using Hidden Markov mOdels Edgar, R., and Sjölander, K., Bioinformatics 2003 Hagopian, R., Davidson, J., Datta, R., Samad, B., Jarvis, G., and Sjölander, K. "SATCHMO-JS: a webserver for simultaneous protein multiple sequence alignment and phylogenetic tree construction," Nucleic Acids Research 2010 Related work • “A Probabilistic Treatment of Phylogeny and Sequence Alignment”, G.J. Mitchison, Journal of Molecular Evolution, 1998, Volume 49, Number 1, 11-22. • “POY version 4: phylogenetic analysis using dynamic homologies”, Andrés Varón, Le Sy Vinh, Ward C. Wheeler, Cladistics, Volume 26, Issue 1, pages 72– 85, February 2010 SATCHMO algorithm • Input: unaligned sequences. • Initialize: a profile HMM is constructed for each sequence using Dirichlet mixture densities; each sequence forms a separate subtree (of a single sequence each) – Dirichlet mixture densities avoid the problems of small counts • While (#subtrees > 1) { – Use profile-profile scoring to select closest pair to join – Align pair to each other, keeping columns fixed within each subtree – Mask columns with many gaps or low scores (use a window). – Construct a profile HMM for the new masked MSA } • Output: Tree and MSA Benchmarking SATCHMO accuracy Evaluating the phylogenetic tree accuracy is difficult ● Simulation studies are used to evaluate evolutionary
Details
-
File Typepdf
-
Upload Time-
-
Content LanguagesEnglish
-
Upload UserAnonymous/Not logged-in
-
File Pages42 Page
-
File Size-