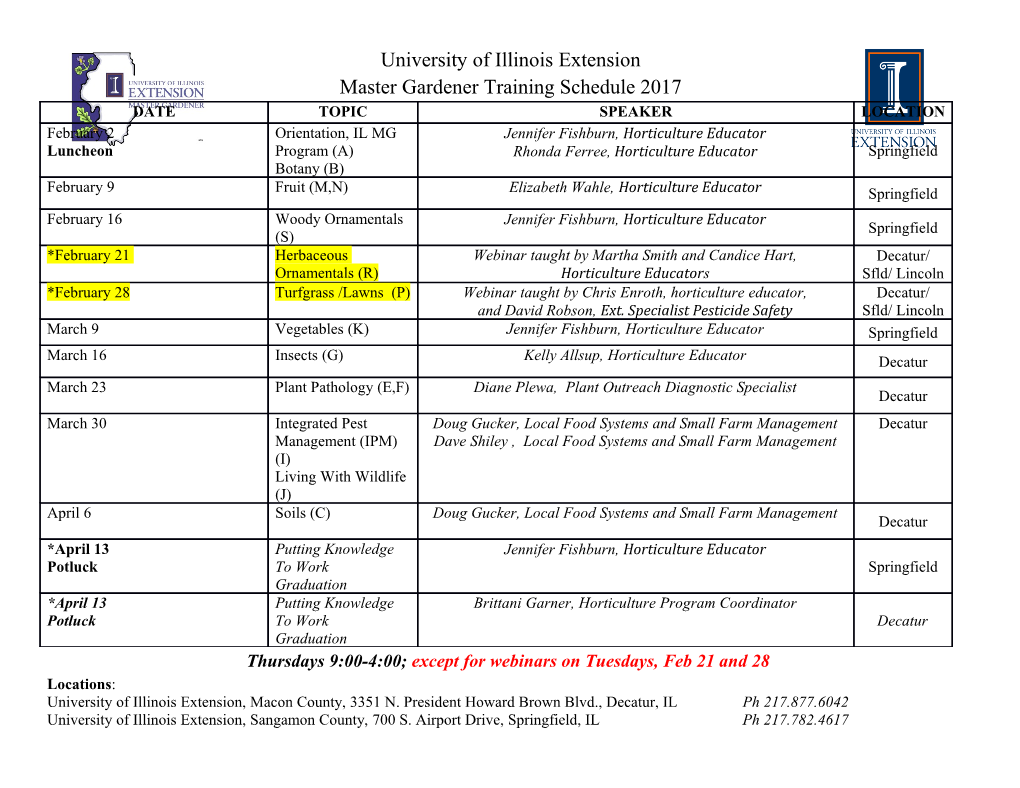
ARTIFICIAL INTELLIGENCE POLICY FORUM Even when not category-jumping, machine learning can be used to draw powerful and compromising in- ferences from self-disclosed, seemingly benign data or readily observed behavior. These inferences can un- Data, privacy, and the greater good dermine a basic goal of many privacy laws—to allow Eric Horvitz1* and Deirdre Mulligan2* individuals to control who knows what about them. Machine learning and inference makes it increasingly Large-scale aggregate analyses of anonymized data can yield valuable results and insights that difficult for individuals to understand what others can address public health challenges and provide new avenues for scientific discovery. These methods know about them based on what they have explicitly can extend our knowledge and provide new tools for enhancing health and wellbeing. However, they or implicitly shared. And these computer-generated raise questions about how to best address potential threats to privacy while reaping benefits for channels of information about health conditions individuals and to society as a whole.The use of machine learning to make leaps across informational join other technically created fissures in existing legal and social contexts to infer health conditions and risks from nonmedical data provides protections for health privacy. In particular, it is dif- representative scenarios for reflections on directions with balancing innovation and regulation. ficult to reliably deidentify publicly shared data sets, given the enormous amount and variety of ancillary hat if analyzing Twitter tweets or Face- Although digital nudging shows promise, a re- data that can be used to reidentify individuals. book posts could identify new mothers cent flare-up in the United Kingdom highlights The capacities of machine learning expose the fun- at risk for postpartum depression (PPD)? the privacy concerns it can ignite. A Twitter suicide- damental limitations of existing U.S. privacy rules that Despite PPD’s serious consequences, early prevention application called Good Samaritan tie the privacy protection of an individual’shealthsta- W ’ identification and prevention remain monitored individuals tweets for words and tustospecificcontextsorspecifictypesofinforma- difficult. Absent a history of depression, detection phrases indicating a potential mental health crisis. tion a priori identified as health information. Health Downloaded from is largely dependent on new mothers’ self-reports. The app notified the person’s followers so they privacy regulations and privacy laws in the United But researchers found that shifts in sets of activ- States generally are based on the assumption ities and language usage on Facebook are pre- that the semantics of data are relatively fixed dictors of PPD (1) (see the photo). This is but one and knowable and reside in isolated contexts. example of promising research that uses machine Machine learning techniques can instead be used learning to derive and leverage health-related in- to infer new meaning within and across contexts ferences from the massive flows of data about in- and is generally unencumbered by privacy rules http://science.sciencemag.org/ dividuals and populations generated through social in the United States. Using publicly available media and other digital data streams. At the same Twitter posts to infer risk of PPD, for example, time, machine learning presents new challenges does not run afoul of existing privacy law. This for protecting individual privacy and ensuring fair might be unsurprising, and seem unproblematic, use of data. We need to strike a new balance be- given that the posts were publicly shared, but tween controls on collecting information and con- there are troubling consequences. trols on how it is used, as well as pursue auditable Current privacy laws often do double duty. At a and accountable technologies and systems that basic level, they limit who has access to infor- facilitate greater use-based privacy protections. mation about a person. This implicitly limits the Researchers have coined terms, such as digi- extent to which that information influences Machine learning can make “category-jumping” infer- on October 16, 2017 tal disease detection (2)andinfodemiology(3), ences about health. New mother’s activities and language decision-making and thus doubles as a limit on to define the new science of harnessing diverse usage on social media are predictors of postpartum the opportunities for information to fuel dis- streams of digital information to inform public depression. crimination. Because of the heightened privacy health and policy, e.g., earlier identification of epi- sensitivities and concerns with health-related dis- demics, (4) modeling communicability and flow of could intervene to avert a potential suicide. But crimination, we have additional laws that regulate illness (5), and stratifying individuals at risk for the app was shuttered after public outcry drew reg- the use of health information outside the health care illness (6). This new form of health research can ulator concern (8). Critics worried the app would context. U.S. laws specifically limit the use of some also inform and extend understandings drawn from encourageonlinestalkers and bullies to target vul- health information in ways considered unfair. For traditional health records and human subjects re- nerable individuals and collected 1200 signatures example, credit-reporting agencies are generally pro- search.Forexample,thedetectionofadversedrug on a petition arguing that the app breached users’ hibited from providing medical information to make reactions could be improved by jointly leveraging privacy by collecting, processing, and sharing sen- decisions about employment, credit, or housing. The data from the U.S. Food and Drug Administration’s sitive information. Despite the developers’ laudable Americans with Disabilities Act (ADA) prohibits dis- Adverse Event Reporting System and anonymized goal of preventing suicide, the nonprofit was chas- crimination on the basis of substantial physical or search logs (7). Search logs can serve as a large- tised for playing fast and loose with the privacy and mental disabilities—or even a mistaken belief that an scale sensing system that can be used for drug mental health of those it was seeking to save (9). individual suffers from such a disability. If machine safety surveillance—pharmacovigilance. Machine learning can facilitate leaps across infor- learning is used to infer that an individual suffers Infodemiology studies are typically large-scale mational and social contexts, making “category- from a physical or mental impairment, an employer aggregate analyses of anonymized data—publicly jumping” inferences about health conditions or who bases a hiring decision on it, even if the in- disclosed or privately held—that yield results and propensities from nonmedical data generated far ference is wrong, would violate the law. insights on public health questions across popula- outside the medical context. The implications for But the ADA does not prohibit discrimination based tions. However, some methods and models can be privacy are profound. Category-jumping inferences on predispositions for such disabilities (10). Machine aimed at making inferences about unique individ- may reveal attributes or conditions an individual has learning might discover those, too. In theory, the uals that could drive actions, such as alerting or specifically withheld from others. To protect against Genetic Information Non-Discrimination Act (GINA) providing digital nudges, to improve individual such violations, the United States heavily regulates should fill this gap by protecting people genetically or public health outcomes. health care privacy. But, although information about predisposed to a disease. But again, machine learn- health conditions garnered from health care treat- ing exposes cracks in this protection. Although 1 2 Microsoft Research, Redmond, WA 98052, USA. University ment and payment must be handled in a manner GINA prohibits discrimination based on informa- of California, Berkeley, Berkeley, CA 94720, USA. *Corresponding author. E-mail: [email protected] (E.H.); that respects patient privacy, machine learning and in- tion derived from genetic tests or a family history of CREDIT: ISTOCK/CHRISTINE GLADE [email protected] (D.M.) ference can sidestep many of the existing protections. adisease(11), it does not limit the use of information SCIENCE sciencemag.org 17 JULY 2015 • VOL 349 ISSUE 6245 253 ARTIFICIAL INTELLIGENCE about such a disposition—even if it is grounded in information and resell or share that information tional law. What exactly individuals receive when genetics—inferred through machine learning tech- with others—toclearlydisclosetoconsumersin- they request access to their data and to processing niques that mine other sorts of data. In other words, formation about the data they collect, as well as logicvariesbycountry,asdoestheimplementation machine learning that predicts future health status thefactthattheyderiveinferencesfromit(21). of the limitation on “purely automated” processing. from nongenetic information—including health status Here,too,theFTCappearsconcernedwithnotjust The EU is expected to adopt a data privacy regula- changes due to genetic predisposition—would cir- the raw data, but inferences from its analysis. tion that will supplant local law, with a single na- cumvent existing legal protections (12). The Obama Administration’s Big Data
Details
-
File Typepdf
-
Upload Time-
-
Content LanguagesEnglish
-
Upload UserAnonymous/Not logged-in
-
File Pages4 Page
-
File Size-