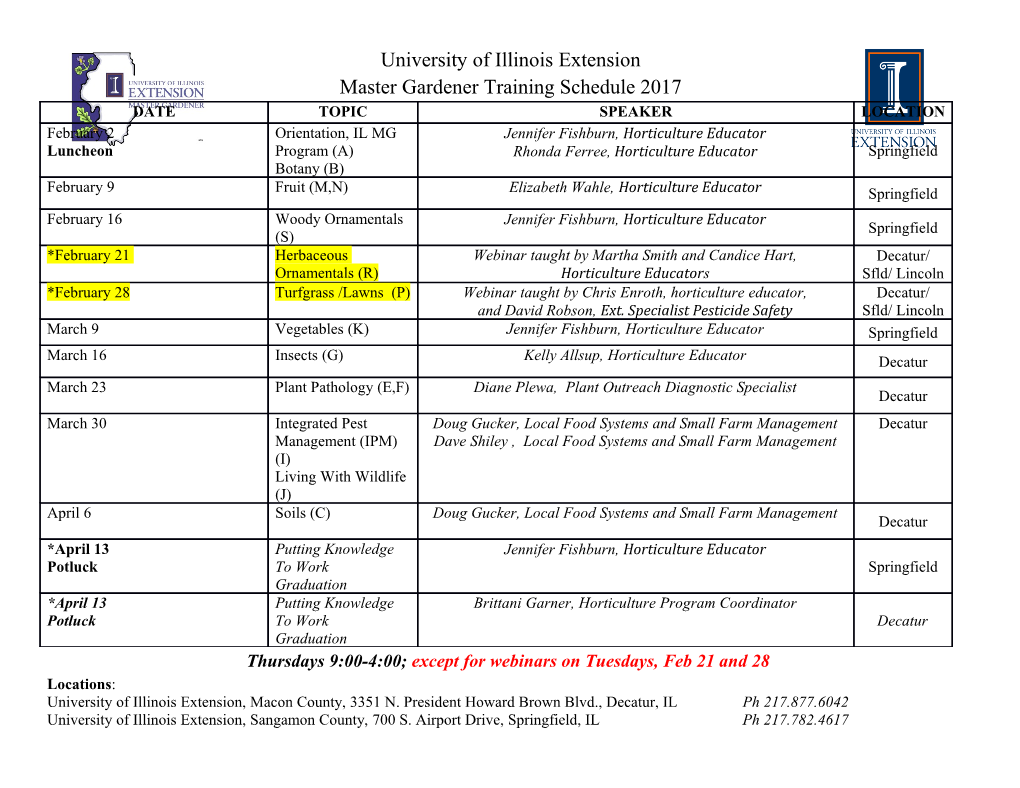
Universally Typical Sets for Ergodic Sources of Multidimensional Data Tyll Kr¨uger,Guido Montufar, Ruedi Seiler and Rainer Siegmund-Schultze http://arxiv.org/abs/1105.0393 • lossless: algorithm ensurs exact reconstruction • main idea (Shannon): encode typical but small set • universal: algorithm does not involve specific properties of random process. • main idea: construction of universally typical sets. Universal Lossless Encoding Algorithms • data modeled by stationary/ergodic random process • main idea (Shannon): encode typical but small set • universal: algorithm does not involve specific properties of random process. • main idea: construction of universally typical sets. Universal Lossless Encoding Algorithms • data modeled by stationary/ergodic random process • lossless: algorithm ensurs exact reconstruction • universal: algorithm does not involve specific properties of random process. • main idea: construction of universally typical sets. Universal Lossless Encoding Algorithms • data modeled by stationary/ergodic random process • lossless: algorithm ensurs exact reconstruction • main idea (Shannon): encode typical but small set • main idea: construction of universally typical sets. Universal Lossless Encoding Algorithms • data modeled by stationary/ergodic random process • lossless: algorithm ensurs exact reconstruction • main idea (Shannon): encode typical but small set • universal: algorithm does not involve specific properties of random process. Universal Lossless Encoding Algorithms • data modeled by stationary/ergodic random process • lossless: algorithm ensurs exact reconstruction • main idea (Shannon): encode typical but small set • universal: algorithm does not involve specific properties of random process. • main idea: construction of universally typical sets. • small size enh(µ) but still • nearly full measure • output sequences with higher or smaler probability than e−nh(µ) will rarely be observed. Entropy Typical Set 1 (xn) with − log µ(xn) ∼ h(µ) 1 n 1 . have the Asymptotic Equipartition Property: n −nh(µ) • all (x1 ) have the same probability e • nearly full measure • output sequences with higher or smaler probability than e−nh(µ) will rarely be observed. Entropy Typical Set 1 (xn) with − log µ(xn) ∼ h(µ) 1 n 1 . have the Asymptotic Equipartition Property: n −nh(µ) • all (x1 ) have the same probability e • small size enh(µ) but still • output sequences with higher or smaler probability than e−nh(µ) will rarely be observed. Entropy Typical Set 1 (xn) with − log µ(xn) ∼ h(µ) 1 n 1 . have the Asymptotic Equipartition Property: n −nh(µ) • all (x1 ) have the same probability e • small size enh(µ) but still • nearly full measure Entropy Typical Set 1 (xn) with − log µ(xn) ∼ h(µ) 1 n 1 . have the Asymptotic Equipartition Property: n −nh(µ) • all (x1 ) have the same probability e • small size enh(µ) but still • nearly full measure • output sequences with higher or smaler probability than e−nh(µ) will rarely be observed. • pointwise almost surely (Mcmillan, Briman) • amenable groups, Zd (Kiefer, Ornstein and Weiss) Shannon-Mcmillan-Briman Z -ergodic processes: 1 n • − n log µ(x1 ) ! h(µ): • in probability (Shannon) • amenable groups, Zd (Kiefer, Ornstein and Weiss) Shannon-Mcmillan-Briman Z -ergodic processes: 1 n • − n log µ(x1 ) ! h(µ): • in probability (Shannon) • pointwise almost surely (Mcmillan, Briman) Shannon-Mcmillan-Briman Z -ergodic processes: 1 n • − n log µ(x1 ) ! h(µ): • in probability (Shannon) • pointwise almost surely (Mcmillan, Briman) • amenable groups, Zd (Kiefer, Ornstein and Weiss) d • Σn := AΛn ; Σ = AZ , A finite alphabet Notation d-dimensional: d • Λn := (i1; : : : ; id) 2 Z+ : 0 ≤ ij ≤ n − 1; j 2 f1; : : : ; dg Notation d-dimensional: d • Λn := (i1; : : : ; id) 2 Z+ : 0 ≤ ij ≤ n − 1; j 2 f1; : : : ; dg d • Σn := AΛn ; Σ = AZ , A finite alphabet log jTn(h0)j 2. lim = h0. n!1 nd 3. optimal Result Theorem (Universally-typical-sets) For any given h0 with 0 < h0 ≤ log(jAj) one can construct a n sequence of subsets fTn(h0) ⊂ Σ gn such that for all µ 2 Perg with h(µ) < h0 the following holds: 1. lim µn (Tn(h0)) = 1, n!1 3. optimal Result Theorem (Universally-typical-sets) For any given h0 with 0 < h0 ≤ log(jAj) one can construct a n sequence of subsets fTn(h0) ⊂ Σ gn such that for all µ 2 Perg with h(µ) < h0 the following holds: 1. lim µn (Tn(h0)) = 1, n!1 log jTn(h0)j 2. lim = h0. n!1 nd Result Theorem (Universally-typical-sets) For any given h0 with 0 < h0 ≤ log(jAj) one can construct a n sequence of subsets fTn(h0) ⊂ Σ gn such that for all µ 2 Perg with h(µ) < h0 the following holds: 1. lim µn (Tn(h0)) = 1, n!1 log jTn(h0)j 2. lim = h0. n!1 nd 3. optimal • 1 k;n Tn(h0) := Πnfx 2 Σ: kd H(~µx ) ≤ h0g d 1 d • k ≤ 1+ logjAj n ; > 0 • log jTn(h0)j lim sup nd ≤ h0 Remarks about Proof: n k;no Λ • for each x 2 Σ empirical measures µ~x on A k . k≤n n k;no Explain empirical measure µ~x by a drawing (black bord) d 1 d • k ≤ 1+ logjAj n ; > 0 • log jTn(h0)j lim sup nd ≤ h0 Remarks about Proof: n k;no Λ • for each x 2 Σ empirical measures µ~x on A k . k≤n • 1 k;n Tn(h0) := Πnfx 2 Σ: kd H(~µx ) ≤ h0g n k;no Explain empirical measure µ~x by a drawing (black bord) • log jTn(h0)j lim sup nd ≤ h0 Remarks about Proof: n k;no Λ • for each x 2 Σ empirical measures µ~x on A k . k≤n • 1 k;n Tn(h0) := Πnfx 2 Σ: kd H(~µx ) ≤ h0g d 1 d • k ≤ 1+ logjAj n ; > 0 n k;no Explain empirical measure µ~x by a drawing (black bord) Remarks about Proof: n k;no Λ • for each x 2 Σ empirical measures µ~x on A k . k≤n • 1 k;n Tn(h0) := Πnfx 2 Σ: kd H(~µx ) ≤ h0g d 1 d • k ≤ 1+ logjAj n ; > 0 • log jTn(h0)j lim sup nd ≤ h0 n k;no Explain empirical measure µ~x by a drawing (black bord) Theorem (Empirical-Entropy Theorem) n!1 Let µ 2 Perg. For any sequence fkng satisfying kn −−−!1 and d d kn = o(n ) we have 1 kn;n lim d H(~µx ) = h(µ) ; µ-almost surely : n!1 kn Main references • Paul C. Shields: The Ergodic Theory of Discrete Sample Paths, Graduate Studies in Mathematics, Vol.13 AMS. • Article to appear in KYBERNETICA Background Material Lemma (Packing Lemma) Consider for any fixed 0 < δ ≤ 1 integers k and m related through k ≥ d · m/δ. Let C ⊂ Σm and x 2 Σ with the property m;k that µ~x;overl(C) ≥ 1 − δ. Then, there exists p 2 Λm such that: p;m;k a) µ~x (C) ≥ 1 − 2δ, and also b) k d p;m;k k d m µ~x (C) ≥ (1 − 4)δ( m + 2) . Theorem 1 Given any µ 2 Perg and any α 2 (0; 2 ) we have the following: • For all k larger than some k0 = k0(α) there is a set k Tk(α) ⊂ Σ satisfying log jT (α)j k ≤ h(µ) + α ; kd and such that for µ-a.e. x the following holds: k;n µ~x (Tk(α)) > 1 − α ; k for all n and k such that n < " for some " = "(α) > 0 and n larger than some n0(x). • (optimality) Definition (Entropy-typical-sets) 1 Let δ < 2 . For some µ with entropy rate h(µ) the entropy-typical-sets are defined as: n m −md(h(µ)+δ) m −md(h(µ)−δ)o Cm(δ) := x 2 Σ : 2 ≤ µ (fxg) ≤ 2 : (1) We will use these sets as basic sets for the typical-sampling-sets defined below. Definition (Typical-sampling-sets) 1 Consider some µ. Consider some δ < 2 . For k ≥ m, we define a k typical-sampling-set Tk(δ; m) as the set of elements in Σ that have a regular m-block partition such that the resulting words belonging to the µ-entropy-typical-set Cm = Cm(δ) contribute at least a (1 − δ)-fraction to the (slightly modified) number of partition elements in that regular m-block partition, more precisely, they occupy at least a (1 − δ)-fraction of all sites in Λk d n X k o T (δ; m) := x 2 Σk : 1 (σ x) ≥ (1−δ) for some p 2 Λ : k [Cm] r+p m m d r2m·Z : (Λm+r+p)\Λk6=;.
Details
-
File Typepdf
-
Upload Time-
-
Content LanguagesEnglish
-
Upload UserAnonymous/Not logged-in
-
File Pages28 Page
-
File Size-