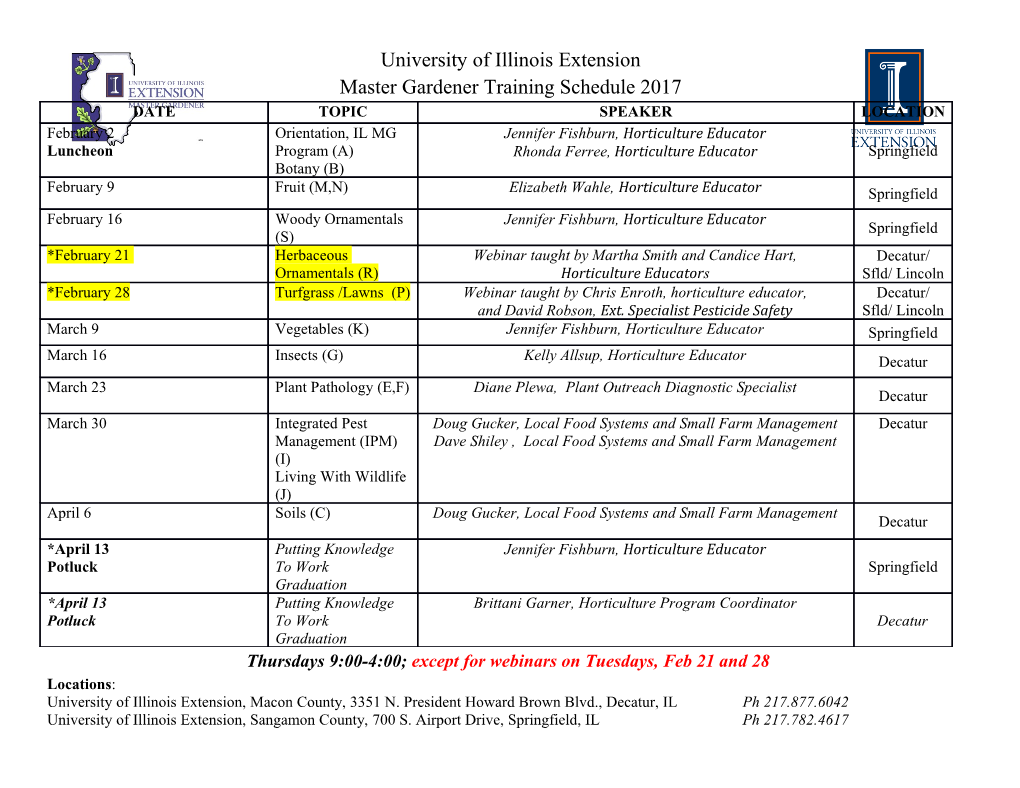
Joint mPlane / BigFoot PhD School: DAY 1 Hadoop MapReduce: Theory and Practice Pietro Michiardi Eurecom bigfootproject.eu ict-mplane.eu Pietro Michiardi (Eurecom) Joint mPlane / BigFoot PhD School: DAY 1 1 / 86 Sources and Acks Jimmy Lin and Chris Dyer, “Data-Intensive Text Processing with MapReduce,” Morgan & Claypool Publishers, 2010. http://lintool.github.io/MapReduceAlgorithms/ Tom White, “Hadoop, The Definitive Guide,” O’Reilly / Yahoo Press, 2012 Anand Rajaraman, Jeffrey D. Ullman, Jure Leskovec, “Mining of Massive Datasets”, Cambridge University Press, 2013 Pietro Michiardi (Eurecom) Joint mPlane / BigFoot PhD School: DAY 1 2 / 86 Introduction and Motivations Introduction and Motivations Pietro Michiardi (Eurecom) Joint mPlane / BigFoot PhD School: DAY 1 3 / 86 Introduction and Motivations What is MapReduce? A programming model: I Inspired by functional programming I Parallel computations on massive amounts of data An execution framework: I Designed for large-scale data processing I Designed to run on clusters of commodity hardware Pietro Michiardi (Eurecom) Joint mPlane / BigFoot PhD School: DAY 1 4 / 86 Introduction and Motivations What is this PhD School About DAY1: The MapReduce Programming Model I Principles of functional programming I Scalable algorithm design DAY2: In-depth description of Hadoop MapReduce I Architecture internals I Software components I Cluster deployments DAY3: Relational Algebra and High-Level Languages I Basic operators and their equivalence in MapReduce I Hadoop Pig and PigLatin Pietro Michiardi (Eurecom) Joint mPlane / BigFoot PhD School: DAY 1 5 / 86 Introduction and Motivations What is Big Data? Vast repositories of data I The Web I Physics I Astronomy I Finance Volume, Velocity, Variety It’s not the algorithm, it’s the data! [1] I More data leads to better accuracy I With more data, accuracy of different algorithms converges Pietro Michiardi (Eurecom) Joint mPlane / BigFoot PhD School: DAY 1 6 / 86 Key Principles Key Principles Pietro Michiardi (Eurecom) Joint mPlane / BigFoot PhD School: DAY 1 7 / 86 Key Principles Scale out, not up! For data-intensive workloads, a large number of commodity servers is preferred over a small number of high-end servers I Cost of super-computers is not linear I But datacenter efficiency is a difficult problem to solve [2, 4] Some numbers (∼ 2012): I Data processed by Google every day: 100+ PB I Data processed by Facebook every day: 10+ PB Pietro Michiardi (Eurecom) Joint mPlane / BigFoot PhD School: DAY 1 8 / 86 Key Principles Implications of Scaling Out Processing data is quick, I/O is very slow I 1 HDD = 75 MB/sec I 1000 HDDs = 75 GB/sec Sharing vs. Shared nothing: I Sharing: manage a common/global state I Shared nothing: independent entities, no common state Sharing is difficult: I Synchronization, deadlocks I Finite bandwidth to access data from SAN I Temporal dependencies are complicated (restarts) Pietro Michiardi (Eurecom) Joint mPlane / BigFoot PhD School: DAY 1 9 / 86 Key Principles Failures are the norm, not the exception LALN data [DSN 2006] I Data for 5000 machines, for 9 years I Hardware: 60%, Software: 20%, Network 5% DRAM error analysis [Sigmetrics 2009] I Data for 2.5 years I 8% of DIMMs affected by errors Disk drive failure analysis [FAST 2007] I Utilization and temperature major causes of failures Amazon Web Service(s) failures [Several!] I Cascading effect Pietro Michiardi (Eurecom) Joint mPlane / BigFoot PhD School: DAY 1 10 / 86 Key Principles Implications of Failures Failures are part of everyday life I Mostly due to the scale and shared environment Sources of Failures I Hardware / Software I Electrical, Cooling, ... I Unavailability of a resource due to overload Failure Types I Permanent I Transient Pietro Michiardi (Eurecom) Joint mPlane / BigFoot PhD School: DAY 1 11 / 86 Key Principles Move Processing to the Data Drastic departure from high-performance computing model I HPC: distinction between processing nodes and storage nodes I HPC: CPU intensive tasks Data intensive workloads I Generally not processor demanding I The network becomes the bottleneck I MapReduce assumes processing and storage nodes to be collocated ! Data Locality Principle Distributed filesystems are necessary Pietro Michiardi (Eurecom) Joint mPlane / BigFoot PhD School: DAY 1 12 / 86 Key Principles Process Data Sequentially and Avoid Random Access Data intensive workloads I Relevant datasets are too large to fit in memory I Such data resides on disks Disk performance is a bottleneck I Seek times for random disk access are the problem 10 F Example: 1 TB DB with 10 100-byte records. Updates on 1% requires 1 month, reading and rewriting the whole DB would take 1 day1 I Organize computation for sequential reads 1From a post by Ted Dunning on the Hadoop mailing list Pietro Michiardi (Eurecom) Joint mPlane / BigFoot PhD School: DAY 1 13 / 86 Key Principles Implications of Data Access Patterns MapReduce is designed for: I Batch processing I involving (mostly) full scans of the data Typically, data is collected “elsewhere” and copied to the distributed filesystem I E.g.: Apache Flume, Hadoop Sqoop, ··· Data-intensive applications I Read and process the whole Web (e.g. PageRank) I Read and process the whole Social Graph (e.g. LinkPrediction, a.k.a. “friend suggest”) I Log analysis (e.g. Network traces, Smart-meter data, ··· ) Pietro Michiardi (Eurecom) Joint mPlane / BigFoot PhD School: DAY 1 14 / 86 Key Principles Hide System-level Details Separate the what from the how I MapReduce abstracts away the “distributed” part of the system I Such details are handled by the framework BUT: In-depth knowledge of the framework is key I Custom data reader/writer I Custom data partitioning I Memory utilization Auxiliary components I Hadoop Pig I Hadoop Hive I Cascading/Scalding I ... and many many more! Pietro Michiardi (Eurecom) Joint mPlane / BigFoot PhD School: DAY 1 15 / 86 Key Principles Seamless Scalability We can define scalability along two dimensions I In terms of data: given twice the amount of data, the same algorithm should take no more than twice as long to run I In terms of resources: given a cluster twice the size, the same algorithm should take no more than half as long to run Embarrassingly parallel problems I Simple definition: independent (shared nothing) computations on fragments of the dataset I How to to decide if a problem is embarrassingly parallel or not? MapReduce is a first attempt, not the final answer Pietro Michiardi (Eurecom) Joint mPlane / BigFoot PhD School: DAY 1 16 / 86 The Programming Model The Programming Model Pietro Michiardi (Eurecom) Joint mPlane / BigFoot PhD School: DAY 1 17 / 86 The Programming Model Functional Programming Roots Key feature: higher order functions I Functions that accept other functions as arguments I Map and Fold f f f f f g g g g g Figure: Illustration of map and fold. Pietro Michiardi (Eurecom) Joint mPlane / BigFoot PhD School: DAY 1 18 / 86 The Programming Model Functional Programming Roots map phase: I Given a list, map takes as an argument a function f (that takes a single argument) and applies it to all element in a list fold phase: I Given a list, fold takes as arguments a function g (that takes two arguments) and an initial value (an accumulator) I g is first applied to the initial value and the first item in the list I The result is stored in an intermediate variable, which is used as an input together with the next item to a second application of g I The process is repeated until all items in the list have been consumed Pietro Michiardi (Eurecom) Joint mPlane / BigFoot PhD School: DAY 1 19 / 86 The Programming Model Functional Programming Roots We can view map as a transformation over a dataset I This transformation is specified by the function f I Each functional application happens in isolation I The application of f to each element of a dataset can be parallelized in a straightforward manner We can view fold as an aggregation operation I The aggregation is defined by the function g I Data locality: elements in the list must be “brought together” I If we can group elements of the list, also the fold phase can proceed in parallel Associative and commutative operations I Allow performance gains through local aggregation and reordering Pietro Michiardi (Eurecom) Joint mPlane / BigFoot PhD School: DAY 1 20 / 86 The Programming Model Functional Programming and MapReduce Equivalence of MapReduce and Functional Programming: I The map of MapReduce corresponds to the map operation I The reduce of MapReduce corresponds to the fold operation The framework coordinates the map and reduce phases: I Grouping intermediate results happens in parallel In practice: I User-specified computation is applied (in parallel) to all input records of a dataset I Intermediate results are aggregated by another user-specified computation Pietro Michiardi (Eurecom) Joint mPlane / BigFoot PhD School: DAY 1 21 / 86 The Programming Model What can we do with MapReduce? MapReduce “implements” a subset of functional programming I The programming model appears quite limited and strict There are several important problems that can be adapted to MapReduce I We will focus on illustrative cases I We will see in detail “design patterns” F How to transform a problem and its input F How to save memory and bandwidth in the system Pietro Michiardi (Eurecom) Joint mPlane / BigFoot PhD School: DAY 1 22 / 86 The Programming Model Data Structures Key-value pairs are the basic data structure in MapReduce I Keys and values can be: integers, float, strings, raw bytes I They can also be arbitrary data structures The design of MapReduce algorithms involves: 2 I Imposing the key-value structure on arbitrary datasets F E.g.: for a collection of Web pages, input keys may be URLs and values may be the HTML content I In some algorithms, input keys are not used, in others they uniquely identify a record I Keys can be combined in complex ways to design various algorithms 2There’s more about it: here we only look at the input to the map function.
Details
-
File Typepdf
-
Upload Time-
-
Content LanguagesEnglish
-
Upload UserAnonymous/Not logged-in
-
File Pages86 Page
-
File Size-