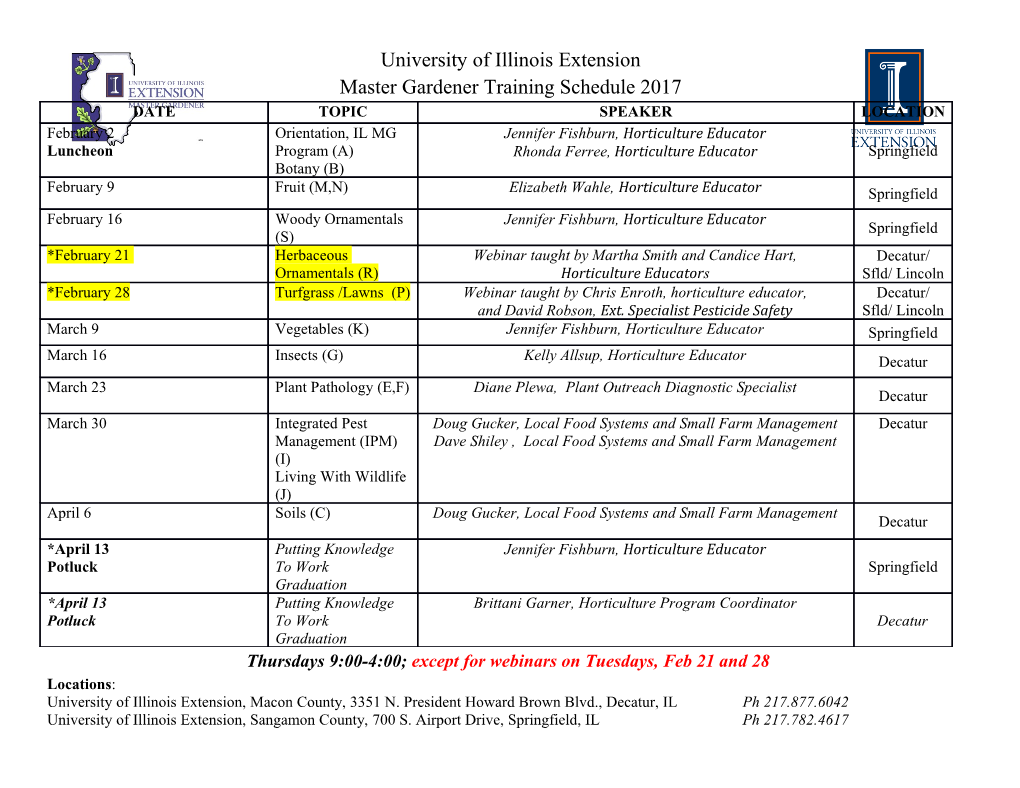
A Comparative Analysis of Empirical Bayes and Bayesian Hierarchical Models in Hotspot Identification By Xiaoyu Guo Graduate Research Assistant Texas A&M Transportation Institute 3135 TAMU, College Station, TX 77843-3135 Tel: (979) 845-8408 fax: (979) 845-6481 Email: [email protected] Lingtao Wu*, Ph.D. Assistant Research Scientist Texas A&M Transportation Institute Texas A&M University System, 3135 TAMU College Station, Texas 77843-3135 Phone: (979) 845-7214, fax: (979) 845-6481 Email: [email protected] Yajie Zou, Ph.D. Associate Professor Department of Transportation Engineering Tongji University 4800 Cao’an Road, Shanghai 201804 Phone: +86 (21) 6958-8152 Email: [email protected] Lee Fawcett, Ph.D. Lecturer School of Mathematics, Statistics & Physics Newcastle University Newcastle upon Tyne, NE1 7RU, UK Phone: +44 (0)191-2087228, fax: +44 (0)191-2087228 Email: [email protected] Word count: 7,488 Words (5,788 Text + 7 Tables * 250 each) November 15, 2018 *Corresponding Author Guo, Wu, Zou, Fawcett 1 1 ABSTRACT 2 Hotspot identification is an important step in the highway safety management process. Errors in 3 hotspot identification (HSID) may result in an inefficient use of limited resources for safety 4 improvements. The empirical Bayesian (EB)-based HSID has been widely applied as an 5 effective approach in identifying hotspots. However, there are some limitations with the EB 6 approach. It assumes that the parameter estimates of the safety performance function (SPF) are 7 correct without any uncertainty, and does not consider temporarily instability in crashes, which 8 has been reported in recent studies. Bayesian hierarchical model is an emerging technique that 9 addresses the limitations on the EB method. Thus, the objective of this study is to compare the 10 performance of the standard EB method and the Bayesian hierarchical model in identifying 11 hotspots. Three methods (i.e. Crash rate, EB, and the Bayesian hierarchical model-based 12 methods) were applied to identify risky intersections with different significant levels. Four 13 evaluation tests (i.e., Site Consistency; Method Consistency; Total Rank Differences; and 14 Poisson Mean Differences tests) were conducted to assess the performance of these three 15 methods. The testing results suggest that: (1) the Bayesian hierarchical model outperforms the 16 crash rate and the EB-based methods in most cases. Bayesian hierarchical model improves the 17 accuracy of HSID significantly; (2) hotspots identified with crash rates are generally unreliable. 18 It is significant for roadway agencies and practitioners to accurately rank sites in the roadway 19 network in order to effectively manage safety investments. Roadway agencies and practitioners 20 are encouraged to consider the Bayesian hierarchical models in identifying hotspots. 21 22 Keywords: Roadway Safety, Hotspot Identification, Empirical Bayes, Bayesian Hierarchical 23 Model Guo, Wu, Zou, Fawcett 2 1 INTRODUCTION 2 The identification of crash hotspots (also known as prone sites, sites with promise, or black 3 spots) is one of the most important tasks in the roadway safety management process. Errors in 4 hotspot identification (HSID) can result in inefficient use of limited resources for safety 5 improvements and cause additional loss of lives. 6 Various methods have been proposed for HSID (1-3), and researchers have been 7 continuously improving the methods (4-8); unfortunately, sites identified by different methods 8 and ranking criteria are not identical (9) (they are not discussed in detail here due to space 9 limitations). Observed crash counts and crash rates were often used by roadway agencies, but 10 analyses have shown that these two methods cannot account for the regression-to-the-mean 11 (RTM) bias and are not reliable (10, 11). Empirical Bayes (EB) based methods have shown 12 superiority in estimating safety as well as in identifying hotspots (8, 10). The standard EB 13 method combines the observed crash counts of one site and the predicted safety of similar sites. 14 The latter one is typically derived from a safety performance function (SPF). The EB method has 15 been included in the first edition of the Highway Safety Manual (12) and is widely used in HSID 16 for its ability in correcting the RTM bias and increasing estimation precision. Although many 17 studies have shown that the EB method always performs better than other common HSID 18 methods, it is not without any limitations. One critical issue with the EB method is the 19 implementation of the SPF to predict crashes. The SPF is usually modeled using crash data 20 occurring at a similar “reference” pool of sites. In the conventional EB method, the SPF assumes 21 the number of crashes at each site follows a Poisson distribution and is independent from each 22 other in different years. In other words, there is no yearly variation in safety at each site when 23 assuming that the traffic volume and other key roadway features remain at the same level. 24 However, this is often not true. With the evolution in vehicles, driving behavior, roadway design 25 standards, etc., the crash data are temporarily instable (13, 14). Without accounting for the 26 instability of crashes, the HSID results estimated through the EB method may be inaccurate 27 under certain situations. In addition, the EB method assumes that the parameters in a fitted SPF 28 are the true estimates, which is also a problematic assumption, especially when the sample siZe 29 of reference sites is low (15-17). 30 Recently, Fawcett et al. (18) proposed a novel Bayesian hierarchical model (denoted as 31 Bayesian hierarchical model hereafter) for estimating and predicting roadway safety. The main 32 advantage of the Bayesian hierarchical model is incorporating crash counts from multiple time- 33 points, with the counts in more recent years lending more weight to safety estimates than the 34 counts from time-points further in the past. The proposed model is able to capture the temporary 35 trend of crashes at a site. Previous study has discussed the structure of the Bayesian hierarchical 36 model and its application to real crash data. It was also noted that the standard EB method is a 37 special case of the Bayesian hierarchical model (18). However, the HSID results have not been 38 examined between the two methods. It is unknown whether or not the novel Bayesian 39 hierarchical model outperforms the commonly used EB method in HSID. As a result, the primary 40 purpose of this paper is to comparatively analyze the performance between the EB method and 41 the Bayesian hierarchical model in HSID. To achieve the objective, this study identifies hotspots Guo, Wu, Zou, Fawcett 3 1 of intersections using the two methods, separately. Evaluation tests are conducted to assess the 2 performance of each method. 3 METHODOLOGY 4 The following two sections introduce the EB method and Bayesian hierarchical model for 5 identifying hotspots, separately. For comparison purpose, the crash rate-based HSID method is 6 also discussed in the first section. 7 Crash Rate and EB-based HSID Methods 8 As the name implies, the crash rate-based method mainly calculates the rate of crashes at each 9 site, and ranks the sites by their rates. It is usually calculated by dividing the observed crash 10 number by exposure (e.g., vehicle miles of travelling, or VMT). There are a few types of crash 11 rates: target crash rates (considering target collisions only), equivalent crash rate (converting 12 crashes into the same severity level by different weight), etc. For the purpose of this study, we 13 consider the number of total crashes, and the exposure as traffic volume traveling through an 14 intersection (see Data Description for more details). Thus, the crash rate for a site is calculated 15 as: � !,# $ Crash Rate!,# = × 10 (1) Volume!,# × � 16 where: $ 17 Crash Rate!,# = crash rate at site i in period j (per 10 vehicles per year); 18 �!,# = total number of observed crashes at site i in period j; 19 N = number of years in period j; and, 20 Volume!,# = traffic volume at site i in period j. 21 Since the crash rate simply relies on the observed number of crashes and exposure at the 22 sites, the randomness of crashes and RTM bias are not well addressed. It has been pointed out 23 that the HSID results using crash rates are not reliable (10, 19, 20). Safety researchers proposed 24 using an EB approach to correct for the RTM bias (21-23). With the EB approach, an estimate of 25 the long-term safety of an entity is obtained from two sources, as described above. Let K be the 26 observed number of crashes which is Poisson-distributed, and let k be the expected crash count; 27 the EB estimator of k is given as: �6 = � × �(�) + (1 − �) × �. (2) 28 �6 denotes the EB estimate of the expected number of crashes. E(k) can be estimated by 29 the crash prediction model. Many statistical models have been proposed to predict safety by 30 transportation safety analysts (24, 25), for instance, the negative binomial (NB) (26), the 31 Poisson-lognormal (27, 28), the Conway-Maxwell-Poisson (29, 30), the gamma (31), the Guo, Wu, Zou, Fawcett 4 1 Sichel(11, 24), the negative binomial-Lindley models (32), and others (33, 34). Among these 2 models, the NB model has been the most frequently used for predicting crashes. The NB model 3 has the following structure: the number of crashes y during a given time period is assumed to be 4 Poisson- distributed, the probability mass function (PMF) for which is given by: %!×'() (,%) �(�|�) = , l > 0, y = 0, 1, 2, …, (3) .! 5 where � = mean response of the observed crash counts during given period.
Details
-
File Typepdf
-
Upload Time-
-
Content LanguagesEnglish
-
Upload UserAnonymous/Not logged-in
-
File Pages19 Page
-
File Size-