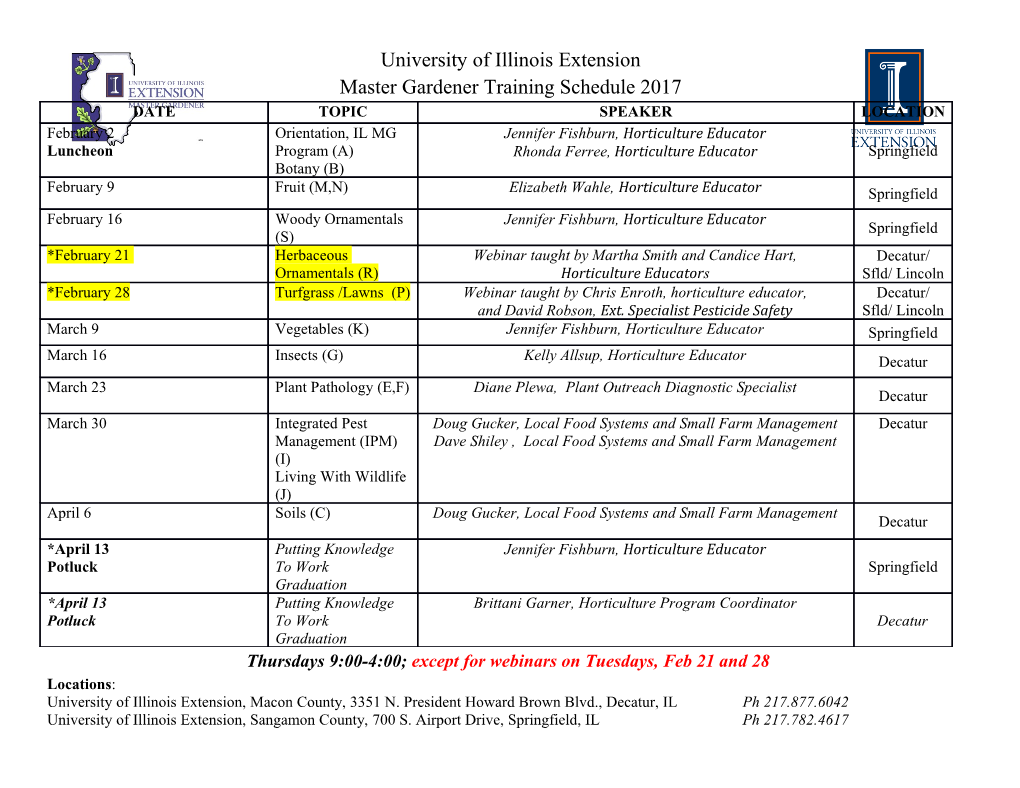
GPU Basics GPU Basics S. Sundar & M. Panchatcharam Types of Parallelism Task-based parallelism Data-Based Parallelism S. Sundar and M. Panchatcharam FLYNN’s Taxonomoy Other Parallel Patterns Classes of Parallel Computers Simple Coding August 9, 2014 47 Outline GPU Basics S. Sundar & M. Panchatcharam 1 Task-based parallelism Task-based parallelism Data-Based Parallelism 2 Data-Based Parallelism FLYNN’s Taxonomoy Other Parallel Patterns Classes of Parallel 3 FLYNN’s Taxonomoy Computers Simple Coding 4 Other Parallel Patterns 5 Classes of Parallel Computers 6 Simple Coding 47 Pipeline parallelism GPU Basics S. Sundar & M. Panchatcharam 3 Task-based parallelism Data-Based Parallelism A typical OS, exploit a type of parallelism called task-based FLYNN’s Taxonomoy parallelism Other Parallel Patterns Classes of Parallel Example: A user read an article on a website while playing Computers music from media player Simple Coding In terms of parallel programming, the linux operator uses pipe commands to execute this 47 Coarse/fine-grained parallelism GPU Basics S. Sundar & M. Panchatcharam 4 Task-based parallelism Applications are often classified according to how often their Data-Based Parallelism subtasks need to synchronize or communicate with each other FLYNN’s Taxonomoy If subtasks communicate many times per second, then it is Other Parallel Patterns called fine-grained parallelism Classes of Parallel Computers If they do not communicate many times per second, then it is Simple Coding called coarser-grained parallelism If they rarely or never have to communicate, then it is called embarrassingly parallel Embarrassingly parallel applications are easiest to parallelize. 47 Task Parallelism GPU Basics S. Sundar & M. Panchatcharam 5 Task-based parallelism Data-Based Parallelism Task Parallelism FLYNN’s Taxonomoy In a multiprocessor system, task parallelism is achieved when each Other Parallel Patterns Classes of Parallel processor executes different thread on the same or different data. Computers Simple Coding Threads may execute same or different code communication between threads takes place by passing data from one thread to next 47 Data Parallelism GPU Basics S. Sundar & M. Panchatcharam Task-based parallelism 6 Data-Based Parallelism Data Parallelism FLYNN’s Taxonomoy In a multiprocessor system, each processor performs the same task Other Parallel Patterns on different pieces of distributed data Classes of Parallel Computers Simple Coding Consider adding two matrices. Addition is the task on different piece of data (each element) It is a fine-grained parallelism 47 Instruction Level Parallelism GPU Basics S. Sundar & M. Panchatcharam Task-based parallelism 7 Data-Based Parallelism Instruction level parallelism is a measure of how many of the FLYNN’s Taxonomoy operations in a computer program can be performed Other Parallel Patterns simultaneously. The potential overlap among instructions is called Classes of Parallel Computers instruction level parallelism. Simple Coding Hardware level works on dynamic parallelism Software level works on static parallelism 47 Taxonomy GPU Basics S. Sundar & M. Panchatcharam Task-based parallelism Data-Based Parallelism Flynn’s Taxonomy is a classification of different computer 8 FLYNN’s Taxonomoy architectures. Various types are as follows: Other Parallel Patterns SIMD - Single Instruction Multiple Data Classes of Parallel Computers MIMD - Multiple Instruction Multiple Data Simple Coding SISD - Single Instruction Single Data MISD - Multiple Instruction Single Data 47 SISD GPU Basics S. Sundar & M. Panchatcharam Standard serial programming Task-based parallelism Single instruction stream on a single data Data-Based Parallelism Single-core CPU is enough 9 FLYNN’s Taxonomoy Other Parallel Patterns Classes of Parallel Computers Simple Coding 47 MIMD GPU Basics S. Sundar & Today’s dual or quad-core desktop machines M. Panchatcharam Work allocated in one of N CPU cores Task-based parallelism Data-Based Each thread has independent stream of instructions Parallelism Hardware has the control logic for decoding separate 10 FLYNN’s Taxonomoy instruction streams Other Parallel Patterns Classes of Parallel Computers Simple Coding 47 SIMD GPU Basics S. Sundar & Type of Data parallelism M. Panchatcharam Single instruction stream at any one point of time Task-based parallelism Data-Based Single set of logic to decode and execute the instruction Parallelism stream 11 FLYNN’s Taxonomoy Other Parallel Patterns Classes of Parallel Computers Simple Coding 47 MISD GPU Basics Many functional units perform different operations on the S. Sundar & same data M. Panchatcharam Task-based parallelism Pipeline architectures belong to this type Data-Based Example: Horner’s Rule is an example Parallelism 12 FLYNN’s Taxonomoy Other Parallel Patterns y = (··· (((an∗x+an−1)∗x+an−2)∗x+an−3)∗x+···+a1)∗x+a0 Classes of Parallel Computers Simple Coding 47 Loop based Pattern GPU Basics S. Sundar & M. Panchatcharam Task-based parallelism Data-Based Parallelism FLYNN’s Taxonomoy Are you familiar with loop structures? 13 Other Parallel Patterns Classes of Parallel Types of loop? Computers Entry level loop Simple Coding Exit level loop 47 Loop based Pattern GPU Basics S. Sundar & M. Panchatcharam Task-based parallelism Data-Based Easy pattern to parallelize Parallelism With inter-loop dependencies removed, decide splitting or FLYNN’s Taxonomoy 14 Other Parallel Patterns partition the work between available processors Classes of Parallel Optimize communication between processors and the use of Computers on chip resources Simple Coding Communication overhead is the bottleneck Decompose based on the number of logical hardware threads available 47 Loop based Pattern GPU Basics S. Sundar & M. Panchatcharam Task-based parallelism Data-Based However, oversubscribing the number of threads leads to poor Parallelism FLYNN’s Taxonomoy performance 15 Other Parallel Patterns Reason: Context switching performed in software by the OS Classes of Parallel Computers Aware of hidden dependencies while doing existing serial Simple Coding implementation Concentrate on inner loops and one or more outer loops Best approach, parallelize only the outer loops 47 Loop based Pattern GPU Basics S. Sundar & M. Panchatcharam Task-based parallelism Data-Based Parallelism Note: Most loop can be flattened FLYNN’s Taxonomoy Reduce inner loop and outer loop to a single loop, if possible 16 Other Parallel Patterns Classes of Parallel Example: Image processing algorithm Computers X pixel axis in the inner loop and Y axis in the outer loop Simple Coding Flatten this loop by considering all pixels as a single dimensional array and iterate over image coordinates 47 Fork/Join Pattern GPU Basics S. Sundar & M. Panchatcharam Task-based parallelism It is a common pattern in serial programming where there are Data-Based synchronization points and only certain aspects of the Parallelism program are parallel. FLYNN’s Taxonomoy 17 Other Parallel Patterns The serial code reaches the work that can be distributed to P Classes of Parallel processors in some manner Computers Simple Coding It then Forks or spawns N threads/processes that perform the calculation in parallel. These processes execute independently and finally converge or join once all the calculations are complete A typical approach found in OpenMP 47 Fork/Join Pattern GPU Basics Code splits into N threads and later converges to a single S. Sundar & thread again M. Panchatcharam See figure, we see a queue of data items Task-based parallelism Data-Based Data items are split into three processing cores Parallelism Each data item is processed independently and later written FLYNN’s Taxonomoy to appropriate destination place 18 Other Parallel Patterns Classes of Parallel Computers Simple Coding 47 Fork/Join Pattern GPU Basics S. Sundar & M. Panchatcharam Typically implemented with partitioning of the data Task-based parallelism Data-Based Serial code launches N threads and divide the dataset equally Parallelism between the N threads FLYNN’s Taxonomoy 19 Other Parallel Patterns Works well, if each packet of data takes same time to process Classes of Parallel Computers If one thread takes too much time to work, it becomes single Simple Coding factor determining the local time Why did we choose three threads, Instead of six threads? Reality: Millions of data items attempting to fork million threads will cause almost all OS to fail OS applies "fair scheduling policy" 47 Fork/Join Pattern GPU Basics S. Sundar & M. Panchatcharam Task-based parallelism Data-Based Programmer and many multithreaded libraries will use the Parallelism number of logical processor threads available as number of FLYNN’s Taxonomoy processes to fork 20 Other Parallel Patterns Classes of Parallel CPU threads are so expensive to create, destroy and utilize Computers Simple Coding Fork/join pattern is useful when there is an unknown amount of concurrency available in a problem Traversing a tree structure may fork additional threads when it encounters another node 47 Divide and Conquer Pattern GPU Basics S. Sundar & M. Panchatcharam Task-based parallelism Data-Based Parallelism A pattern for breaking down(divide) large problems into FLYNN’s Taxonomoy smaller sections each of which can be conquered 21 Other Parallel Patterns Classes of Parallel Useful with recursion Computers Example: Quick Sort Simple Coding Quick sort recursively partitions the data into two sets, above pivot point and below pivot point 47 Divide and Conquer Pattern GPU Basics S. Sundar & M. Panchatcharam Task-based
Details
-
File Typepdf
-
Upload Time-
-
Content LanguagesEnglish
-
Upload UserAnonymous/Not logged-in
-
File Pages47 Page
-
File Size-