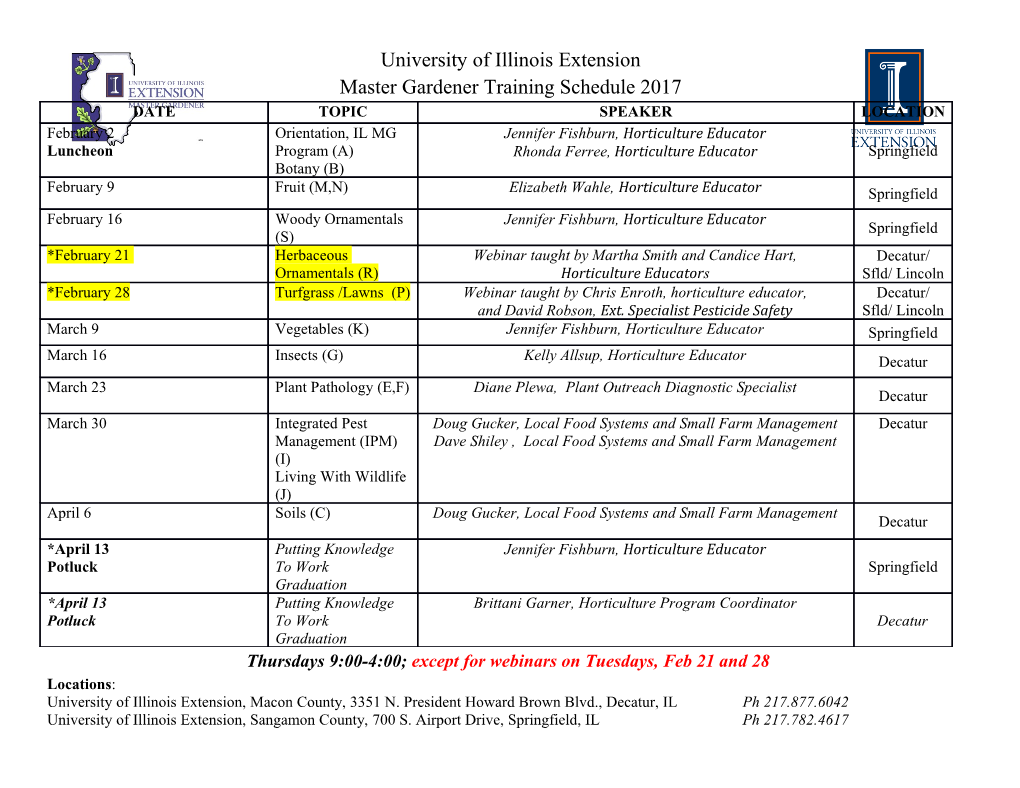
Copyright Warning & Restrictions The copyright law of the United States (Title 17, United States Code) governs the making of photocopies or other reproductions of copyrighted material. Under certain conditions specified in the law, libraries and archives are authorized to furnish a photocopy or other reproduction. One of these specified conditions is that the photocopy or reproduction is not to be “used for any purpose other than private study, scholarship, or research.” If a, user makes a request for, or later uses, a photocopy or reproduction for purposes in excess of “fair use” that user may be liable for copyright infringement, This institution reserves the right to refuse to accept a copying order if, in its judgment, fulfillment of the order would involve violation of copyright law. Please Note: The author retains the copyright while the New Jersey Institute of Technology reserves the right to distribute this thesis or dissertation Printing note: If you do not wish to print this page, then select “Pages from: first page # to: last page #” on the print dialog screen The Van Houten library has removed some of the personal information and all signatures from the approval page and biographical sketches of theses and dissertations in order to protect the identity of NJIT graduates and faculty. ABSTRACT APPROXIMATE STRING MATCHING METHODS FOR DUPLICATE DETECTION AND CLUSTERING TASKS by Oleksandr Rudniy Approximate string matching methods are utilized by a vast number of duplicate detection and clustering applications in various knowledge domains. The application area is expected to grow due to the recent significant increase in the amount of digital data and knowledge sources. Despite the large number of existing string similarity metrics, there is a need for more precise approximate string matching methods to improve the efficiency of computer-driven data processing, thus decreasing labor-intensive human involvement. This work introduces a family of novel string similarity methods, which outperform a number of effective well-known and widely used string similarity functions. The new algorithms are designed to overcome the most common problem of the existing methods which is the lack of context sensitivity. In this evaluation, the Longest Approximately Common Prefix (LACP) method achieved the highest values of average precision and maximum F1 on three out of four medical informatics datasets used. The LACP demonstrated the lowest execution time ensured by the linear computational complexity within the set of evaluated algorithms. An online interactive spell checker of biomedical terms was developed based on the LACP method. The main goal of the spell checker was to evaluate the LACP method’s ability to make it possible to estimate the similarity of resulting sets at a glance. The Shortest Path Edit Distance (SPED) outperformed all evaluated similarity functions and gained the highest possible values of the average precision and maximum F1 measures on the bioinformatics datasets. The SPED design was inspired by the preceding work on the Markov Random Field Edit Distance (MRFED). The SPED eradicates two shortcomings of the MRFED, which are prolonged execution time and moderate performance. Four modifications of the Histogram Difference (HD) method demonstrated the best performance on the majority of the life and social sciences data sources used in the experiments. The modifications of the HD algorithm were achieved using several re- scorers: HD with Normalized Smith-Waterman Re-scorer, HD with TFIDF and Jaccard re-scorers, HD with the Longest Common Prefix and TFIDF re-scorers, and HD with the Unweighted Longest Common Prefix Re-scorer. Another contribution of this dissertation includes the extensive analysis of the string similarity methods evaluation for duplicate detection and clustering tasks on the life and social sciences, bioinformatics, and medical informatics domains. The experimental results are illustrated with precision-recall charts and a number of tables presenting the average precision, maximum F1, and execution time. APPROXIMATE STRING MATCHING METHODS FOR DUPLICATE DETECTION AND CLUSTERING TASKS by Oleksandr Rudniy A Dissertation Submitted to the Faculty of New Jersey Institute of Technology in Partial Fulfillment of the Requirements for the Degree of Doctor of Philosophy in Computer Science Department of Computer Science January 2012 Copyright © 2012 by Oleksandr Rudniy ALL RIGHTS RESERVED APPROVAL PAGE APPROXIMATE STRING MATCHING METHODS FOR DUPLICATE DETECTION AND CLUSTERING TASKS Oleksandr Rudniy Dr. James Geller, Dissertation Co-Advisor Date Professor of Computer Science, NJIT Dr. Min Song, Dissertation Co-Advisor Date Assistant Professor of Information Systems, NJIT Dr. Narain Gehani, Committee Member Date Professor of Computer Science, NJIT Dean of College of Computing Sciences, NJIT Dr. Vincent Oria, Committee Member Date Associate Professor of Computer Science, NJIT Dr. Xiaohua Tony Hu, Committee Member Date Associate Professor of Drexel University, Philadelphia, PA BIOGRAPHICAL SKETCH Author: Oleksandr Rudniy Degree: Doctor of Philosophy Date: January 2012 Undergraduate and Graduate Education: • Ph.D. Student in Computer Science, New Jersey Institute of Technology, Newark, NJ, August 2011 • Master of Science in Applied Mathematics, Kharkiv State University of Radio Electronics, Kharkiv, Ukraine, 2001 • Bachelor of Science in Applied Mathematics, Kharkiv State University of Radio Electronics, Kharkiv, Ukraine, 2000 Presentations and Publications: M. Song and A. Rudniy, "Detecting duplicate biological entities using Markov random field-based edit distance," Knowledge and Inform. Syst. , vol. 25, no. 2, pp. 371-387, 2010. A. Rudniy et al., "Detecting duplicate biological entities using Shortest Path Edit Distance," Int. J. of Data Mining and Bioinf. , vol. 4, no. 4, pp. 395-410, 2010. A. Rudniy et al., "Shortest Path Edit Distance for Enhancing UMLS Integration and Audit," in Proc. of AMIA 2010 Symp. , Washington, D.C., 2010, pp. 697-701. A. Rudniy et al., "Shortest Path Edit Distance for Detecting Duplicate Biological Entities," in Proc. of ACM Int. Conf. on Bioinf. and Comput. Biol. , Niagara Falls, NY, 2010, pp. 442-444. M. Song and A. Rudniy, "Detecting Duplicate Biological Entities Using Markov Random Field-Based Edit Distance," in IEEE Int. Conf. on Bioinf. and Biomed. , Philadelphia, PA, 2008, pp. 457-460. A. Rudniy et al., "Histogram Difference String Distance for Enhancing Ontology Integration in Bioinformatics," submitted to Int. Conf. on Bioinf. and Comp. Biol. iv TABLE OF CONTENTS Chapter Page 1 INTRODUCTION……............................………………..…………………………. 1 1.1 Motivation .........………………………………….......................................... 1 1.1.1 Bioinformatics Applications ………………............................................ 1 1.1.2 Medical Informatics Applications ........................................................... 2 1.1.3 Human Speech Applications ................................................................... 2 1.1.4 Signal Processing Applications ............................................................... 3 1.1.5 Text Retrieval Applications ..................................................................... 3 1.1.6 Musical Data Mining Applications ......................................................... 4 1.1.7 Bird Vocalization Applications ............................................................... 4 1.1.8 Document Clustering Applications .…………………………………… 5 1.2 Organization of the Dissertation …………………............................................. 7 2 BACKGROUND …………………………………………….………….......……… 9 2.1 Summary ….......………………………………….............................................. 9 2.2 Review of the Related Work ….......………………………............................... 9 2.3 Research Problem ……….………………………….......................................... 14 2.3.1 Research Problem in Life and Social Sciences Domain ...…………... 15 2.3.2 Research Problem in Bioinformatics Domain ……………………….. 16 2.3.3 Research Problem in Medical Informatics Domain …………………. 19 2.4 String Distances ……………………………………………………………….. 20 2.4.1 Edit Distance ........................................................................................... 20 2.4.2 Traces ...................................................................................................... 21 2.4.3 Edit Path .................................................................................................. 21 v TABLE OF CONTENTS (Continued) Chapter Page 2.4.4 Post-Normalized Edit Distance .............................................................. 23 2.4.5 Normalized Edit Distance ....................................................................... 24 2.4.6 Damerau-Levenshtein Distance .............................................................. 24 2.4.7 Jaro Metric .............................................................................................. 25 2.4.8 Jaro-Winkler Metric ............................................................................... 25 2.4.9 Jaccard Similarity ................................................................................... 26 2.4.10 Smith-Waterman Algorithm ................................................................... 26 2.4.11 Gotoh Algorithm .................................................................................... 27 2.4.12 Monge-Elkan Algorithm ........................................................................ 28 2.4.13 Needleman-Wunsch Algorithm .............................................................
Details
-
File Typepdf
-
Upload Time-
-
Content LanguagesEnglish
-
Upload UserAnonymous/Not logged-in
-
File Pages177 Page
-
File Size-