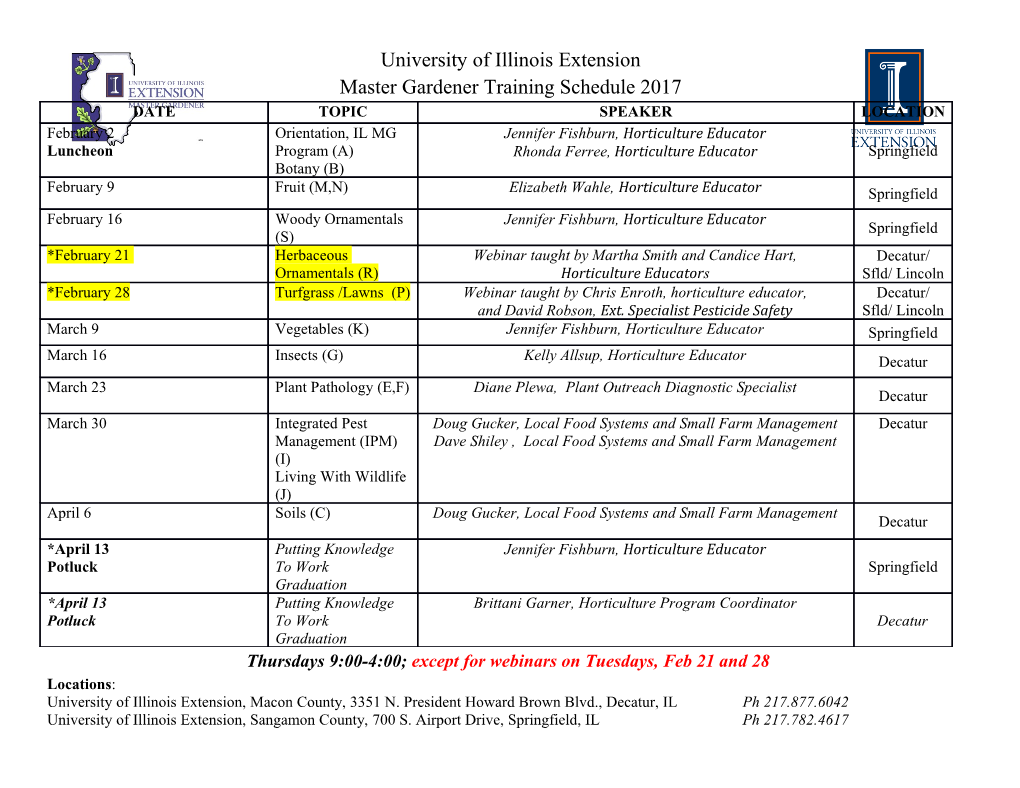
Bernoulli 6(6), 2000, 1109±1120 The central limit theorem under censoring MICHAEL G. AKRITAS Department of Statistics, Penn State University, 326 Thomas Bldg., University Park PA 16802, USA. E-mail: [email protected] The central limit theorem for integrals of the Kaplan±Meier estimator is obtained. The basic tools are the martingale methods developed by Gill and the identities and inequalities of Efron and Johnstone. The assumptions needed are both weaker and more transparent than those in the recent literature, and the resulting variance expression is simpler, especially for distributions with atoms. Keywords: distributions with atoms; i.i.d. representation; Kaplan±Meier integrals; martingales for counting processes 1. Introduction A number of recent papers have been putting the ®nal touches to the asymptotic theory of the Kaplan±Meier estimator (Kaplan and Meier 1958) and functionals based on it (see Wang 1987; Gijbels and Veraverbeke 1991; Einmahl and Koning 1992; Gill 1994; Stute 1995). The last paper cited establishes a central limit theorem (CLT) for a Kaplan±Meier integral by ®rst expressing it as a sum of independent and identically distributed (i.i.d.) random variables plus an asymptotically negligible remainder term. Stute's result allows both discontinuous populations and a general class of functions, thus generalizing other CLT results (Gill 1983; Schick et al. 1988; Yang 1994). However, it is obtained using a delicate (and computation- intensive) approach based on U-statistic approximations. He justi®es this approach by citing dif®culties in the application of the counting processes techniques, and requires stronger assumptions than those used with martingale methods. In addition, the expression for the terms in his i.i.d. representation (and consequently for the asymptotic variance) is quite complicated, especially for distributions with atoms. The main purpose of the present paper is to prove the CLT and provide an alternative i.i.d. representation with simpler terms and under weaker conditions. This is made possible by using the martingale methods developed by Gill (1980; 1983), and the identities and inequalities of Efron and Johnstone (1990). With the present approach the CLT is established directly, not as a consequence of the i.i.d. representation. These techniques require that the Kaplan±Meier integral be re-expressed as an integral in terms of the cumulative hazard function. Efron and Johnstone (1990) studied extensively the conse- quences of such re-expressions in the uncensored data context, and their results are central to understanding the relation between the expressions given here and those of Stute (1995). It will be seen that the variance expression in the present paper is related to Efron and Johnstone's `advance time' transformation A, while the terms in our i.i.d. representation are related to its adjoint transformation B. Surprisingly, expressions resulting from these transformations do not change much under random censoring, while the traditional 1350±7265 # 2000 ISI/BS 1110 M.G. Akritas expressions which Stute (1995) worked with do. This explains why the present i.i.d. representation and formula for the asymptotic variance are simpler under censoring. The next section sets the notation and formulates the main result. The longer proofs are given in Section 3. 2. Central limit theorem and i.i.d. representation 2.1. Notation and assumptions Let Ti, i 1, ..., n, be i.i.d. random variables on the real line and let F denote their common distribution function. The survival function of the Ti will be denoted by S, and the t 1 cumulative hazard function by Ë; thus S 1 F and Ë(t) 1[S(x)] dF(x). The observed data consist of X i min(Ti, Ci) and Äi I(X i Ti), i 1, ..., n, (1) where C1, ..., Cn are i.i.d. random variables which are also independent of the Ts, and I(E) denotes the indicator of the event E. The common distribution of the Ci will be denoted by G. The distribution function of the X i will be denoted by H. Thus, 1 H (1 F)(1 G). Pn Pn Let Ni(t) I(X i < t, Äi 1), N:(t) i1 Ni(t), Yi(t) I(X i > t), Y:(t) i1Yi(t). We will assume that all random variables are de®ned on the probability space (Ù, F , P) and we will consider the ®ltration F t N _ ó f(X i, Äi)I(X i < s), I(X i . s):1 , s < t, i 1, ..., ng, (2) t where N consists of all P-null sets of F . Then, Mi(t) Ni(t) 1 Yi(s)dË(s)isa martingale with respect to the ®ltration in (2). By analogy with the notation introduced above, Pn M:(t) i1 Mi(t). The Kaplan and Meier (1958) product-limit estimator (PLE) of S based on the observations (1) will be denoted by S^, while F^ 1 S^, Ë^ will denote the corresponding estimators of F, Ë, respectively. A(s)orA(s) will denote the left- continuous version of a right-continuous function A, and ÄA(s) A(s) A(s). Unless otherwise explicitly indicated, the domain of integration includes the upper and lower integration limits. Finally, de®ne ôn max(X 1, ..., X n), and let ôF supfx : F(x) , 1g, for any distribution function F. 2 Let ö : R ! R be any measurable function such that ö dF , 1. The objective of this paper is to obtain a CLT for the Kaplan±Meier integral ö dF^ and to derive an i.i.d. representation for it. The only assumption needed for the CLT and i.i.d. representation is the following: Assumption 1. Let ô ô H . Then ô ö(s)2 dF(s) , 1: 1 1 G(s) Note that assumption (1.5) of Stute (1995) is analogous to the present Assumption 1, and The central limit theorem under censoring 1111 apparently of comparable strength. Indeed, in the uncensored case both reduce to ö(s)2 dF(s) , 1, while if both F and G are continuous Stute shows that his assumption (1.5) reduces to the simple and transparent form of the present Assumption 1 (see his relation (1.9)). Therefore, his assumption (1.6) is an additional assumption which is not needed in the present derivation of the CLT. For any integrable function ö, we de®ne 1 ö(s) ö(t)dF(t): (3) S(s) (s,ô] p The asymptotic variance of n ö dF^ will be ô S(s) ó 2 [ö(s) ö(s)]2 dF(s), (4) 1 1 H(s) provided that ôn , ôF almost surely, or ö(ôF ) 0. Remark 1. The assumption that ôn , ôF a.s. is also used, for example, in Shorack and Wellner (1986, p. 301), but we remark here that this entails no loss of generality. Indeed, if ô ^ ô ^ ô ^ ôn ôF , then 1 ö d(F F) 1(ö ö(ô))d(F F) 1(ö ö(ô))d(F F), and the asymptotic distribution in this case follows from the ®rst since, by rede®ning ö, the condition ö(ôF ) 0 is satis®ed. 1=2 Remark 2. Set ø(s) ö(s)(1 G(s)) , so that, according to Assumption 1, ô 2 1 ø(s) dF(s) , 1. Assume for the moment that ô ôF . Then, the expression for the variance of ø(T) obtained in Efron and Johnstone (1990) (see their relations (1.8), (3.16) and Section 4 for the continuous, discrete and general case, respectively) is ô S(s) (ø(s) ø(s))2 dF(s): (5) 1 S(s) Therefore the expression in (5) is ®nite, and since S(s)=S(s) < 1, ø 2 L 2(F) and L 2 spaces are linear, we obtain ô S(s)=S(s)ø(s)2 dF(s) , 1. Repeating the same argument 1 ô 2 for |ø|, it follows that 1(S(s)=S(s))jø(s)j dF(s) , 1. If in this last relation we evaluate 1=2 (1 G) at the lower limit of the inside integral (the one de®ning jøjand pull it out of that integral, we obtain !2 ô S(s) 1 jö(x)j dF(x) dF(s) , 1: (6) 1 1 H(s) S(s) (s,ô] If ô , ôF , the above arguments applied to the conditional distribution of T given that it is at most ô, imply that (6) holds with F replaced by (F(s)=F(ô))I(s < ô) I(s > ô), and thus also for F. Relation (6) will be useful for bounding certain quantities in the proofs. In particular, Assumption 1 and relation (6) imply that ó 2 , 1. Remark 3. It is easy to see that with uncensored data the variance expression given in Corollary 1.2 of Stute (1995) reduces to var(ö(T)), while the variance expression in (4) 1112 M.G. Akritas reduces to (5). Thus, (4) is related to the `advance time' transformation A of Efron and Johnstone (1990). While the latter variance expression is practically never used with uncensored data (due to its unusual form), its advantage is that it undergoes a very minor modi®cation under censoring. Furthermore, Corollary 1.2 of Stute (1995) shows that the traditional variance expression becomes considerably more complicated under censoring. An alternative expression for ó 2 in (4), which is perhaps more familiar in the biostatistical literature, is ô ó 2 h(s)(1 ÄË(s))dË(s), (7) 1 where I(s < ô) h(s) h(s; ô) ö~(s)2 , (8) S(s)(1 G(s)) "# 1 ö~(s) ö~(s; ô) S(s) ö(s) ö(t)dF(t) : (9) S(s) (s,ô] The function ö~ will appear again later in the re-expression of the Kaplan±Meier integral as an integral in terms of the cumulative hazard function.
Details
-
File Typepdf
-
Upload Time-
-
Content LanguagesEnglish
-
Upload UserAnonymous/Not logged-in
-
File Pages12 Page
-
File Size-