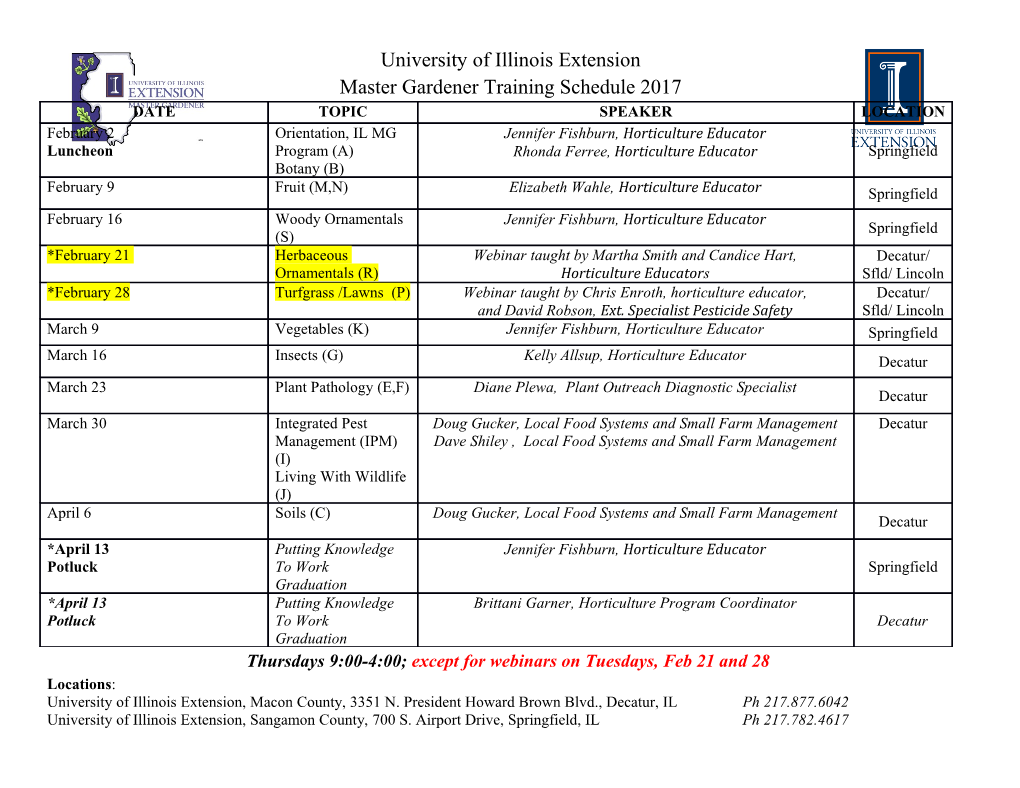
RANK-BASED TEMPO-SPATIAL CLUSTERING: A FRAMEWORK FOR RAPID OUTBREAK DETECTION USING SINGLE OR MULTIPLE DATA STREAMS by Jialan Que Bachelor of Science, Nankai University, China, 2001 Master of Science, Nankai University, China, 2004 Master of Science, University of Pittsburgh, 2008 Submitted to the Graduate Faculty of Intelligent Systems Program in partial fulfillment of the requirements for the degree of Doctor of Philosophy University of Pittsburgh 2012 UNIVERSITY OF PITTSBURGH INTELLIGENT SYSTEMS PROGRAM This dissertation was presented by Jialan Que It was defended on April 13, 2012 and approved by Gregory F. Cooper, Associate Professor, Biomedical Informatics and Intelligent Systems Roger S. Day, Associate Professor, Biomedical Informatics and Biostatistics Milos Hauskrecht, Associate Professor, Computer Science Dissertation Advisor: Fu-Chiang Tsui, Research Assistant Professor, Biomedical Informatics and Intelligent Systems ii Copyright © by Jialan Que 2012 iii RANK-BASED TEMPO-SPATIAL CLUSTERING: A FRAMEWORK FOR RAPID OUTBREAK DETECTION USING SINGLE OR MULTIPLE DATA STREAMS Jialan Que, PhD University of Pittsburgh, 2012 In recent decades, algorithms for disease outbreak detection have become one of the main interests of public health practitioners as a way to identify and localize an outbreak as early as possible in order to inform further public health response to prevent a pandemic from developing. Today’s increased threat of biological warfare and terrorism provide an even stronger impetus to develop methods for outbreak detection based on symptoms as well as definitive laboratory diagnoses. In this dissertation work, I explore the problems inherent to rapid disease outbreak detection using both spatial and temporal information. I develop a framework of non- parameterized algorithms which search for patterns of disease outbreak in spatial sub- regions of the monitored region within a certain period. Compared to the current existing spatial or tempo-spatial algorithm, the algorithms in this framework provide a methodology for fast searching of either a univariate data set or multivariate data set. It first measures how likely a study area has an outbreak occur given the baseline data and currently observed data. Then it applies a greedy searching mechanism to look for clusters with high posterior probabilities given the risk measurement for each unit area as a heuristic. The performance of the proposed algorithms is then evaluated. From the perspective of predictive modeling, I adopted a Gamma-Poisson (GP) model to compute the probability of having an outbreak in each cluster when analyzing iv univariate data. I built a multinomial generalized Dirichlet (MGD) model to identify outbreak clusters from multivariate data that include the OTC data streams collected by the national retail data monitor (NRDM) [1] and the ED data streams collected by the RODS system [2]. Key contributions of this dissertation include 1) the introduction of a rank-based tempo-spatial clustering algorithm, RSC, which utilizes greedy searching and a Bayesian GP model for disease outbreak detection with comparable detection timeliness, cluster positive prediction value (PPV) and improved running time; 2) the proposing of a multivariate extension of RSC (MRSC) which applies an MGD model. The evaluation demonstrates the advantage of the MGD model in effectively suppressing the false alarms caused by baseline shifts. v TABLE OF CONTENTS TABLE OF CONTENTS ................................................................................................... vi LIST OF TABLES ............................................................................................................. ix LIST OF FIGURES ........................................................................................................... xi 1.0 Introduction .............................................................................................................. 1 1.1 Research domain .................................................................................................. 2 1.2 Overview of the proposed methodology .............................................................. 4 1.3 Dissertation hypothesis ........................................................................................ 5 1.4 Guide for the reader.............................................................................................. 6 2.0 Background .............................................................................................................. 9 2.1 Bayesian framework ........................................................................................... 10 2.1.1 Bayes’ Theorem ...................................................................................... 10 2.2 Priors .................................................................................................................. 10 2.3 Several statistical distributions ........................................................................... 12 2.3.1 Gamma and Poisson distribution ............................................................ 12 2.3.2 Multinomial distribution ......................................................................... 12 2.3.3 Dirichlet distribution ............................................................................... 14 2.3.4 Generalized Dirichlet distribution ........................................................... 17 3.0 Related work .......................................................................................................... 20 3.1 Temporal detection methods .............................................................................. 21 3.2 Spatial and tempo-spatial detection methods ..................................................... 21 3.2.1 Frequentist approaches ............................................................................ 22 3.2.2 Bayesian approaches ............................................................................... 24 3.2.3 Issues of algorithms using scan statistics ................................................ 26 3.3 Multivariate methods.......................................................................................... 28 3.4 Calculation of baselines ..................................................................................... 35 3.5 Computational considerations ............................................................................ 37 vi 3.6 The hypothetical advantages of proposed algorithms ........................................ 38 4.0 The experimental domain ...................................................................................... 41 4.1 Background data ................................................................................................. 42 4.1.1 ED data .................................................................................................... 42 4.1.2 OTC data ................................................................................................. 43 4.1.3 OTC data simulation model using NRDM data ...................................... 43 4.2 Linear outbreak simulator .................................................................................. 49 4.3 Multivariate spatial-temporal event simulator ................................................... 50 4.3.1 Parameters ............................................................................................... 51 4.3.2 The model ................................................................................................ 52 5.0 Rank-based tempo-spatial clustering (RSC) .......................................................... 55 5.1 An algorithm for early outbreak detection—rank-based tempo-spatial clustering (RSC) ............................................................................................................................ 55 5.1.1 Risk rate assessment ................................................................................ 56 5.1.2 Adjacency criterion ................................................................................. 59 5.1.3 Searching for clusters .............................................................................. 60 5.1.4 Priors ....................................................................................................... 61 5.1.5 Computing posterior probability of a cluster .......................................... 62 5.1.6 The temporal window.............................................................................. 63 5.1.7 Experiments ............................................................................................. 64 5.1.8 Discussion ............................................................................................... 72 5.2 Grid-based RSC (G-RSC) .................................................................................. 74 5.2.1 Experiments ............................................................................................. 75 5.2.2 Experimental results ................................................................................ 76 5.2.3 Discussion ............................................................................................... 77 6.0 The multivariate rank-based clustering (MRSC) ................................................... 80 6.1 Computing likelihoods using the Multinomial-generalized-Dirichlet (MGD) model ............................................................................................................................ 84 6.2 Bayesian
Details
-
File Typepdf
-
Upload Time-
-
Content LanguagesEnglish
-
Upload UserAnonymous/Not logged-in
-
File Pages161 Page
-
File Size-