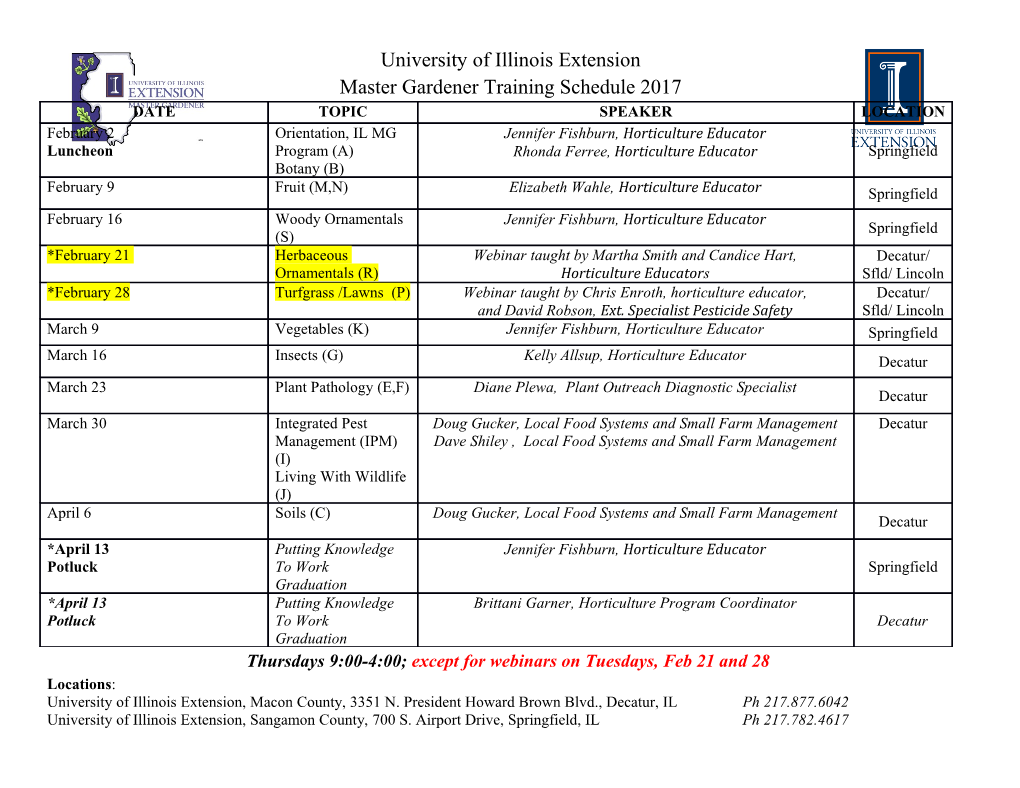
Curso 2012/13 CIENCIAS Y TECNOLOGÍAS/23 I.S.B.N.: 978-84-15910-90-9 SANTIAGO BASALDÚA LEMARCHAND Monte-Carlo tree search using expert knowledge: an application to computer go and human genetics Directores J. MARCOS MORENO VEGA CARLOS A. FLORES INFANTE SOPORTES AUDIOVISUALES E INFORMÁTICOS Serie Tesis Doctorales ciencias 23 (Santiago Basaldúa Lemarchand).indd 1 18/02/2014 11:24:43 Universidad de La Laguna Abstract Monte-Carlo Tree Search Using Expert Knowledge: An Application to Computer Go and Human Genetics During the years in which the research described in this PhD dissertation was done, Monte-Carlo Tree Search has become the preeminent algorithm in many AI and computer science fields. This dissertation analyzes how expert knowledge and also online learned knowledge can be used to enhance the search. The work describes two different implementations: as a two player search in computer go and as an optimization method in human genetics. It is established that in large problems MCTS has to be combined with domain specific or online learned knowledge to improve its strength. This work analyzes different successful ideas about how to do it, the resulting findings and their implications, hence improving our insight of MCTS. The main contributions to the field are: an analytical mathematical model improving the understanding of simulations, a problem definition and a framework including code and data to compare algorithms in human genetics and three successful implementations: in the field of 19x19 go openings named M-eval, in the field of learning playouts and in the field of genetic etiology. Also, an open source integer representation of proportions as Win/Loss States (WLS), a negative result in the field of playouts, an unexpected finding of a possible problem in optimization and further insight on the limitations of MCTS are worth mentioning. With the exception of some background materials introducing the game of go and MCTS, the dissertation is entirely written in English. Incorporación de conocimiento experto a la búsqueda en árbol mediante procesos estocásticos y su aplicación en el juego abstracto del go y en genética humana Durante el periodo de desarrollo de esta tesis Monte-Carlo Tree Search (MCTS la búsqueda en árbol mediante procesos estocásticos) se ha convertido en el algoritmo principal en muchos problemas de inteligencia artificial e informática. Esta tesis analiza la incorporación de conocimiento experto para mejorar la búsqueda. El trabajo describe dos aplicaciones: una en el juego del go por ordenador y otra en el campo de la genética humana. Es un hecho establecido que, en problemas complejos, MCTS requiere del apoyo de conocimiento específico o aprendido online para mejorar su rendimiento. Lo que este trabajo analiza son diferentes ideas de cómo hacerlo, sus resultados e implicaciones, mejorando así nuestra comprensión de MCTS. Las principales contribuciones al área son: un modelo analítico de las simulaciones que mejora la comprensión del papel de las simulaciones, un marco competitivo incluyendo código y datos para comparar métodos en etiología genética y tres aplicaciones con éxito: una en el campo de las aperturas en go de 19x19 llamada M-eval, otra sobre simulaciones que aprenden y una en etiología genética. Además, merece la pena destacar: un modelo para representar proporciones mediante estados llamado WLS con software libre, un resultado negativo sobre una idea para las simulaciones, el descubrimiento inesperado de un posible problema utilizando MCTS en optimización y un análisis original de las limitaciones. Con la excepción de unos materiales introductorios sobre el juego del go y Monte-Carlo Tree Search, la tesis está completamente escrita en inglés. by Santiago Basaldúa Lemarchand TABLE OF CONTENTS CHAPTER 1. INTRODUCTION ................................................................................................................. 2 1.1 THESIS OVERVIEW FOR ENGLISH READERS ..................................................................................... 2 1.2 DESCRIPCIÓN DE LA TESIS EN ESPAÑOL ........................................................................................... 3 CHAPTER 2. BACKGROUND (IN SPANISH) .......................................................................................... 5 2.1 INTRODUCCIÓN AL JUEGO DEL GO ................................................................................................... 5 2.1.1 Historia del juego del go ............................................................................................................ 5 2.1.2 Las reglas básicas del go ........................................................................................................... 7 2.1.3 Evaluación de la habilidad del los jugadores ............................................................................12 2.1.4 El papel del conocimiento en go ................................................................................................13 2.2 EL GO COMO PROBLEMA COMPUTACIONAL ....................................................................................14 2.3 INTRODUCCIÓN A MCTS ...............................................................................................................17 2.3.1 UCB y el dilema de exploración/explotación .............................................................................17 2.3.2 Los métodos Monte-Carlo ingenuos ..........................................................................................18 2.3.3 UCT es UCB aplicado en un árbol a una evaluación estocástica ...............................................18 2.3.4 Ejemplo: UCT aplicado al SPP .................................................................................................20 2.3.5 MCTS .......................................................................................................................................25 2.4 APLICACIÓN DE MCTS EN GENÉTICA HUMANA ..............................................................................26 2.4.1 Breve descripción del problema ................................................................................................26 2.4.2 El algoritmo de clasificación utilizado ......................................................................................27 2.4.3 Las medidas de asociación comúnmente empleadas ..................................................................27 2.4.4 Los experimentos realizados......................................................................................................28 2.4.5 Los resultados obtenidos ...........................................................................................................29 CHAPTER 3. APPLYING KNOWLEDGE IN THE TREE SEARCH .....................................................30 3.1 SUCCESSFUL IDEAS USED IN OTHER PROGRAMS .............................................................................30 3.1.1 Biasing moves ...........................................................................................................................31 3.1.2 RAVE ........................................................................................................................................31 3.1.3 Progressive widening ................................................................................................................32 3.1.4 Parallelization of MCTS ............................................................................................................34 3.2 INCLUDING A PRIORI INFORMATION IN THE TREE............................................................................35 3.2.1 Mathematical models described to deal with a priori information ..............................................36 3.2.2 A priori information and tree search as implemented in GoKnot ...............................................38 3.3 CURRENT LIMITS OF MCTS ...........................................................................................................39 3.3.1 Limits in terms of computer science ...........................................................................................39 3.3.2 Limits in go terms......................................................................................................................40 3.3.3 The opening as a limitation .......................................................................................................41 3.3.4 Divide and conquer ...................................................................................................................42 3.4 M-EVAL: A MULTIVARIATE FULL BOARD EVALUATION FUNCTION .................................................44 3.4.1 Positional judgment in go ..........................................................................................................44 3.4.2 M-eval rationale .......................................................................................................................45 3.4.3 NMF: Non-negative matrix factorization ...................................................................................46 3.4.4 Qualities implemented in M-eval ...............................................................................................47 3.4.5 M-eval search ...........................................................................................................................49 i + 3.4.6 Offline learning of H pd ............................................................................................................50 3.4.7 Online learning of L'd1 .............................................................................................................52
Details
-
File Typepdf
-
Upload Time-
-
Content LanguagesEnglish
-
Upload UserAnonymous/Not logged-in
-
File Pages175 Page
-
File Size-