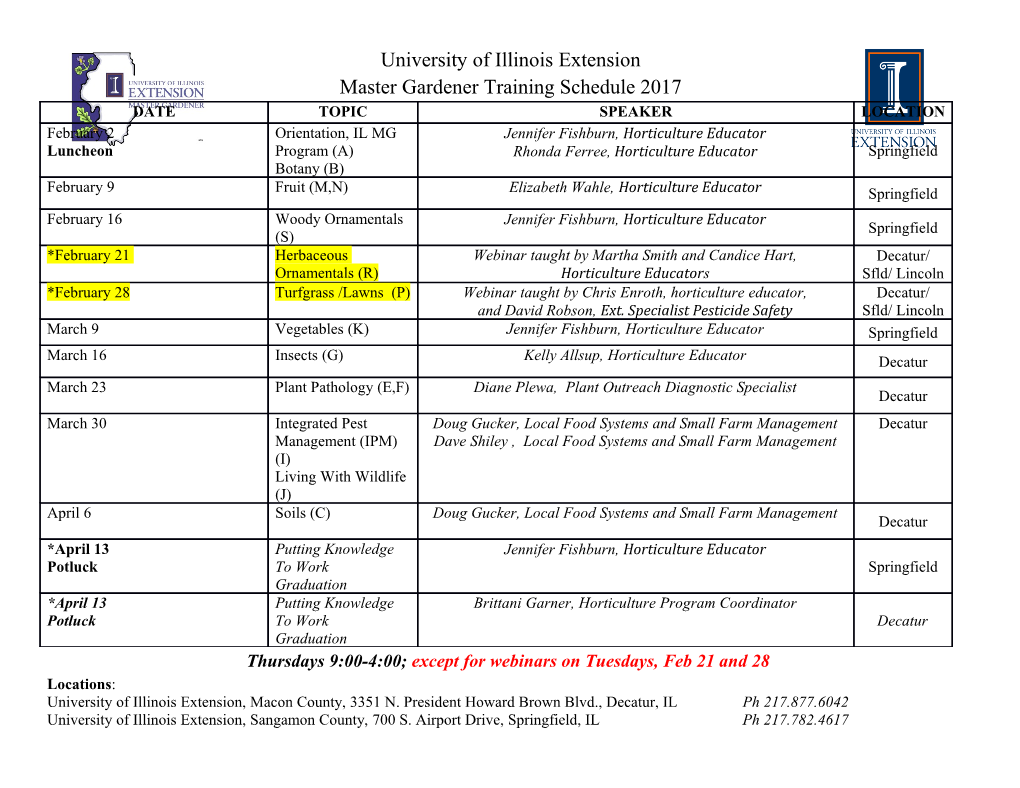
A Subspace Identification Extension to the Phase Correlation Method William Scott Hoge, Member, IEEE Abstract— The phase correlation method is known to pro- where (k; l) are the Fourier domain coordinates, and a and vide straightforward estimation of rigid translational mo- b are the magnitude of the horizontal and vertical shifts tion between two images. It is often claimed that the origi- nal method is best suited to identify integer pixel displace- that occur between A and B. ments, which has prompted the development of numerous To identify a and b using the PCM approach, one com- subpixel displacement identification methods. However, the putes a normalized cross power spectrum between A and fact that the phase correlation matrix is rank one for a noise- B to identify the phase correlation matrix: free rigid translation model is often overlooked. This prop- erty leads to the low complexity subspace identification tech- B(k; l)A(k; l)¤ nique presented here. The combination of non-integer pixel Q(k; l) = = expf¡j(ka + lb)g: (2) displacement identification without interpolation, robust- jA(k; l)A(k; l)¤j ness to noise, and limited computational complexity make this approach a very attractive extension of the phase cor- Once computed, the approach cited in the literature [5,6] is relation method. In addition, this approach is shown to be to compute the inverse Fourier transform of Q. If the two complementary with other subpixel phase correlation based functions under comparison were in fact continuous, then techniques. this representation would be a delta function, Keywords— phase correlation method, SVD, subpixel im- age registration Q(x; y) = ±(x ¡ a; y ¡ b); (3) where the function peak identifies the magnitude of the I. Introduction lateral shift. The ability to detect and estimate lateral shifts between However, in the case of images (which are 2D functions similar images is an integral part of many image processing sampled on a discrete grid), Q will only display a delta-like applications. For example, displacement estimation is often function if a and b are integers. Non-integer translations needed in medical imaging to compensate for inter-image between two similar images cause the peak in Q(x; y) to motion of a patient during imaging sessions and for regis- spread across neighboring pixels, subsequently degrading tration of features in image studies of multiple patients. In the quality of the translation estimate. Furthermore, if response to this need, many methods have been developed the images are not spatially band-limited when sampled, to estimate the translational bulk displacement between aliasing will occur and (1) may not be strictly valid for all similar images. The phase correlation method (PCM) [1] (k; l). The sharpness and clarity of the peak can also be is a popular choice due to its robust performance and com- degraded by image edge effects as discussed in Sec. II-B. putational simplicity. To identify non-integer shifts in the spatial domain, the While the PCM technique is applicable to images ac- common approach is to apply bilinear, Lagrange, or other quired via any modality, the emphasis here is on im- interpolation methods. Alternatively, one can work di- ages acquired via magnetic resonance imaging (MRI). This rectly in the Fourier domain to identify the subpixel shift. method is a natural fit with MRI because the acquired im- Two methods that follow this approach were recently de- age data is typically sampled in the spatial Fourier domain scribed in [4] and [7]. While focused on the effects of alias- [2,3]. Thus, image registration using phase correlation can ing on the shift estimation, the translation parameter es- be applied directly to the raw MRI data before the spatial timation in [7] is performed using a least-squares fit to a images are reconstructed. two dimensional data set — which the authors of [4] claim is difficult because it requires fitting a plane to noisy phase The phase correlation method is based on the well-known difference data. As shown below, the dimensionality of the Fourier shift property. Specifically, a shift in the coordinate least squares fit can be reduced through a subspace iden- frame of two functions results in a linear phase difference tification of the phase correlation matrix itself. Thus, the in the Fourier transform of the two functions [4]. Given method described below is complementary to both of the a pair of two dimensional functions, A and B, that are methods cited above. related by a simple translational shift, the elements of the Fourier transform of B, denoted B, are related to A by II. Strategy A close inspection of (2) reveals that the noise-free model B(k; l) = A(k; l)expf¡j(ka + lb)g (1) for Q is in fact a rank one matrix. Each element in Q can be separated as Q(k; l) = expf¡jkagexpf¡jlbg. This allows This work was supported under a US NIH T32 Grant (PA-00-103). Please direct all inquires to: W. Scott Hoge, Ph.D. ([email protected]) the definition of two vectors, Harvard Medical School and Brigham and Women’s Hospital, 75 Francis Street, Boston, MA 02115 USA qa(k) = expf¡jkag and qb(l) = expf+jlbg: (4) (c) 2003 IEEE. IEEE Trans. Medical Imaging, 22(2):277-280, Feb 2003 ACCEPTED FOR PUBLICATION BY IEEE TRANS. MEDICAL IMAGING 2 and the phase correlation matrix can be rewritten as Q = sampling system is aliased to lower frequencies. This fact H qaqb . This allows one to rewrite (1) in matrix notation as was the driving motivation for the subpixel identification method described in [7,8]. H B = (qaqb ) ± A (5) Medical images are not immune to aliasing, although the spatial-frequency aliasing found in optical systems is not a where f¢gH denotes a complex-conjugate transpose, and concern in well-formed MR images. This is because MRI ± indicates an element-by-element product, also known as data acquisition typically samples the spatial Fourier spec- the Schur or Hadamard product [9]. trum of the field of view (FOV) directly. Along the fre- A. The Algorithm quency encoding direction, spatial spectrum energy at fre- quencies above the maximum sampled are truncated, with The illuminating feature of (5) is that the problem of the visible result of Gibbs ringing in the reconstructed im- finding the exact lateral shift between two images is recast age if the truncation occurs at a sufficiently low spatial as finding the rank one approximation of the normalized frequency [3]. Aliasing can occur in MRI when the spacing phase correlation matrix, Q. A straight-forward approach between phase encode lines is not dense enough to com- to finding the dominant rank one subspace of Q is to use the pletely cover the region of excited spins. The immediately singular value decomposition (SVD) [9]. The linear phase noticeable effect is multiple copies of the aliased spatial coefficients can then be identified independently in the left components in the reconstructed image — a very different and right dominant singular vectors. From these, estimates result than aliasing in optical systems. of the vertical and horizontal shift can be derived, even for Nonetheless, the SIE-PCM algorithm is completely com- non-integer translational motion over a large range. plementary to the aliasing compensation approaches de- To identify the linear phase coefficients in each of the scribed in [7]. Stone, et. al., recommend masking the right and left dominant singular vectors of Q, a least- phase-correlation matrix, Q, to restrict the spectrum com- squares fit (LSF) to the unwrapped phase component of ponents corrupted by aliasing from the shift estimation. the dominant singular vectors is used. For a matrix A of This mask captures the components of A with magnitude size M £ N, k = 2¼x=M and l = 2¼y=N. For a given sin- larger than a given threshold ® that are present within a gular vector, v, we construct the set of normal equations radius r = 0:6(L=2) of the spectrum origin. Here, L is the R [ ¹ c ]T = unwrapf6 vg where the rows of R are equal minimum number of samples in the vertical and horizontal to [ r 1 ] for r = f0; 1; 2; ¢ ¢ ¢ ; (s ¡ 1)g, with s equal to the dimensions. This masking approach can be applied to the length of v. Here, ¹ and c are the slope and abscissa of the SIE-PCM method, where only those components in each fitted line, respectively. This system is then solved to give vector within a prescribed distance from the D.C. compo- · ¸ ¹ nent is utilized in the linear phase angle determination. = (RT R)¡1RT unwrapf6 vg: (6) c Alternatively, one could potentially mask the phase corre- lation matrix first, and then use the SVD approach on the The slope of the fitted line, ¹, maps to the translational sparse matrix to identify the shift parameters. shift. Specifically, a = ¹(M=2¼) for the case v = qa, and Additionally, image features close to the image edge can b = ¹(N=2¼) for the case v = qb. have a negative effect on the ability to identify translational The quality of the linear fit depends on the linearity motion between two images. The discrete Fourier trans- of the unwrapped phase vector. In practice, the implicit form (DFT) imposes a cyclic repetition on finite length eigen-filtering nature of identifying the dominant singular signals. For images, these edge effects imply that pixels vectors of Q provides the unwrapping algorithm with less- on the right (top) will be appended to the left (bottom) noisy data. Furthermore, because the unwrapping need in an infinite cyclic pattern when constructing the DFT of only be done along one dimension, it is inherently less com- the image, [10,11].
Details
-
File Typepdf
-
Upload Time-
-
Content LanguagesEnglish
-
Upload UserAnonymous/Not logged-in
-
File Pages4 Page
-
File Size-