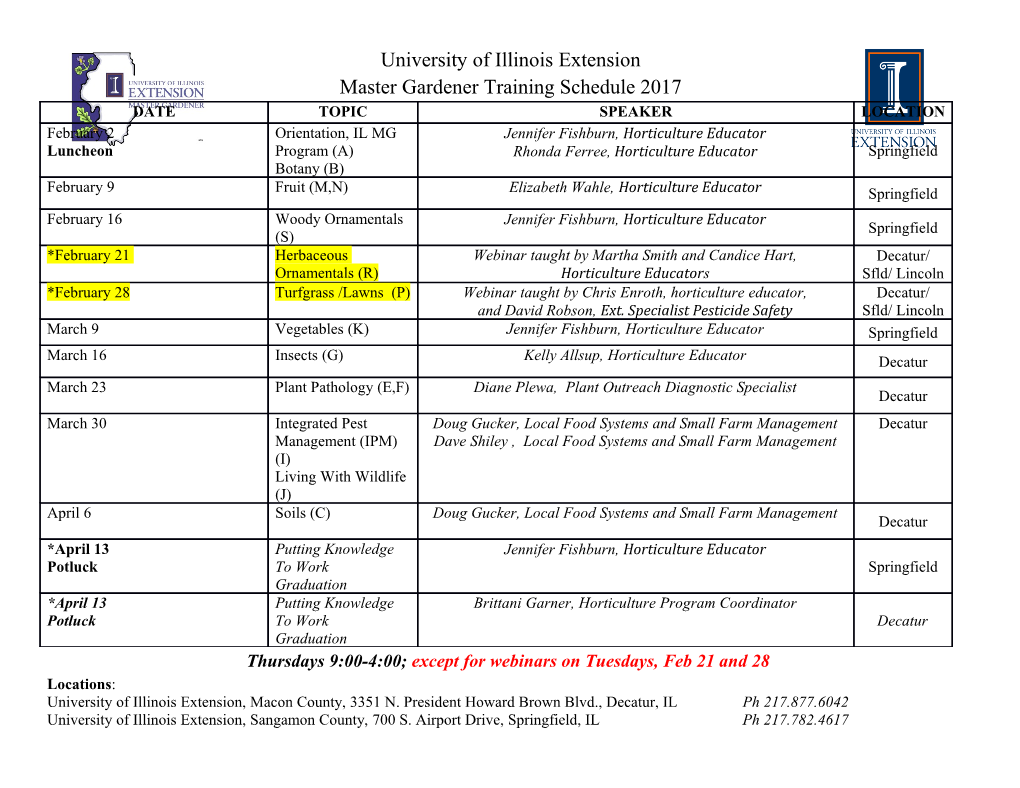
Network Anomaly Detection Using LightGBM: A Gradient Boosting Classifier Md. Khairul Islam1, Prithula Hridi1, Md. Shohrab Hossain1, Husnu S. Narman2 1Department of Computer Science and Engineering, Bangladesh University of Engineering and Technology, Bangladesh 2Weisberg Division of Computer Science, Marshall University, Huntington, WV, USA Email: [email protected], [email protected], [email protected], [email protected] Abstract—Anomaly detection systems are significant in rec- U2R and the R2U attacks, the DoS attack to the collective ognizing intruders or suspicious activities by detecting unseen anomaly, and the Probe attack to the contextual anomaly. and unknown attacks. In this paper, we have worked on a Machine learning techniques, in many cases they have benchmark network anomaly detection dataset UNSW-NB15, that reflects modern-day network traffic. Previous works on this outperformed the previous state-of-the-art models. As UNSW- dataset either lacked a proper validation approach or followed NB15 [4] is a benchmark network anomaly detection dataset, only one evaluation setup which made it difficult to compare numerous studies have been done on it. However, to evaluate their contributions with others using the same dataset but with the same dataset many different setups were adopted (Section different validation steps. In this paper, we have used a machine II). For example, train and evaluate on train data [5], [6] learning classifier LightGBM to perform binary classification on this dataset. We have presented a thorough study of the dataset . Ten-fold cross-validation on train data [7], [8]), test data with feature engineering, preprocessing, feature selection. We [9], combined (train+test) data [10], [11]. Five-fold cross- have evaluated the performance of our model using different validation on train and test data [12] . Train on train data experimental setups (used in several previous works) to clearly and evaluate on test data [13], [14]. evaluate and compare with others. Using ten-fold cross-validation With so many different experimental setups, it is difficult on the train, test, and combined (training and test) dataset, to find the single best work on this dataset. Moreover, works our model has achieved 97.21%, 98.33%, and 96.21% f1 scores, respectively. Also, the model fitted only on train data, achieved that followed the same experimentation setup did not compare 92.96% f1 score on the separate test data. So our model their results with prior works in some cases (for example, also provides significant performance on unseen data. We have Kanimozhi et al. [6] and Nawir et al. [10] did not compare their presented complete comparisons with the prior arts using all results with Koroniotis et al. [11]). Therefore, it is difficult performance metrics available on them. And we have also shown to validate improvements. Mogal et al. [5] and Kanimozhi et that our model outperformed them in most metrics and thus can detect network anomalies better. al. [6] mentioned near-perfect detection scores. However, they Index Terms—anomaly detection, machine learning, network did not mention the significant technical flaws regarding their security. approaches, which we have explained in Section IV-B. Some other works [6], [10], [12] followed only one validation setup. I. INTRODUCTION Hence, it is impossible to compare those works with the ones, which have worked on the same dataset but with different Web applications are getting increasingly popular, and the validation setups. Internet has become an important part in our daily life. As The novelty and contributions of this work are as follows: a consequence, network systems are being targeted more by • We have provided a thorough study of the UNSW-NB15 attackers [1] with malicious intent [2]. To detect intruders in a dataset with feature engineering, preprocessing, selection. network system, there are generally two approaches: signature- • We have explored the performance of a boosting algo- based and anomaly-based detection. Signature-based systems rithm in binary classification on the dataset following all maintain a database of previously known attacks and raise experimentation setups found from prior studies whereas alarms when any match is found with the analyzed data. each of the previous works focused on only one setup. However, they are vulnerable to zero-day attacks. • We have compared our results to prior state-of-the-art An anomaly in a network means a deviation of traffic data works using all related performance metrics. from its normal pattern. Thus, anomaly detection techniques Our results show that feature engineering can make the have the advantage of detecting zero-day attacks. However, in model more generalized. So our model performance improved a complex and large network system, it is not easy to define in cross-validation experiments, as well as when evaluated on a set of valid requests or normal behavior of the endpoints. separate test data. We have also shown a very small false Hence, anomaly detection faces the disadvantage of having a alarm rate (1.83% - 4.81%). Our work can help in detecting high false-positive error rate. unseen anomaly attacks better having very few false alarms. According to Ahmed et al. [3], the main attack types are And Our different experimentation setups will help visualize DoS, Probe, User to Root (U2R), and Remote to User (R2U) the impact of validation strategies on the model performance attacks. Ahmed et al. [3] mapped the point anomaly with the of this dataset. The rest of the paper has been organized as follows. by using the WEKA tool. They also compared centralized and SectionII describes the recent works related to NIDS (Network distributed AODE algorithms based on accuracy against the Intrusion Detection Systems) on the UNSW-NB15 dataset. number of nodes. Our proposed methodology has been explained in Section Meftah et al. [8] applied both binary and multiclass clas- III. Section IV describes the experimentation setups and our sification on the UNSW-NB15 dataset. They found that for results as well as some comparisons with the prior state-of-the- binary classification SVM performs the best in ten-fold cross- art. The rest of the comparisons regarding evaluating on train validation and decision tree (C5.0) for multiclass classification. and test data, cross-validation approaches have been shown in Hanif et al. [9] used ANN(Artificial Neural Network) on the Section V. Finally, SectionVI has the concluding remarks. same dataset. They compared their performance with prior works on the NSL-KDD dataset, instead of works on the same II. RELATED WORKS dataset. Meghdouri et al. [12] applied feature preprocessing For network intrusion detection KDDCUP99, NSL-KDD, and principal component analysis on the UNSW-NB15 dataset. DARPA, UNSW-NB15 are among the benchmark dataset. As Then performed five-fold cross-validation using a RandomFor- a popular dataset, we focus on binary classification of the est classifier. UNSW-NB15 dataset [4] which is used in several anomaly detection works. Based on the model evaluation process, we D. Validation on separate test data have divided them into several parts. Moustafa et al. [14] analyzed the statistical properties of the UNSW-NB15 dataset and showed that it is more complex A. Random train test compared to the KDD99 dataset. Vinaykumar et al. [18] Moustafa et al. [15] used central points of attribute values used classical machine learning classifiers, and deep neural and Association Rule Mining for feature selection on a high networks on several intrusion detection datasets. The classical level of abstraction from datasets UNSW-NB15 and NSL- models performed much better than the neural network mod- KDD. They have partitioned the datasets into train and test els. Dahiya et al. [19] applied feature reduction techniques on sets following an equation. Then evaluated performance us- both larger and smaller versions of the UNSW-NB15 dataset. ing Expectation-Maximisation clustering (EM), Logistic Re- Bhamare et al. [13] tested the robustness of machine learning gression (LR), and Nave Bayes (NB). Moustafa et al. [16] models in cloud scenarios. They trained classifiers on the also proposed a beta mixture model-based anomaly detection UNSW-NB15 dataset and tested them on a cloud security system on the UNSW-NB15 dataset. They first selected eight dataset ISOT. They found that these models did not perform features from the dataset, then randomly selected samples from well in the cloud environment. it. In another work, Moustafa et al. [17] selected random samples from the UNSW-NB15 dataset and ran ten-fold cross- E. Gap analysis validation on it. To the best of our knowledge, there has been no work that has provided a thorough study of the UNSW-NB15 dataset B. Validation on same data used for training with feature engineering to improve results. Moreover, most Mogal et al. [5] used machine learning classifiers on both of the previous works on this dataset focused on only one UNSW-NB15 and KDDCUP99 datasets. They achieved nearly evaluation process. So it is difficult to make a proper com- 100% accuracy on both datasets using Naive Bayes and parison among them. For example, the performance achieved Logistic Regression on train data. Kanimozhi et al. [6] choose in five-fold cross-validation, can not be compared with that the best four features of the UNSW-NB15 dataset using achieved in ten-fold cross-validation. So, we have evaluated the RandomForest classifier. They also used a Multi-Layer our model performance using all possible experimentation Perceptron to show how neural networks would perform on setups found in prior arts and provided a thorough comparison this dataset. with prior state-of-the-art techniques. We have also used feature engineering to reduce overfitting, thereby providing a C. Cross validation more generalized model. Koroniotis et al. [11] selected the top ten features of the UNSW-NB15 combined (train+test) dataset using Information III.
Details
-
File Typepdf
-
Upload Time-
-
Content LanguagesEnglish
-
Upload UserAnonymous/Not logged-in
-
File Pages7 Page
-
File Size-