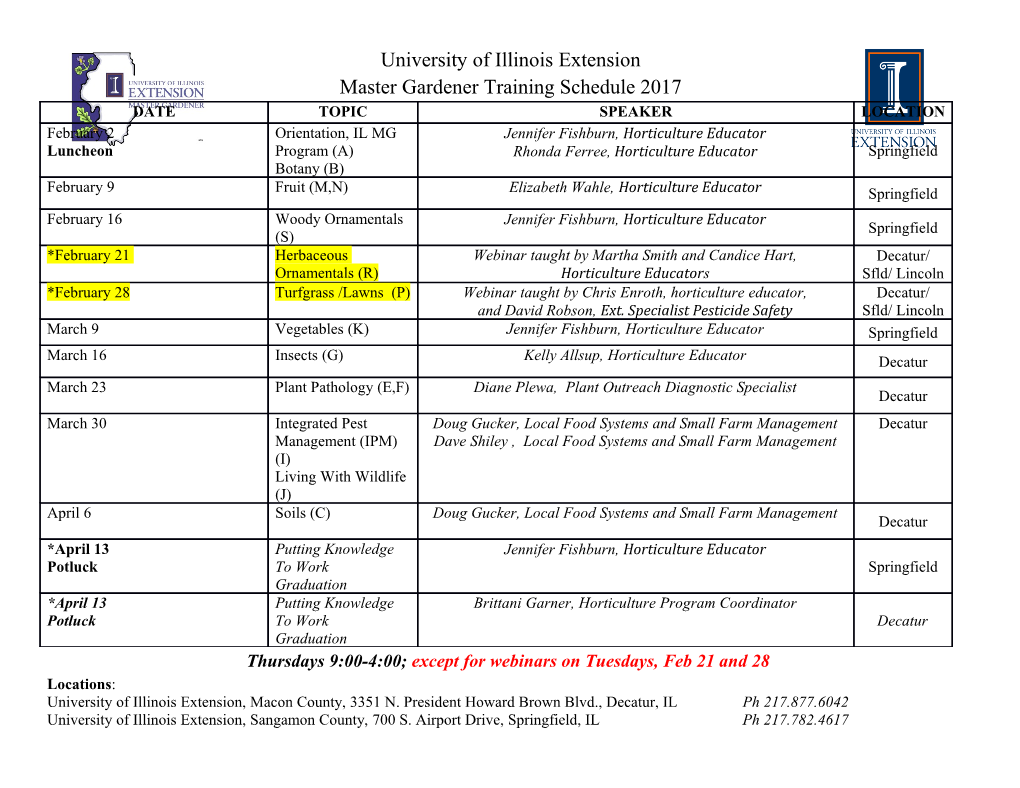
47 Journal of MultiDisciplinary Evaluation The Book of Why: The New Volume 14, Issue 31, 2018 Science of Cause and Effect Pearl, J., & Mackenzie, D. (2018). New York, NY: ISSN 1556-8180 Basic Books. http://www.jmde.com Steve Powell ProMENTE Social Research, Sarajevo This is a review of “The Book of Why”, (Pearl & The central premise of the book is that we have Mackenzie, 2018), the first book for a general been lacking almost any well-established, formal readership by Turing Prize winner Judea Pearl, one way to talk about causality – to express causal of the parents both of Artificial Intelligence and of questions or answers, to write down the equation the “Causal Revolution” in statistics. The present for “smoking causes cancer” or the question “what review is more extensive than most book reviews was the causal contribution of this intervention to because of the fundamental significance of this that effect?” The place most empirical social book and its subject matter to the evaluation scientists have turned to in the search for such a community. language is statistics. But for nearly a century, Causality matters to evaluators. We need to discourse around causality was dominated by the understand how elements of a project or program thousand-pound gorillas of classical, associationist are supposed to work: how interventions made on statistics, originally in the persons of R.A. Fisher some things might contribute to differences made and Karl Pearson, who told us that firstly to other things, which themselves have further correlation is not causation, and secondly that there consequences. As such, we as evaluators are is no such thing as evidence for causation except in concerned with causality. Also when we try to judge the case of a Randomised Controlled Trial (RCT), in retrospect what in fact contributed to what, we and thirdly that even when we have an RCT there is are dealing with causality. no way to explain what happened to individuals To be sure, most evaluators have abandoned, or within that very trial; explanations were restricted would like to have the freedom to abandon, overly- to the collective. Consequently, we were told that in mechanistic and linear models of how interventions cases where no trial exists there can be no causal work. The theories of change we (would like to) deal explanations, and where no trial can ever be with are perhaps structured more like diagrams of conducted (perhaps for ethical reasons) there will organisms or ecosystems than like diagrams of never be any causal explanations. So, essentially, machines, and they may have non-linear, partial, except in the rare and special case of reporting the probabilistic connections with feedback loops, and result of a randomised controlled trial, the word may have vague and/or missing information. But “cause” was taboo. they are networks of putative causal connections all Some fringe disciplines did flourish at the edge the same. Even when someone says, “this is a of the jungle away from these thousand-pound complex system, it is very hard to predict its gorillas, giving us fragments of languages to talk behavior, we need to identify key leverage points to about causation - Fuzzy Sets and Fuzzy Causal influence its development in such-and-such a way”, Maps (Kosko, 1986; Zadeh, 1973), Contribution they are still expressing a causal theory, albeit a Analysis (Mayne J, 2001), Process Tracing – see fuzzy one, that tweaking this might influence that. Collier (2011) – amongst others. But no alternative So, the concept of causality in one form or another paradigm was strong enough to unseat the is essential to evaluation. 48 Powell associationist gorillas. Evaluation and the social anything about the direction or underlying nature sciences suffered as a result. of this connection - and Pearl and his colleagues In practice, of course, we pose causal questions had no way to store this information in an and give causal explanations all the time, very often artificially intelligent system. But, he reasoned, if on an ad-hoc basis. Children learn to do it in their children can learn to understand and store causal very first years (even though they never conduct information, machines can too. randomised controlled trials), just as they learn to Pearl went back to the work of the geneticist use mathematical language. In later years, they will Sewall Wright who invented Path Analysis as a way learn how to formalise mathematical questions and to encode causal information using diagrams in the calculate their answers using special symbols such early 1920s (Wright, 1921). These causal diagrams as the “+” symbol for addition. But until recently, represent connections prior to the probabilistic no-one was taught how to formalise causal information we actually observe. They explain and questions or how to calculate or express the go beyond our observations. answers. Wright’s work was vigorously and explicitly Now all that is changing. A causal revolution is discouraged by the thousand-pound gorillas of shaking the jungle, thanks primarily to the work of Pearson and Fisher. They stuck almost religiously statistician Donald Rubin and, above all, to to the positivistic ideology that all knowledge is philosopher-statistician Judea Pearl. This is not a sensory information, and sensory information can fringe skirmish or a dissenting footnote but a root- not encode causal connections. and-branch rewriting of how we formulate and The authors also refer to the work of Barbara gather evidence for causal statements, which is Burks (1926), who may have preceded Wright with being felt in disciplines from epidemiology to the use of causal diagrams in particular in the study climate science. of mediation, but the uptake of her work suffered Most evaluators are not aware of this under the twin pressures of mainstream statistics revolution. The present review attempts to and the prejudices against women in science in the contribute to spreading the good news to the early and mid-twentieth century. evaluation community. Causal diagrams are easy to understand and are not so different in principle from the logic models Judea Pearl and his Contribution and theories of change, with all their various weaknesses, which evaluators and program staff use all the time. Essentially, a causal arrow from Judea Pearl started his career as an engineer. In the “fire” to “smoke” says “intervening on the variable 1980s he was working on problems in artificial ‘fire’ will do something to the variable ‘smoke’”. As intelligence. He wanted to be able to teach an a by-product, this intervention may also make artificially intelligent system to navigate and “smoke” more probable; but this depends also on interact with the real world. He invented Bayesian other circumstances (other variables affecting Networks in order to facilitate the processing of “smoke”). The arrow in a Pearlian diagram is about probabilistic rather than only deterministic causal contribution, not about probability: it information (some of his work powers the phone in explains the probabilities. It remains a stable and your pocket, along with many other innovations). valid piece of knowledge even if in a particular More specifically, although the idea of using instance someone sucks away the smoke with a probabilistic information was not new, in practice, vacuum cleaner. probabilistic knowledge was encoded in huge By around 2000, Pearl had succeeded in probability tables in which the association of every providing a formal way to store causal information variable with every other was stored, and these and solve causal problems using a combination of required just too much computing power to work diagrams and mathematical expressions. This work with. Pearl’s contribution was to show how to use is summarised in his outstanding contribution network diagrams to break down these tables into (Pearl, 2000). Unfortunately, even a reader with much more manageable units. some familiarity with graph theory, statistics and But Pearl was still frustrated because these formal logic will probably find it a difficult read. Bayesian Networks could not encode causal Pearl’s web page does list some public information. They could not explain why making presentations which are more accessible, but only the cock crow does not cause the sun to rise, even with the publication of “The Book of Why” in 2018 though the two events are highly correlated: if one does the ordinary reader finally have access to some occurs, the other is highly probable. Classical of Pearl’s most consequential ideas. statistics does not give us any convincing way to say Journal of MultiDisciplinary Evaluation 49 What Pearl and his co-workers have done is to representing explicitly causal, not correlational, break the taboo imposed by classical statistics on links. explicitly causal language, whether graphical or Each link in a diagram corresponds to a written written, in mainstream empirical social science. expression of the form What is in the Book? smoke = f(fire, ...) in which the value of one variable is expressed as a Pearl presents a ladder of knowledge with three (mathematical) function of one or more other levels: Association, Causation and Counterfactuals, variables. It is important to note that these are not differentiated in terms of the kind of statements equations in the normal sense, in particular which can be asked and answered at each level. The because it is not usually possible to reverse them: chapters of the book are loosely structured around the sentence above says that smoke is causally these levels. dependent on fire, and not vice versa. Some evaluators might be about to stop reading Level One (Association) at this point because it might seem that this approach is only useful for things which can be Classical and Bayesian statistics are situated on this measured precisely in numbers.
Details
-
File Typepdf
-
Upload Time-
-
Content LanguagesEnglish
-
Upload UserAnonymous/Not logged-in
-
File Pages8 Page
-
File Size-