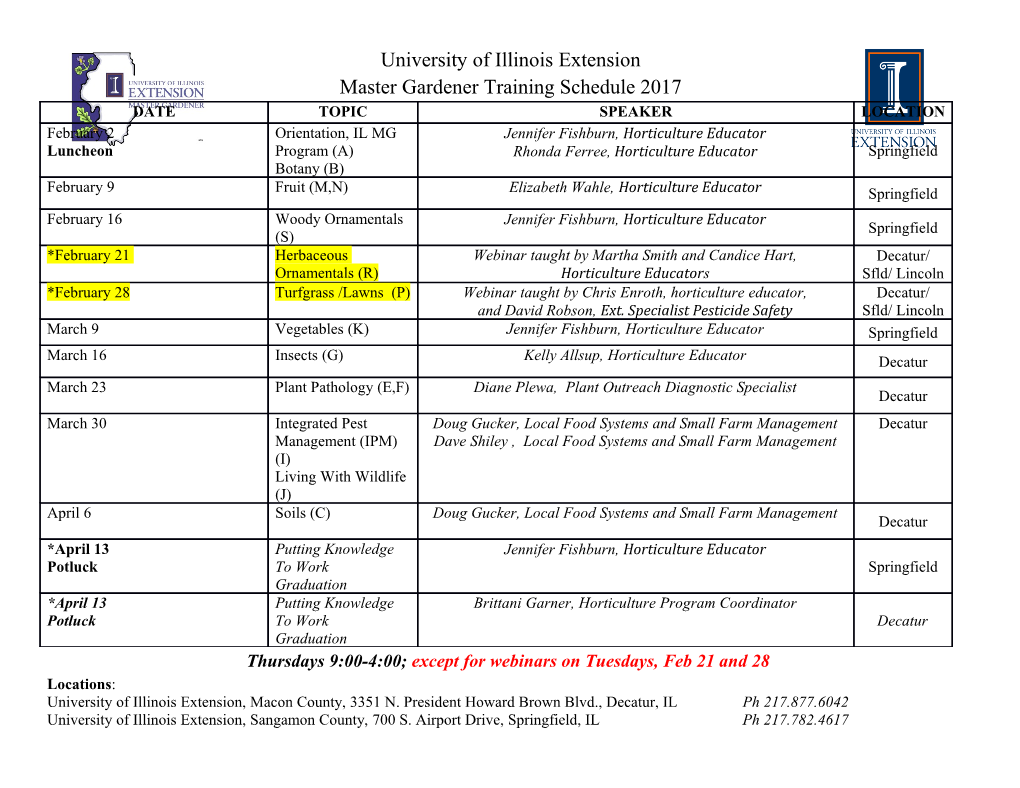
AMS 311 Joe Mitchell Notes on Expectation, Moment Generating Functions, Variance, Covariance For a random variable X, the expected value of X (or the mean or the average of X) is given by x∈A x · p(x) if X is discrete E(X)= ∞ ( P−∞ xf(x)dx if X is continuous We can also compute expectationsR of functions of the random variable X, using the “Law of the Unconscious Statistician”: x∈A g(x) · p(x) if X is discrete E(g(X)) = ∞ ( P−∞ g(x) · f(x)dx if X is continuous WARNING: Do NOT use the LawR of the Unemployed Statistician, which would say that E(g(X)) = g(E(X)). (Example: It is NOT true, in general, that E(X2) = [E(X)]2, since this means that var(X) = 0.) Example: If X ∼ exp(λ), then ∞ E(X3 cos X)= x3 cos x · λe−λxdx Z0 . More generally, for two random variables X and Y , x y g(x, y) · p(x, y) if X, Y are discrete E(g(X,Y )) = ∞ ∞ ( P−∞P−∞ g(x, y) · f(x, y)dydx if X and Y are continuous R R Examples: If X and Y are uniform over the 2-by-2 square centered on the origin, then 1 1 1 E(eX Y 3)= exy3 · dydx − − Z 1 Z 1 4 and 1 1 1 E(X)= x · dydx − − Z 1 Z 1 4 Def. The nth moment of X is E(Xn). tX Def. The moment generating function of X is MX (t)= E(e ), provided that this expec- tation exists (is finite) for values of t in some interval (−δ, δ) that contains t = 0. Moment generating functions are useful for generating the moments of X (hence, the name!): to compute the nth moment of X, we simply take the nth derivative (with respect to t) of MX (t), and then plug in t = 0: ′ 2 ′′ n (n) E(X)= MX (0),E(X )= MX (0),E(X )= MX (0) Example: If X ∼ exp(λ), then ∞ tX tx −λx λ MX (t)= E(e )= e · λe dx = Z0 λ − t (we assume that t<λ, or else the integral blows up to infinity). Thus, we can compute ′ 2 ′′ 3 MX (t) = λ/(λ − t) , MX (t) = (2λ)/(λ − t) . This gives us the first and second moments: ′ 2 2 ′′ 3 2 E(X)= MX (0) = λ/(λ − 0) = 1/λ, and E(X )= MX (0) = (2λ)/(λ − 0) = 2/λ . Facts About Expectations (1). E(aX + b)= aE(X)+ b (2). E(X + Y ) = E(X)+ E(Y ), even if X and Y are NOT independent. More generally, E( i aiXi)= i aiE(Xi). P P Def. The variance of X is var(X)= E((X − µ)2)= E(X2) − µ2, where µ = E(X) is the mean of X. Def. The covariance of X and Y is cov(X,Y )= E((X − E(X))(Y − E(Y ))) = E(XY ) − E(X)E(Y ). Def. The correlation of X and Y is cov(X,Y ) ρ(X,Y )= var(X) · var(Y ) q Facts About Variance and Covariance (1). var(aX + b)= a2 · var(X); in particular, putting a = 0 gives: var(b) = 0, for a constant b. (2). If X and Y are independent, then E[g(X)h(Y )] = E(g(X)) · E(h(Y )). (NOTE: the converse is FALSE – if E[g(X)h(Y )] = E(g(X)) · E(h(Y )), then X and Y need not be independent!) (3). If X and Y are independent, then cov(X,Y ) = 0. (follows immediately from (2), and the definition of covariance) (NOTE: again, if cov(X,Y ) = 0, X and Y need not be independent! For an example, let X = −1, 0, 1, each with probability 1/3. Let Y be 0 if X =6 0, and Y is 1 if X = 0. Then Y certainly depends on X; the rv’s are NOT independent. But you can compute that cov(X,Y ) = 0.) (4). var(X + Y )= var(X)+ var(Y ) + 2cov(X,Y ) var( Xi)= var(Xi) + 2 cov(Xi, Xj) i i i<j X X X X In particular, IF X and Y are independent, then var(X + Y )= var(X)+ var(Y ). 2 (5). −1 ≤ ρ(X,Y ) ≤ 1 (6). cov(a, X) = 0 cov(a + bX, c + dY )= bd · cov(X,Y ) cov(X + Y, Z)= cov(X, Z)+ cov(Y, Z) cov( Xi, Yj)= cov(Xi,Yj) Xi Xj Xi Xj Examples: 1. cov(X, 5X + 3) = 1 · 5 · cov(X, X) = 5 · var(X) 2. 5var(X) ρ(X, 5X +3) = = 1 var(X)var(5X + 3) q 3. Roll 2 dice. Let W1 and W2 be the values shown. Let X = W1 +W2 and Y = W1 −W2. Then, cov(X,Y ) = cov(W1 + W2,W1 − W2)= cov(W1,W1) − cov(W2 − W2)+ cov(W1,W2) − cov(W1,W2) = var(W1) − var(W2) = 0 where we have used the fact that W1 and W2 are independent to conclude that cov(W1,W2)= 0. BUT, note that even though cov(X,Y ) = 0, X and Y are NOT indpendent. (Why? Well, if we know something about X, does this affect the distribution of Y ? YES: If we know that X = 12, then we know that Y = 0.) 4. If X and Y have joint density 1 (x + y) 0 <x< 1, 0 <y< 2 f(x, y)= 3 0 otherwise then we can compute var(2X − 3Y + 8) = 4 · var(X) + 9 · var(Y ) + 2 · 2 · (−3) · cov(X,Y ) 2 2 1 2 1 using var(X)= E(X ) − [E(X)] , cov(X,Y )= E(XY ) − E(X)E(Y ), E(X)= 0 0 x 3 (x + 2 1 2 2 1 1 2 1 2 1 2 2 1 y)dydx, E(X )= 0 0 x 3 (x + y)dydx, E(Y )= 0 0 y 3 (x + y)dydx, E(Y )= R0 R0 y 3 (x + 1 2 1 y)dydx, E(XY )=R 0R 0 xy 3 (x + y)dydx. R R R R R R 3.
Details
-
File Typepdf
-
Upload Time-
-
Content LanguagesEnglish
-
Upload UserAnonymous/Not logged-in
-
File Pages3 Page
-
File Size-