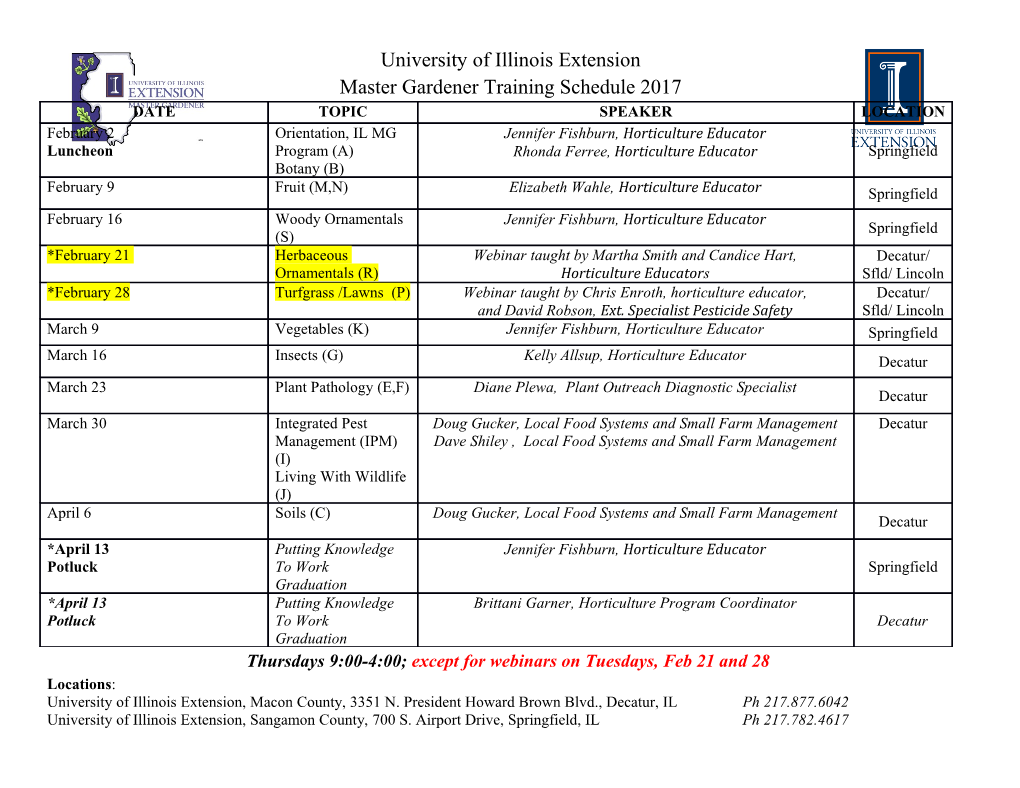
Computer Science Technical Reports Computer Science 1995 A Neural Network Architecture for High-Speed Database Query Processing Chun-Hsien Chen Iowa State University Vasant Honavar Iowa State University Follow this and additional works at: http://lib.dr.iastate.edu/cs_techreports Part of the OS and Networks Commons, Systems Architecture Commons, and the Theory and Algorithms Commons Recommended Citation Chen, Chun-Hsien and Honavar, Vasant, "A Neural Network Architecture for High-Speed Database Query Processing" (1995). Computer Science Technical Reports. 43. http://lib.dr.iastate.edu/cs_techreports/43 This Article is brought to you for free and open access by the Computer Science at Iowa State University Digital Repository. It has been accepted for inclusion in Computer Science Technical Reports by an authorized administrator of Iowa State University Digital Repository. For more information, please contact [email protected]. A Neural Network Architecture for High-Speed Database Query Processing Abstract Artificial neural networks (ANN), due to their inherent parallelism and potential fault tolerance offer an attractive paradigm for robust and efficient implementations of large modern database and knowledge base systems. This paper explores a neural network model for efficient implementation of a database query system. The ppa lication of the proposed model to a high-speed library query system for retrieval of multiple items is based on partial match of the specified query criteria with the stored records. The performance of the ANN realization of the database query module is analyzed and compared with other techniques commonly in current computer systems. The er sults of this analysis suggest that the proposed ANN design offers an attractive approach for the realization of query modules in large database and knowledge base systems, especially for retrieval based on partial matches. Disciplines OS and Networks | Systems Architecture | Theory and Algorithms This article is available at Iowa State University Digital Repository: http://lib.dr.iastate.edu/cs_techreports/43 A Neural Network Architecture for High-Sp eed Database Query Pro cessing Chun-Hsien Chen & Vasant Honavar Department of Computer Science 226 Atanaso Hall, Iowa State University, Ames, IA 50011. U.S.A. Abstract Arti cial neural networks ANN, due to their inherent parallelism and p otential fault tolerance o er an attractive paradigm for robust and ecient implementations of large mo dern database and knowl- edge base systems. This pap er explores a neural network mo del for ecient implementation of a database query system. The applica- tion of the prop osed mo del to a high-sp eed library query system for retrieval of multiple items is based on partial match of the sp eci ed query criteria with the stored records. The p erformance of the ANN realization of the database query mo dule is analyzed and compared with other techniques commonly in current computer systems. The results of this analysis suggest that the prop osed ANN design o ers an attractive approach for the realization of query mo dules in large database and knowledge base systems, esp ecially for retrieval based on partial matches. This researchwas partially supp orted by the National Science Foundation through the grant IRI-9409580 to Vasant Honavar. A preliminary version of this pap er [Chen and Honavar, 1995c] app ears in the Pro ceedings of the 1995 World Congress on Neural Networks. 1 1 Intro duction Arti cial neural networks ANN o er an attractive computational mo del foravariety of applications in pattern classi cation, language pro cessing, complex systems mo delling, control, optimization, prediction and automated reasoning for a variety of reasons including: p otential for massively parallel, high-sp eed pro cessing, resilience in the presence of faults failure of comp o- nents and noise. Despite a large numb er of successful applications of ANN in pattern classi cation, signal pro cessing, and control, their use in complex symb olic computing tasks including storage and retrieval of records in large databases, and inference in deductive knowledge bases is only b eginning to b e explored Honavar, & Uhr, 1994; Sun, 1994; Levine & Aparicioiv, 1994; Go onatilake & Khebbal, 1995. This pap er explores the application of neural networks to database query pro cessing. Database query can b e viewed as a pro cess of table lo okup which is used in a wide variety of computing applications. Examples of such lo okup tables include: routing tables used in routing of messages in communication networks, symbol tables used in compiling computer programs written in high level languages, and lexicons or dictionaries used in in natural language pro- cessing. Many applications require the lo okup mechanism or query pro cessing system to b e capable of retrieving items based on partial matches or retrieval of multiple records matching the sp eci ed query criteria. This capabilityis computationally rather exp ensive in many current systems. The ANN-based approach to database query pro cessing that is prop osed in this pap er exploits the fact that the database lo okup task can b e viewed at an abstract level, in terms of binary mappings which can b e eciently and robustly realized using ANNs Chen & Honavar, 1994; Chen & Honavar, 1995a. The rest of the pap er is organized as follows: Section 2 reviews the mathematical mo del of multiple recall from par- tially sp eci ed input pattern in a neural network prop osed in Chen & Honavar, 1995a. Section 3 develops an ANN design for a high-sp eed library query system in detail based on the mo del describ ed in section 2. Section 4 compares the p erformance of the prop osed ANN-based query pro cessing system with that of several currently used techniques. Section 5 concludes with a summary of the pap er. 2 Multiple Recall from Partially Sp eci ed Input Most of database management systems store data in the form of structured records. When a query is made, the database system searches and retrieves records that match the user's query criteria whichtypically only partially sp ecify the contents of records to b e retrieved. Also, there are usually multi- ple records that match a query e.g., b o oks written by a particular author in a library query system. Thus, query pro cessing in a database can b e viewed as an instance of the task of recall of multiple stored patterns given a partial sp eci cation of the patterns to b e recalled. A neural asso ciative memory for p erforming this task was prop osed in Chen & Honavar, 1995a. This section summarizes the relevant prop erties of the neural memory develop ed in Chen & Honavar, 1995a. 2.1 Asso ciative Memory with Bip olar Input and Bi- nary Output The asso ciative memory used is based on 2-layer p erceptron with a layer of input neurons, a layer of hidden neurons, and a layer of output neurons; and hence twolayers of connection weights. Given a set U of k bip olar input vectors u ,...,u of dimension n and a set V of k desired binary output 1 k vectors v ,...,v of dimension m, such an asso ciative memory mo dule can b e 1 k synthesized using one-shot learning as follows: The memory mo dule has n input, k hidden and m output neurons. For each asso ciative ordered pair u ;v , where 1 i k , a hidden neuron i is created with threshold n 2p , i i i where p 2 N is the adjustable precision level for that asso ciative pair. The i connection weight from input neuron g to hidden neuron i is u and that ig from hidden neuron i to output neuron h is v . The threshold for each of the ih output neurons is set to 0. The activation function at hidden no de is binary hardlimiter function f , and that at output neuron can b e binary hardlimiter h function f or identity function I , i.e., I x= x, dep ending on the particular h hardware implementation employed. The binary hardlimiter function f is h de ned by f x=1 if x 0 and 0 otherwise. h 2.2 Asso ciative Recall from Partially Sp eci ed Input Pattern This section summarizes a mathematical mo del of asso ciative recall from a partially sp eci ed bip olar pattern and its neural network realization. The mo del assumes that the unavailable comp onents of an input pattern vector have a default value of 0. Thus, a partial input pattern is completed by lling in a 0 for each of the unavailable comp onents. Let u_ b e a partially sp eci ed n-dimensional bip olar pattern with the values of some of its comp onents b eing unknown; bits_u, a function which counts the numb er of comp onents with known values +1 or -1 of partial patternu _ ; pad0u _ , a function which pads the unavailable bits of bip olar partial patternu _ with 0's; and , a binary predicate which tests whether \ isapartial pattern of ", where \ is a partial pattern of " means that the values of available bits of are same as those of their corresp onding bits in . _ Let D _u; v_ denotes the Hamming distance b etween two bip olar partial H patterns, with same corresp onding unavailable comp onents,u _ andv _ resp ec- tively.Ifbits_u=j , pad0u _ is called as padded j-bit partial pattern derived j _ from partially sp eci ed patternu _ . De ne U = fu_ j bits_u= j &_u u g, i Pi j _ 1 j n &1 i k , i.e., U is the set of partial patterns, with j Pi j available bits, of bip olar pattern u .
Details
-
File Typepdf
-
Upload Time-
-
Content LanguagesEnglish
-
Upload UserAnonymous/Not logged-in
-
File Pages18 Page
-
File Size-