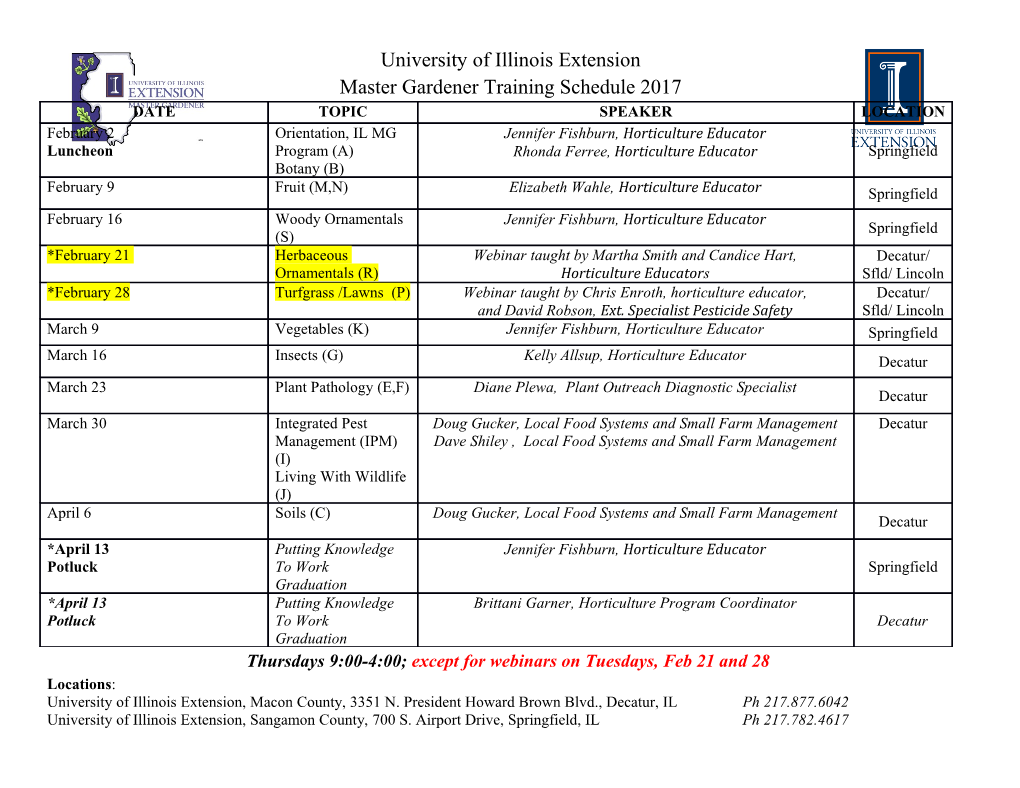
Using Data Science & Predictive Models to Produce Foresight: The case of the presumptuous assumptions Steve Cohen Partner in4mation insights Needham, MA ig Data Analytics Founders are Thought Leaders B Consumer & Market Segmentation Customer Lifetime Value & Churn Digital Attribution Top Marketing Science Advisors Market Structure Marketing Mix Modeling New Product & Service Design Hierarchical Bayesian Statistics Pricing & Promotion Optimization Assortment Optimization Retail Site Location Proprietary Hardware & Software Marketing Ecosystem Models © 2014 by in4mation insights, LLC 2 Why should you care what I have to say? First to do Choice based Conjoint Analysis commercially (1983) First academic paper for Latent Class CBCA (1995) First integrated model for multiway segmentation based on Latent Class Models (1996) First academic paper for Menu based Conjoint Analysis (2000) Introduced MaxDiff scaling at ESOMAR (2000) Best paper at Sawtooth Conference: MaxDiff (2003) ESOMAR best paper of the year award for MaxDiff (2004) Best paper in Marketing Research Magazine: MaxDiff (2005) American Marketing Association Parlin Award (2011) NextGen Marketing Research LinkedIn group: Individual Disruptive Innovator award (2012) Marketing Research Council of NYC: MR Hall of Fame (2013) Over two dozen papers and presentations at industry conferences on analytics & modeling © 2014 by in4mation insights, LLC 3 What is Data Science? What is Data Science? © 2014 by in4mation insights, LLC 5 What is Data Science? Credit: Drew Conway © 2014 by in4mation insights, LLC 6 What is Data Science? © 2014 by in4mation insights, LLC 7 Market Segmentation Analytic steps in a typical segmentation study Tandem Clustering Factor Cluster Assignment Collect Ratings Analysis Analysis Tool “Tandem clustering (i.e. factor analysis followed by cluster analysis) is an outmoded and statistically insupportable practice.” Arabie & Hubert (1994) © 2014 by in4mation insights, LLC 9 What are you doing? Principle Components Analysis Principal Components Analysis © 2014 by in4mation insights, LLC 10 What are you doing? Discriminate Analysis Discriminant Analysis © 2014 by in4mation insights, LLC 11 What’s my beef with common segmentation practice? The short list. Guiding Principle: Segmentation is a search for differences Rating Scales Factor Analysis Cluster Analysis © 2014 by in4mation insights, LLC 12 Hierarchical Bayesian Modeling What are the effects of price and in-store display on sales of supermarket product? UpperLower Model 1 = 1�+�11�∗+�� � !21 =∗__+ � +1 �"�#31� �∗ℎ+$+2 ln'41(�∗_)�"� ) 2 = 2 +12 ∗+22 ∗__+32 ∗ℎ+42 ∗_ © 2014 by in4mation insights, LLC 14 What could effect sales of SKUs in a store? Lower Model Lower Model Upper Model Base Price National TV Channel Geography Discounted Price Local TV Feature Ingredients Radio Display Location at point of sale Outdoor Form Store size Magazines Size Store age Coupons Newspapers Store format Seasonality Social media activity Company vs. franchise Holidays Website & search Demos of trading area Weather © 2014 by in4mation insights, LLC 15 Bayesian analysis works best when there are many items, brands, stores or regions that need to be compared. $28,328 TV Effectiveness: Sales/GRPs Growth Items can be opportunity compared to $16,980 average $11,939 $9,318 Category Average Items can be $6,828 $9,722 $4,766 $4,751 indexed $3,312 against their $1,518 volume Brand A Brand B Brand C Brand D Brand E Brand F Brand G Brand H Brand I Effectiveness Index per $1MM in Brand Size: 121121 3030 97 97 91 9 1 7722 125 125 181 181 111 111 7272 © 2014 by in4mation insights, LLC 16 Choice Modeling & Trade-Up What are you doing? Discreet Choice Model Discrete Choice Model © 2014 by in4mation insights, LLC 18 True or False: Discrete Choice Models are the exact same thing as Choice-based Conjoint Analysis A typology of choice models How many brands or items chosen? Only one More than one Dell HP Sony Vaio Only one Only 100 GB Hard Drive 200 GB Hard Drive 500 GB Hard Drive 4 MB RAM 2 MB RAM 4 MB RAM Basic Processor Enhanced Processor Basic Processor 17-inch Screen 19-inch Screen 14-inch Screen MS Office MS Office No MS Office 90-day Warranty 90-day Warranty 1 Year Warranty Total Price: $1,170 Total Price: $1,480 Total Price: $1,840 More than one than More How many units of each item chosen? item each of units many How © 2014 by in4mation insights, LLC 20 Price elasticity is about substitutability $229 $249 $13.99 $10.99 $234 $12.99 $199 $199 © 2014 by in4mation insights, LLC $179 21 Trade-up happens when shoppers are willing to spend more. Quality Count Size Coca-Cola Coca-Cola Coca-Cola Classic Classic Classic 12-pk, 12oz cans 12-pk, 12oz cans 2 liter bottle $5.49 $5.49 $1.89 Private Label Coca-Cola Coca-Cola Cola Classic Classic 12-pk, 12oz cans 6-pk, 12oz cans 20 oz bottle $2.99 $3.99 $1.49 © 2014 by in4mation insights, LLC 22 Trade-Up Model assumptions Products are not substitutes Trade up/down is asymmetric Consumers will purchase the most quantity that they can Subject to their budget limit Subject to diminishing returns Having money left over after making the purchase is good © 2014 by in4mation insights, LLC 23 Market share simulations: Trade-Up vs. HB CBCA Logit Model 18% 16% BRAND B (Tradeup) BRAND B (HB Logit) Market share is 14% predicted to be BRAND A (Tradeup) higher for Brand A in 12% the Trade-up model. 10% BRAND A (HB Logit) BRAND C (Tradeup) 8% BRAND C (HB Logit) 6% 4% redicted Market Share Market redicted P 2% 0% $299 $399 $499 $699 $899 Price of Brand A © 2014 by in4mation insights, LLC 24 Market share simulations: Trade-Up vs. HB CBCA Logit Model Which price elasticity makes more sense? 18% 16% 14% 12% 10% 8% BRAND A (HB Logit) 6% 4% redicted Market Share Market redicted BRAND A (Tradeup) P 2% 0% $299 $399 $499 $699 $899 Price of Brand A © 2014 by in4mation insights, LLC 25 Modeling the Marketing Ecosystem The Business Intelligence Landscape is changing. More holistic view of business needed Increasing role of social & digital media Fusing data sources into new databases Mine existing data Existing analytic tools assume static rather than dynamic view Integrate consumer-based metrics into modeling and planning models Need to accurately measure both short- and long-term marketing effects Need reliable measurement of effects of traditional marketing vs. social/digital media © 2014 by in4mation insights, LLC 27 Any time series can be modeled as a simple process, where next month is a function of previous months. Offline & Online Marketing Tactics BHMs & Social Metrics Sales © 2014 by in4mation insights, LLC 28 Some marketing tactics may have an immediate effect on sales, while others may take time to change opinions. Offline & Online Marketing Tactics I mmediate BHMs & Social Metrics Sales © 2014 by in4mation insights, LLC 29 Once opinions have changed, time passes before the impact on sales may be seen. Offline & Online Marketing Tactics I mmediate BHMs & Social Metrics Sales © 2014 by in4mation insights, LLC 30 Feedback occurs as higher sales affect consumer perceptions, leading to changes in consumer sentiment and more online ‘buzz” and activity. Offline & Online Marketing Tactics I mmediate BHMs & Social Metrics Sales © 2014 by in4mation insights, LLC 31 Recent application 200 Millward Brown attributes & funnel metrics 20 marketing spend tactics Number of channels = 11 Number of SKUs = 15 Number of time periods = 39 each SKU © 2014 by in4mation insights, LLC 32 Hierarchical Bayesian statistics Complex systems of linear or nonlinear equations Often no analytic solution Uses Monte Carlo simulation Predict quantitative or qualitative phenomena Incorporate sensible prior beliefs or knowledge Different coefficient for each unit of analysis at the “lower” level “Upper” level = Context = “why behind the what” © 2014 by in4mation insights, LLC 34 Big Data in, Big Data out Total coefficients = N_Units * (Lower + Lower * Upper) at every iteration of the Bayesian estimation So, if Lower = 50 and Upper = 100, for 5,000 iterations Confectionery ~ 3,000 SKUs 15,000,000 coefficients Laundry products ~ 5,000 SKUs 25,000,000 coefficients Auto Parts ~ 75,000 SKUs ~ 400 Million coefficients © 2014 by in4mation insights, LLC 35 Not all SKUs and retailers are created equal. Fragrances Makeup Skin Care A B Retailers C D Low High Low High Low High Lower Price Elasticity Higher © 2014 by in4mation insights, LLC 36 Trade-up has become a familiar part of the global consumer landscape. Perceptually superior and higher price © 2014 by in4mation insights, LLC 37 Behavioral Model Analytic What What Consumers` What Framework Marketers Do Think & Feel Consumers Do Brand Tracking Lack comprehensive view Advertising Testing Market Response Models Proposed Marketing Brand Health & Sales model Tactics Social Metrics Performance © 2014 by in4mation insights, LLC 38.
Details
-
File Typepdf
-
Upload Time-
-
Content LanguagesEnglish
-
Upload UserAnonymous/Not logged-in
-
File Pages38 Page
-
File Size-