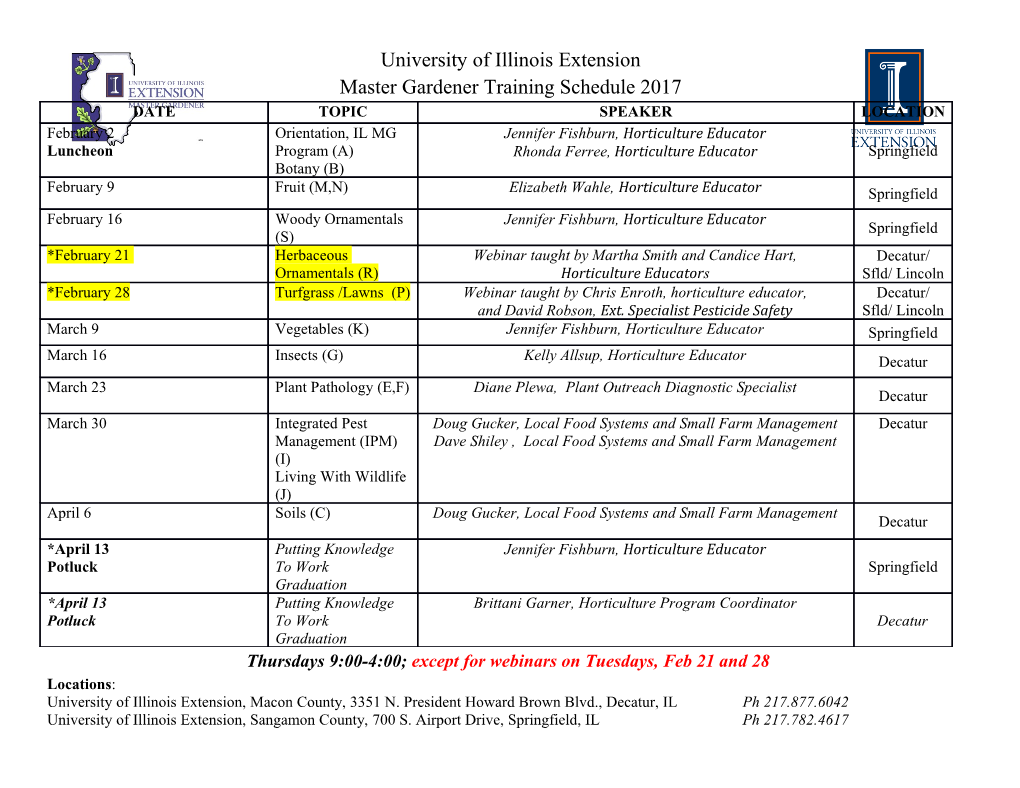
2.2 STATISTICAL ANALYSIS OF SEA SURFACE TEMPERATURE IN THE NORTH ATLANTIC OCEAN Constantin Andronache* Boston College, Chestnut Hill, Massachusetts 1. INTRODUCTION Before the satellite era, observations did not The importance of sea surface temperature cover the whole ocean, and various techniques anomalies (SSTA) for the climate of the North have been employed to produce global data sets. Atlantic region has been documented in previous Since the 1980s satellites have been increasingly studies (for instance, see Marshall et al., 2001; utilized to measure SST and have provided an Sutton and Hodson, 2003, 2005; Desser et al., enormous rise in the ability to view the spatial and 2003). Over ocean regions, there is significant temporal variation in SST. Such advances in covariance between SSTA and the anomalies of air observations contribute to improvement of model temperature at surface, in monthly, seasonal and initial and boundary conditions specification, as well annual average values. Such covariance is due to as in statistical analyses of climate variations exchange of heat and momentum between the (Marshall et al., 2001). In this study we use the upper ocean layer and the air near surface (Desser NOAA SST monthly time series (1856 – 2008), and et al., 2003). Oceans have significant thermal the NCEP/NCAR SST data sets (1948 – 2008) for inertia and provide slow variations of the lower the North Atlantic ocean. boundary of the atmosphere (Saravanan, 1998). This feature is useful in studies of climate 3. METHOD predictability at time scales from season to decade. SSTA variations have been linked to climate Three methods are used to obtain the monthly variations in North America and Europe. Such SST residuals of importance for seasonal climate studies are supported by statistical analysis of variations at the time scale of interest. These observations as well as by recent modeling studies methods aim to obtain approximately stationary (Marshall et al., 2001). A notable phenomenon for yearly time series of the monthly SSTA. We are the long term SST behavior in North Atlantic in the particularly interested in the correlation between Atlantic Multidecadal Oscillation (AMO) which SSTA between various months, in the stationary provides support for climate predictions at decadal time series (Wilkis, 1995; von Storck and Zwiers, time scale (Knight et al., 2005; 2006). It has been 1999). The presence of the long term trend and reported that some climate predictability might be AMO type variations in the original SSTA time possible at various scales, as long as strong SST series tends to produce high correlations. We signals or oscillations are identified (such as ENSO, attempt to describe the SSTA correlations at short events, for example) (Keller, 1999; Marshall et al., time scales (such as season), which might become 2001; Andronache and Phillips, 2008). Research is more evident after achieving stationary conditions. underway to address the climate prediction at These three methods are briefly described here. seasonal scale and develop practical applications, where such forecasts can have significant impact 1) For each month, from the yearly time series: a) (Palmer, 2010; Timofeyeva, 2010; Tugrul Yilmaz, remove the linear trend; b) remove the AMO type of 2010). In the context of the climate predictability, oscillation (Andronache, 2009). The long term trend we address the problem of SSTA persistence at is largely attributed to increase of global seasonal scale in North Atlantic ocean. The data temperature over the last century (Andronache and and methodology are presented in the next section. Phillips, 2008). The AMO phenomenon is an internal oscillation of the ocean circulation, driven 2. SST DATA by a natural mode of variability in the thermohaline circulation in the North Atlantic, with possible There are complete global monthly SST analyses, alterations due to climate change (Delworth and used extensively in climate studies (Reynolds and Mann 2000; Knight et al., 2005). Smith, 1994; Kaplan et al., 1998). Such data sets are constrained by quality, quantity and distribution 2) Remove the linear trend between breakpoints of the original measurements (Hurrell and (BP) in the time series. This method does not make Trenberth, 1999). any assumptions about long term trend over the _________________________________________ whole time interval, and does not consider explicitly *Corresponding author address: Constantin any low-frequency oscillation. Instead, it finds the Andronache, Boston College, Chestnut Hill, MA local min and max SST values over few decades, 02467, e-mail: [email protected]. and does a linear regression between two such consecutive local extreme values. Thus, it removes 1 the linear trend established between such BP, as which is the difference (y – yLT), versus time, in will be illustrated in Figure 4. years. This residual SST has no trend but exhibits a multidecadal oscillatory component. Figure 3 3) Remove a polynomial fit of the SST time series shows: (a) The January monthly SST residual (y – (Andronache et al., 2008). This method does a yLT) and the least square of a sine function, versus least squares (LS) fit with low order polynomials, as time, in years; (b) The January monthly SST an approximation of the data over the whole residual r1, versus time, in years. This residual time observation period. The method works well for short series is approximately stationary. time series, and for low order polynomials. High order polynomials tend to present too much detail 24 of the original time series, and have large errors at Jan Feb the end of the time interval. 23 Mar Apr Notations used in this study: 22 y – the yearly time series of the monthly average May Jun SST for a given month; 21 Jul yLT – the linear trend of y; SST (C) Aug yAMO – the sine LS fit of the (y-yLT); 20 Sep r1 = y- yLT – yAMO – the SST residual of y after Oct removal of linear trend and AMO approximation. 19 Nov Dec 18 For method 2, the residual r2 is defined as 1860 1880 1900 1920 1940 1960 1980 2000 2020 Year r2=y-yBP (the difference between y and the LS linear fit between breaking points of y). Fig. 1. Time series of the monthly SST versus time, For method 3, the residual r3 is in years. r3=y-yP (the difference between y and the polynomial fit of y, where the order of the polynomial used in this study is n = 7). (a) 20 y The three methods provide similar results, and y 19.5 LT they were used to check the robustness of the first one which is illustrated here in detail. The time 19 series of the SST residuals obtained by the above SST (C) 18.5 methods are stationary in weak sense (the mean 1860 1880 1900 1920 1940 1960 1980 2000 2020 and the variance are constant in time). We illustrate (b) how SST residuals are obtained by removing the 0.5 linear trend and the AMO type oscillation. ) (C) LT 0 4. RESULTS AND DISCUSSION (y − y −0.5 1860 1880 1900 1920 1940 1960 1980 2000 2020 Year Figure 1 shows the SST time series for each month. Due to the annual cycle, there are pairs of months with very similar variations. For instance, at Fig. 2. (a) Time series of monthly SST for January, the bottom of the plot, February and March SST versus time in years. The linear trend in least have very similar behavior, and they seem highly square sense is shown; (b) The monthly SST correlated. These months correspond to the lowest residual for January, (y – yLT), versus time, in years. SST values during the cold season in North Atlantic region. At the top of the plot, August and This procedure in applied for all months. We note September SST have similar variations, that yAMO, approximated by a sine function with corresponding to the highest values in the warm three parameters (amplitude, period, and phase) is season. Few characteristics are evident: a) the long the first Fourier component of the time series, which term positive trend; b) the multidecadal oscillation. correspond to a well defined physical phenomenon We are interested to find out a statistical (AMO). By taking more Fourier series terms, we relationship between SST in various months, and can obtain a better approximation of the (y – yLT) we have to produce SST time series that are data, at shorter time scales, but our purpose here is stationary. These conditions are illustrated in to remove only the dominant multidecadal Figures 2 and 3 for the month of January. Figure 2 oscillatory features (such as AMO). The application shows: (a) the time series of monthly SST for of this method for all twelve months produced some January, versus time in years. The LS linear trend variability in the fitting parameters (amplitude, is shown; (b) the monthly SST residual for January, period, and phase), consistent with previous results. 2 4.2 Correlation of SST residuals (a) (y − y ) LT 0.5 y We note first that the residuals obtained by the AMO three methods are similar. Figure 4 a shows the ) (C) LT 0 SST and the linear regression between BP in the time series. Figure 4 b shows the polynomial fit (y − y −0.5 (order = 7) of the same data. Figure 4c compares 1860 1880 1900 1920 1940 1960 1980 2000 2020 the three residuals obtain using these methods. (b) More detailed tests (not shown) were performed to 0.4 (C) determine any significant variations between these 1 0.2 methodologies, and we found that they produce 0 similar stationary time series of the SST residuals. −0.2 −0.4 Residual r 1860 1880 1900 1920 1940 1960 1980 2000 2020 (a) 20 Year y 19.5 y BP 19 SST (C) 18.5 Fig.
Details
-
File Typepdf
-
Upload Time-
-
Content LanguagesEnglish
-
Upload UserAnonymous/Not logged-in
-
File Pages5 Page
-
File Size-