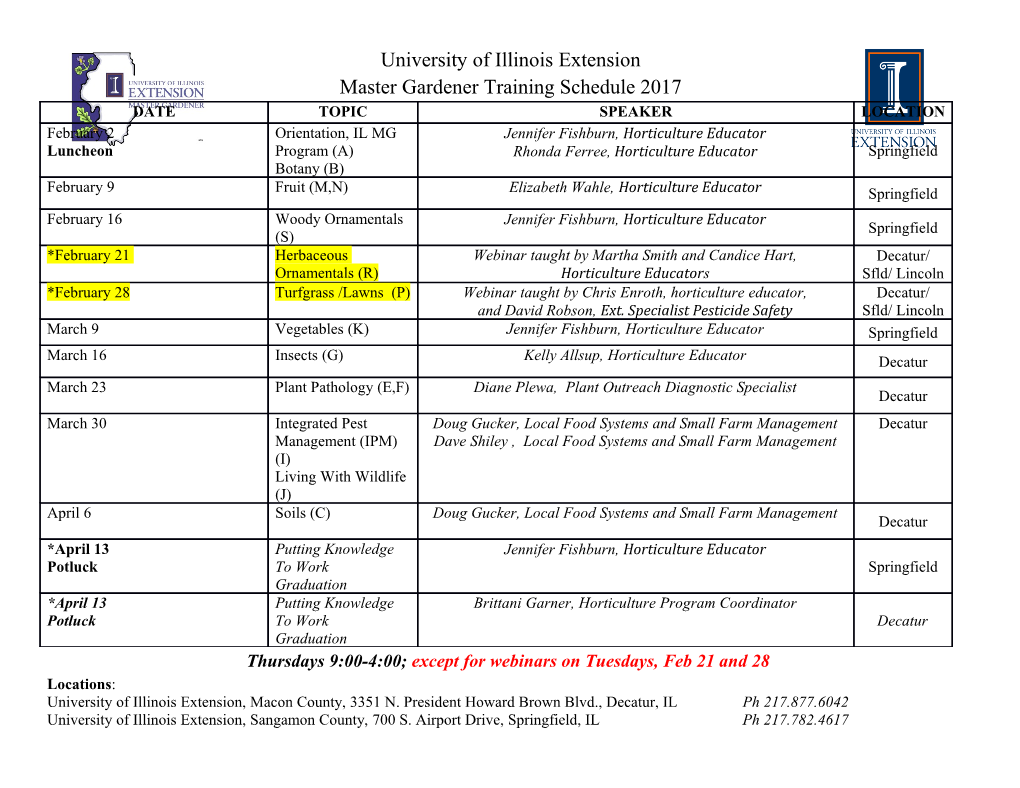
B u m p e r X m a s E d i The Devil’s Advocate tio n The odd newsletter from the Jolly Contrarian Edition 1: December 2020 Here’s looking at you, old normal The Devil’s Advocate 2 December 2020 The End of Insurance? Will the rise of the networked economy and the emerging power of big data supercharge the business of insurance, or kill it? Through the prism of new-age insurer Root, Marc Rubinstein investigates. Daniel Bernoulli wasn’t even the wealthy than this would be fool- surance goes like this: best mathematician in his own ish to provide the surety, but it “For centuries, traditional insur- family. But his contribution to makes sense for a wealthier man ance companies have grouped reckons that only now, through the study of risk is profound. to do so.” people into risk pools and long mobile phone deployment, has it In his essay of 1738, Exposition It wasn’t a particularly useful relied on the ‘law of large num- become scalable. Its predictive of a New Theory on the Measure- theory and in 1979 it was fa- bers’ to produce acceptable pric- analytics are a work-in-progress, ment of Risk, Bernoulli applies mously revised by Daniel Kahne- ing on an aggregate basis. Fair- but it reckons that the worst his mind to the question of insur- man and Amos Tversky.1 But it ness at the individual level has 10-15% drivers it screens are two ance. The basics of probability captures why insurance compa- been largely ignored. Root is dif- times more likely to get in an ac- theory had already been un- nies are big. ferent—we use technology to cident than its average targeted customer. earthed, but Bernoulli was puz- Insurance is predicated on two measure risk based on individual zled by how insurance fit into its principles: performance, prioritizing fair- Various third party vendors sell framework. ness to the customer.” similar data: Law of large numbers. If you re- His thinking goes like this: to be peat a random experiment often The company relies on data to Octo Telematics claims it has 248 viable, an insurer would need to enough, the average of the out- predict the probability of a cus- billion miles of driving data col- charge a premium at least equal comes will converge towards the tomer suffering a loss. It gathers lected and over 464,000 insur- to the expected value of any expected value. A larger book of this data from customers’ mobile ance events analysed; claims that may be made against business therefore confers phones, picking up braking and Cambridge Mobile Analytics it; to lock in a profit margin, the greater loss clarity to an insurer turning speeds, miles driven, partners with State Farm, Liberty insurer would have to charge than a smaller book. The greater phone usage while driving and Mutual, Nationwide and other more. Yet why would a buyer the number of similar risks the more. Its app picks up over 200 leading insurers and has Soft- want to pay more than the ex- insurer can bring together, the factors in total, which add to oth- bank backing; pected value of their losses? An er, traditional factors like age, higher their confidence at pre- Greater Than is a listed Swedish insurance contract is zero-sum— gender and ZIP code. dicting overall claims. technology company which has one party’s loss is the other par- Risk pooling. For typical insur- In the traditional model, risk analysed 750 million driving pro- ty’s gain. Which means that in a able risks, the frequency of pools would be formed along a files. It claims its AI “predicts ac- world of economically rational claims is low and an insurer can set of rate factors, with each pool cidents before they happen”. people, there isn’t a price at corresponding to a certain com- spread losses suffered by a few Taken to its extreme, individual which insurance makes sense. bination of rate factor categories policyholders across a large risk profiling undermines the Clearly, empirical evidence is (or intervals where the factors group of similar policies. A hun- very need for insurance. If acci- such that insurance does make are continuous). Within each dred people of similar risk profile dents can be predicted before sense. Today, buyers spend $6.3 pool, actuaries analyse historic each paying a premium of $200 a they happen, they become unin- trillion a year on insurance pre- claims data to arrive at an esti- year can cover the risk of any one surable—like an insurance un- miums. mate of the minimum payment of them losing $20,000. derwriter’s Minority Report. per policy required to cover ex- Bernoulli proposed a fix that be- These twin concepts give an in- More realistically, predictive ana- pected losses. In order to over- came a cornerstone of economic surance company the grounding lytics could lead to materially come adverse selection – where thinking for the next two and half to offer policyholders risk trans- higher rates for riskier con- policyholders take advantage of centuries. He suggested that fer at an acceptable premium. sumers, making insurance unaf- an insurance company that has rather than consider profit and They allow the transformation of fordable for them. Insurance was failed to price risk correctly – a loss in absolute terms, the parties unknown individual uncertainty built upon the recognition of the bonus system is incorporated to consider the utility of their profit about the future into a measur- irreducible opacity of individu- reduce the premium for policy- and loss. Because their utility able aggregate risk. als; behavioural data offers to lift functions don’t have to be the holders with a good claims histo- Except now we have data. Lots that opacity. same, a price emerges at which ry. and lots of data. Data can be cap- For various reasons, we’re not yet both parties are comfortable en- Root characterises this process as tured on a much more granular at that extreme. Most insurance tering an insurance contract. one of correlation and not and personalised level of detail. companies use behavioural data Bernoulli suggested that such causality, and it “strive[s] to This means that rather than rely as a complement to their tradi- utility was a function of how price based more on causality on a collective approach to risk, tional methods of pricing, re- much wealth each of the parties than correlation”. So it mines insurers can underwrite to spe- warding customers with a dis- had in reserve. The greater the driving performance data for sig- cific individualised risk. count on the traditional tariff. wealth of the insurance buyer, nals of cause. It claims to have Even Root, despite its disruptive the lower their propensity to buy Enter Root data from over 10 billion miles of credentials, isn’t there yet: insurance. Meanwhile, the Next week Root goes public. It’s driving and hundreds of thou- greater the wealth of the insurer, an insurance company focussed sands of claims. Although telem- “Over time we hope that we can the greater their propensity to on the US auto market. Their atics technology has been around replace all correlation-related in- sell insurance: “A man less view on the twin principles of in- for many years, the company puts to our pricing model, such The Devil’s Advocate December 2020 3 as credit scores, with a fully be- Second is that there are some havioral pricing model.” regulatory implications. Policy- This edition’s contributors makers are concerned about data The company recently an- Graeme Johnston nounced an initiative to remove privacy (a risk factor cited by credit scoring from its underwrit- Root) and also about discrimina- Graeme is the CEO of Juralio, a software ing criteria by 2025. In the tion. Discrimination applies un- company based in Scotland. He’s also meantime, its pricing still relies der current standards, too—Eu- coordinating the open source legal project noslegal.org. Get in heavily on it, together with other ropean legislation prohibits the touch with Graeme at [email protected] use of a gender rating; but if al- traditional factors like age, gen- Electra Japonas der and ZIP code. An analysis of gorithms reverse engineer gen- Root’s regulatory rate filings der, that could be seen as prob- Electra is the CEO and shows that its premium has his- lematic. founder of The Law Boutique, torically tracked Progressive’s. Finally, driver behaviour may not a legal company that takes a holistic approach to legal support, Its premium per policy isn’t that be as consistent as fixed variables beyond just the law. Get in touch with Electra at different either, so it doesn’t look like age and address. Root under- [email protected] like it’s picking up analytically writes policyholders after observ- lower risk customers by offering ing their driving patterns for two Andrew Pegler them a fairer price. to four weeks on a test drive. Yet Andrew is the Founder and CEO of Andrew Nor are the benefits of predictive six months later, it doesn’t offer Pegler Media, is an award winning plain analytics visible in its loss trends. renewal policies to a third of pol- English training, editing and writing agency The company reports losses of icyholders, indicating a change in in Melbourne. Get in touch with Andrew at around 100 cents on every dollar behaviour between the first two www.andrewpeglermedia.com.au of premium earned. That com- to four weeks and the next six Marc Rubinstein pares with peers who lose around months.
Details
-
File Typepdf
-
Upload Time-
-
Content LanguagesEnglish
-
Upload UserAnonymous/Not logged-in
-
File Pages14 Page
-
File Size-