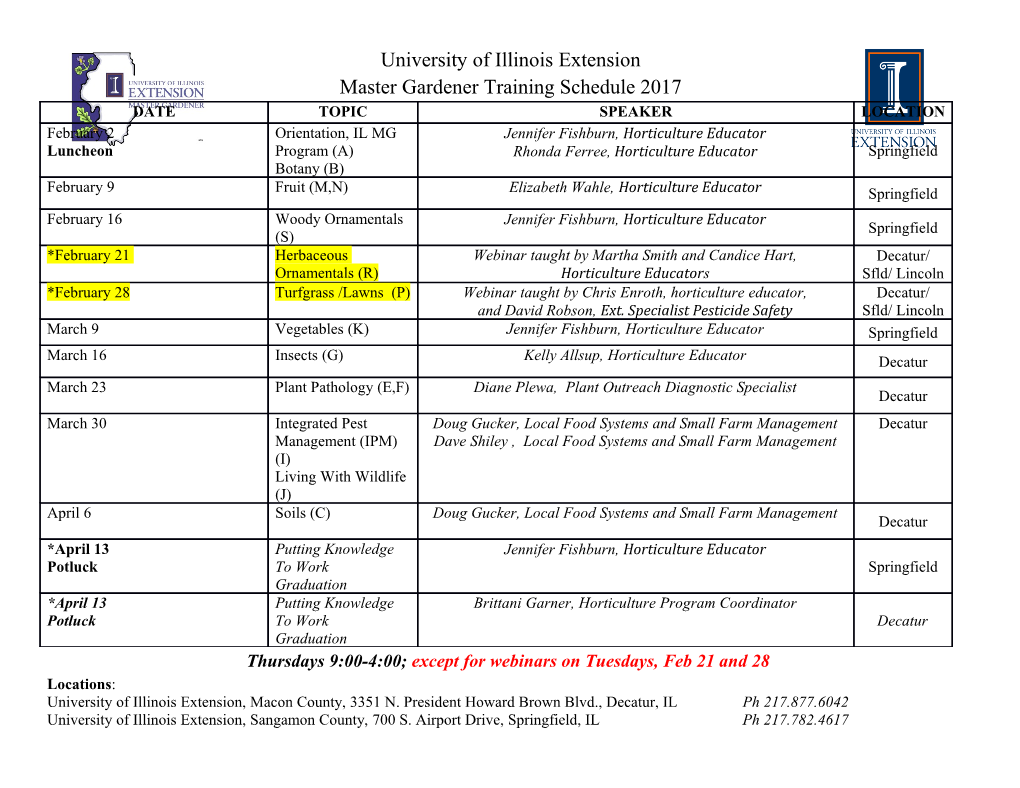
STAT3014/3914 Applied Stat.-Sampling C3-Ratio & reg est. 3 Ratio and regression estimators 3.1 Motivating examples Frequently, we are interested in measuring the ratio of a matched pair of variables. This occurs when the sampling unit comprises a group or cluster of individuals, and our interest is in the population mean per individual. For example, to estimate average income/adult in the population in a household survey, we record for the ith household (i = 1; ··· ; n) the number of adults who live there, xi, and the household income, yi. Then the parameter, average income per adult in the population, N P Y household income i R = = i=1 N total no. of adults P Xi i=1 can be estimated by the ratio estimator n P yi i=1 y¯ Rb = r = n = : P x¯ xi i=1 Relationship between estimates Ratio Mean Total R −!×X Y −!×N Y R −!×X Y SydU STAT3014 (2015) Second semester Dr. J. Chan 34 STAT3014/3914 Applied Stat.-Sampling C3-Ratio & reg est. 3.2 Two characteristics per unit in SRS Theorem: If Xi and Yi are a pair of numerical characteristics defined on every unit of the population, andy ¯ andx ¯ are the corresponding means from a SRS without replacement of size n , then " # n 1 PN (Y − Y¯ )(X − X¯ ) n S Cov (¯x; y¯) = 1 − i=1 i i = 1 − xy N n N − 1 N n (1) and Pn (y − y¯)(x − x¯) PN (Y − Y¯ )(X − X¯ ) E i=1 i i = i=1 i i : (2) n − 1 N − 1 Proof. Consider Ui = Xi +Yi and the corresponding sample values are ui = xi + yi. Clearly " # n S2 n 1 PN (X − X¯ + Y − Y¯ )2 Var (¯u) = 1 − U = 1 − i=1 i i N n N n N − 1 " # n 1 PN (X − X¯)2 + PN (Y − Y¯ )2 + 2 PN (X − X¯)(Y − Y¯ ) = 1 − i=1 i i=1 i i=1 i i N n N − 1 " # 2 PN (X − X¯)(Y − Y¯ ) n = Var (¯x) + Var (¯y) + i=1 i i 1 − : n N − 1 N Since Var (u¯) = Var (¯x +y ¯) = Var (¯x) + Var (¯y) + 2Cov(¯x; y¯), (1) is proved. (2) can be proved in a similar way. Theorem: For large sample, (a) E(r) − R ≈ 0, approximately unbiased, " # 1 n 1 PN (Y − RX )2 1 n S2 (b) Var(r) ≈ 1 − i=1 i i = 1 − r : X¯ 2 N n N − 1 X¯ 2 N n SydU STAT3014 (2015) Second semester Dr. J. Chan 35 STAT3014/3914 Applied Stat.-Sampling C3-Ratio & reg est. Proof: (a) Recall E(y¯) = Y¯ , E(x¯) = X¯ and Var(x¯) = O(n−1) (order of n−1). Thus for large sample, y¯ E(y¯) E(r) = E ≈ = R: x¯ X¯ (b) Note that y¯ y¯ − Rx¯ r − R = − R ≈ : x¯ X¯ Thus, for large sample, 1 E(d¯2) Var(d¯) Var(r) = E[(r − R)2] ≈ E[(y¯ − Rx¯)2] = = X¯ 2 X¯ 2 X¯ 2 ¯ where d = y¯−Rx¯ is the sample mean of di = yi−Rxi, i = 1; ··· ; n, drawn from the population of Di = Yi − RXi; i = 1; ··· ;N with Y¯ E(d¯) = E(y¯ − Rx¯) = E(¯y) − RE(¯x) = Y¯ − RX¯ = Y¯ − X¯ = 0: X¯ For a SRS of di, n S2 Var(d¯) = 1 − r N n where N N 1 X 1 X S2 = (D − D¯ )2 = (Y − RX )2: r N − 1 i N − 1 i i i=1 i=1 Hence " N # 1 n 1 1 X 1 n S2 Var(r) ≈ 1 − (Y − RX )2 = 1 − r ; X¯ 2 N n N − 1 i i X¯ 2 N n i=1 " n # 1 n 1 1 X 1 n s2 var(r) ≈ 1 − (y − rx )2 = 1 − r X¯ 2 N n n − 1 i i X¯ 2 N n i=1 SydU STAT3014 (2015) Second semester Dr. J. Chan 36 STAT3014/3914 Applied Stat.-Sampling C3-Ratio & reg est. 2 1. Ordinary: x not related to y ¯ ¯ n sy Yb = y¯ & var(Yb) = 1 − N n y 6 yi s Solid line: yi − y¯ © y¯ s P (y −y¯)2 s 2 i i sy = n−1 s s -x 2 2. Ratio: x positively related to y ¯ X ¯ n sr Yb r = y¯ x¯ & var(Yb r) = 1 − N n y 6 yi Solid line: zi = yi − rxi rxs i rX ¯ 2 s Y P z s 2 i i 2 6 sr = n−1 < sy sy = rx 2 2 2 = sy − 2rρs^ xsy + r sx s(a = 0,b = r) - x X 2 Calculation of sr: n 1 X s2 = (y − rx )2 r n − 1 i i i=1 n 1 X y¯ = [(y − y¯) − r(x − x¯)]2 since y¯ − rx¯ = y¯ − x¯ = 0 n − 1 i i x¯ i=1 " n n n # 1 X X X = (y − y¯)2 − 2r (x − x¯)(y − y¯) + r2 (x − x¯)2 n − 1 i i i i i=1 i=1 i=1 2 2 2 2 2 2 = sy − 2r sxy + r sx = sy − 2r ρs^ xsy + r sx n n n ! 1 X X X or s2 = y2 − 2r x y + r2 x2 : r n − 1 i i i i i=1 i=1 i=1 SydU STAT3014 (2015) Second semester Dr. J. Chan 37 STAT3014/3914 Applied Stat.-Sampling C3-Ratio & reg est. Remark: 2 2 1. If Xi and Yi are positively related, we have sr sy. Hence Xi can be used as an auxiliary variable which provides additional information and hence improves the precision of the estimate Y¯ . 2. When X is replaced by x if it is unknown, ordinary estimator results. 3. When ratio estimation is used, estimates of variance and sample size are quite sensitive to data points that do not fit the ideal pattern called influential observation. It is important to plot the data and look for these unusual data points before proceeding with an analysis. y 4. The `ratio of means' Rb = x is biased and can be almost unbiased if n is large. Another ratio estimator is the `mean of ratios' n N R∗ = r∗ = 1 P yi where r∗ = yi is unbiased for R∗ = 1 P yi . b n xi i xi N xi i=1 i=1 However Rb∗ gives equal weight to each cluster which may vary greatly in size. Unlike Rb∗, Rb is weighed by the cluster size which is an advantage over Rb∗. SydU STAT3014 (2015) Second semester Dr. J. Chan 38 STAT3014/3914 Applied Stat.-Sampling C3-Ratio & reg est. 3.3 Ratio estimate for population mean and total The ratio estimator of the population total Y is y¯ Ybr = X = rX x¯ Similarly, the ratio estimator of population mean is y¯ Yb¯ = X¯ = rX¯ r x¯ These ratio estimates use extra information of xi; i = 1; ··· ; n and the true total and mean X or X¯ , thus improving the precision of ratio estimates over the ordinary estimates Yb = Ny¯ and Yb¯ =y ¯ respectively. From the previous result, (a) E(Yb¯ r) = XE¯ (r) ≈ XR¯ = Y¯ . Similarly E(Ybr) = XE(r) ≈ XR = Y . 2 2 ¯ n Sr 2 n Sr (b) Since Var(Yb r) ≈ 1 − and Var(Ybr) ≈ N 1 − , N n N n 2 2 ¯ n sr 2 n sr var(Yb r) = 1 − and var(Ybr) = N 1 − : N n N n ¯ The estimator r for R is generally biased , so Ybr and Yb r are also biased for Y and Y¯ respectively. Bias: y¯ Cov(r; x¯) = E(rx¯) − E(r)E(¯x) = E x¯ − E(r)E(x¯) x¯ so E(¯y) Cov(r; x¯) ρ σ σ E(r) = − = R − r;x¯ r x¯ : E(¯x) E(¯x) X¯ SydU STAT3014 (2015) Second semester Dr. J. Chan 39 STAT3014/3914 Applied Stat.-Sampling C3-Ratio & reg est. Therefore for any ratio estimates, jbias rj jR − E(r)j ρ σ σ = = r;x¯ x¯ ≤ x¯ = cv(¯x) (3) σr σr X¯ X¯ since jρr;x¯j ≤ 1. Thus if the CV(x¯) is small, the bias of Rb = r is small relative to SE(r) of Rb. But if n is small, the bias can be large. Efficiency: The ratio estimator is more efficient than the ordinary estimator, that is var(Yb) > var(Yb r), if cv(x) ρ^ > (4) 2cv(y) where cv(y) is the sample cv for Y defined as s cv(y) = y : y Then n 1 var(Yb) − var(Yb ) > 0 ) 1 − [s2 − s2] > 0 r N n y r 2 2 2 2 ) [sy − (sy − 2rρs^ xsy + r sx)] > 0 ) rsx(2^ρsy − rsx) > 0 ) 2ρs^ y − rsx > 0 since r > 0 & sx > 0 y s cv(x) y ) ρ^ > x = since r = x 2sy 2cv(y) x cv(x) and the equality holds when ρ^ = . 2cv(y) SydU STAT3014 (2015) Second semester Dr. J. Chan 40 STAT3014/3914 Applied Stat.-Sampling C3-Ratio & reg est. Example: (7-11) The manager of 7-11 is interested in estimating the total sale in thousands for all of its 300 branches. From last year record, the total sale in thousands for all the 300 branches is 21300. Careful check of this year records are obtained for a SRS of 15 branches with the following results: Branch Last year sale x This year sale y Branch Last year sale x This year sale y 1 50 56 9 100 165 2 35 48 10 250 409 3 12 22 11 50 73 4 10 14 12 50 70 5 15 18 13 150 95 6 30 26 14 100 55 7 9 11 15 40 83 8 25 30 n n n n n X X 2 X X 2 X xi = 926; xi = 117400; yi = 1175; yi = 231815; xiyi = 155753 i=1 i=1 i=1 i=1 i=1 2 sy = 9983:81 The ordinary estimate of the total sale this year in thousands is 1175 Yb = Ny = 300 = 23500 15 with r 2 s n sy 15 9983:81 se(Yb) = N (1 − ) = 300 1 − = 7543:72: N n 300 15 The ratio estimate and its se for the total sale this year in thousands are 1175 Ybr = Xr = 21300 = 27027:54 926 SydU STAT3014 (2015) Second semester Dr.
Details
-
File Typepdf
-
Upload Time-
-
Content LanguagesEnglish
-
Upload UserAnonymous/Not logged-in
-
File Pages27 Page
-
File Size-