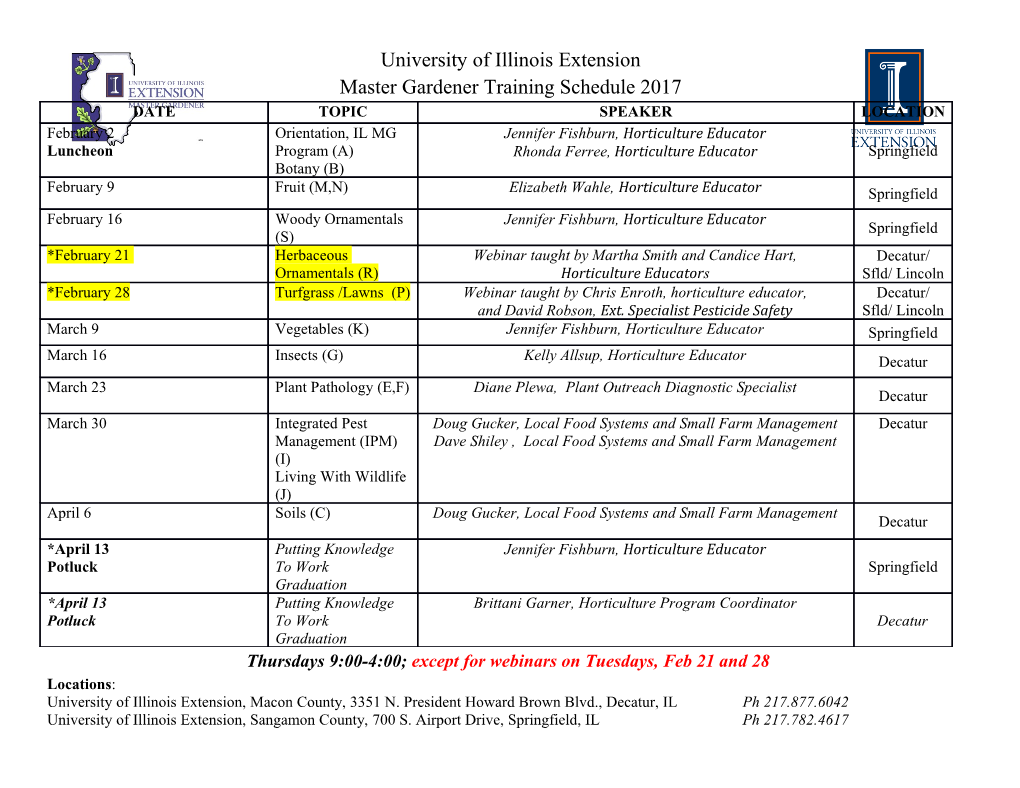
MASTER’S THESIS KENNETH LOLK VESTER MOSES CLAUS MARTINY INFORMATION RETRIEVAL IN DOCUMENT SPACES USING CLUSTERING Department of Informatics and In collaboration with: Mathematical Modelling AUGUST 2005 Technical University of Denmark Technical University of Denmark Informatics and Mathematical Modelling Building 321, DK-2800 Kongens Lyngby, Denmark Phone +45 4525 3351, Fax +45 4588 2673 [email protected] www.imm.dtu.dk In collaboration with: Mondosoft A/S Vestergade 18 E DK-1456 Copenhagen K, Denmark Phone +45 7020 2009, Fax +45 7020 2509 [email protected] www.mondosoft.com IMM-THESIS: ISSN 1601-233X “Information is the oxygen of the modern age. It seeps through the walls topped by barbed wire, it wafts across the electrified borders.” – Ronald Reagan Abstract Today, information retrieval plays a large part of our everyday lives – especially with the advent of the World Wide Web. During the last 10 years, the amount of information available in electronic form on the Web has grown exponentially. However, this development has introduced problems of its own; finding useful information is increasingly becoming a hit-or-miss experience that often ends in information overload. In this thesis, we propose document clustering as a possible solution for improving information retrieval on the Web. The primary objective of this project was to assist the software company Mondosoft in evaluating the feasibility of using document clustering to improve their information retrieval products. To achieve this end, we have designed and implemented a clustering toolkit that allows experiments with various clustering algorithms in connection with real websites. The construction of the toolkit was based on a comprehensive analysis of current research within the area. The toolkit encompasses the entire clustering process, including data extraction, various preprocessing steps, the actual clustering and postprocessing. The aim of the document clustering is finding similar pages and, to a lesser degree, search result clustering of webpages. The toolkit is fully integrated with Mondosoft’s search engine and utilises a two-stage approach to document clustering, where keywords are first extracted and then clustering is performed using these keywords. The toolkit includes prototype implementations of several promising algorithms, including sev- eral novel ideas/approaches of our own. The toolkit implements the following 5 clustering algorithms: K-Means, CURE, PDDP, GALOIS and a novel extended version of Apriori. In addition to this, we introduce two novel approaches for extracting n-grams and a novel keyword extraction scheme based on Latent Semantic Analysis. To test the capabilities of the implemented algorithms, we have subjected them to extensive performance tests, both in terms of memory and computational requirements. Our tests clearly show that CURE and GALOIS become infeasible in connection with larger websites (10,000+ pages). To evaluate the quality of the remaining three algorithms and the toolkit in general, we have also performed a user test based on the similar pages found by the algorithms. The user test shows with statistic significance that the quality of the algorithms for this task can be ranked in the following order: Apriori, K-Means and PDDP. Furthermore, the test provided evidence that both K-Means and Apriori were as good as or better than a search-based approach for finding similar pages. Finally, we have found strong evidence that the LSA-based method for keyword extraction is better for subsequent clustering, than pure truncation of terms based on their local weight. Preface This thesis is submitted in partial fulfillment of the requirements for the Master of Science degree in Engineering (M.Sc. Eng.) at the Technical University of Denmark (DTU), Kongens Lyngby, Copenhagen. The authors are Moses Claus Martiny and Kenneth Lolk Vester. The project supervisor was Jan Larsen from the Department of Informatics and Mathematical Modelling at DTU. The thesis was made in collaboration with Mondosoft A/S. Project work was done at Mondosoft in Copenhagen during the Spring and Summer of 2005. Acknowledgements Before we commence presenting our work, we would like to extend our thanks to the people who made it possible. First, everyone at Mondosoft - none mentioned, none forgotten - for their invaluable help and inspiration during the project. Our supervisor Jan Larsen for keeping us on track and providing us with help, inspiration and encouragement throughout the project. Dr. Irwin King from the Chinese University of Hong Kong for providing the initial spark of inspiration for this project and for his help during the project. Our test participants for taking the time to provide us with the test data necessary for assessing the quality of our algorithms. Finally, we would like to thank our teachers at DTU and elsewhere for providing us with the tools necessary to undertake a project of this magnitude. Kongens Lyngby, August 11, 2005 Kenneth Lolk Vester Moses Claus Martiny Contents I Main Report 1 1 Introduction 1 1.1 Structure of the Report . 1 2 Background and Scope 5 2.1 A Brief Introduction to Information Retrieval . 5 2.1.1 The Boolean Model . 7 2.1.2 The Vector Model . 8 2.1.3 Other Models . 9 2.2 Document Clustering . 9 2.2.1 The Cluster Hypothesis . 10 2.2.2 Applications of Document Clustering . 10 2.2.3 A Taxonomy of Clustering Methods . 11 2.2.4 Challenges in Document Clustering . 13 2.3 Scope of the Project . 14 2.3.1 Introduction to Mondosoft . 15 2.3.2 Problem Definition . 16 3 Analysis and Literature Study 17 3.1 Common Clustering Methods . 18 3.1.1 Partition-based Clustering . 19 3.1.2 Hierarchical Clustering . 20 3.1.3 Keyword-based Clustering . 22 3.1.4 Model-based Clustering . 23 vi CONTENTS 3.1.5 Other Clustering Methods . 24 3.2 Using Latent Semantic Analysis (LSA) to Improve Clustering . 25 3.2.1 Introduction to LSA . 25 3.2.2 Singular Value Decomposition (SVD) . 27 3.2.3 Using LSA in Connection with Document Clustering . 29 3.3 Commonly Used Preprocessing Techniques . 30 3.3.1 Stop Words and Other Kinds of Term Filtering . 30 3.3.2 Stemming . 31 3.3.3 Term Weighting . 34 3.3.4 Usage of N-Grams . 37 3.4 Postprocessing . 38 3.4.1 Finding Similar Documents (More-Like-This) . 38 3.4.2 Search Result Clustering . 38 4 Chosen Approach 41 4.1 Using Core-Terms/Keywords to Represent Documents . 41 4.2 Chosen Algorithms . 42 4.3 Toolkit Architecture . 44 4.3.1 A Modular Design . 44 4.3.2 Internal Data Representation . 46 5 Implemented Preprocessing 47 5.1 Extraction of Data from MondoSearchTM ........................... 47 5.2 Filtering of Terms . 48 5.3 Stemming . 49 5.3.1 Porter Stemmer . 49 5.3.2 Data Structures: Trie . 49 5.3.3 Data Structures: Stem Group . 50 5.3.4 Modifications to the Porter Stemmer . 50 5.4 Term Weighting . 51 5.4.1 Local Term Weighting . 51 CONTENTS vii 5.4.2 Global Term Weighting . 51 5.5 Bigram Extraction . 52 5.5.1 Scheme 1: Using Behaviour Tracking Data . 52 5.5.2 Scheme 2: Using Frequent 2-itemsets . 53 6 Implemented Keyword Extraction Algorithms 55 6.1 Synergy - LSA Based Keyword Extraction . 55 6.1.1 Description . 55 6.1.2 Algorithm . 57 6.1.3 Time and Memory Complexity . 58 6.1.4 Advantages . 59 6.1.5 Weaknesses . 60 6.1.6 Implementation Details . 60 6.2 Pure Truncation . 62 7 Implemented Clustering Algorithms 63 7.1 K-Means . 63 7.1.1 Description . 63 7.1.2 Algorithm . 63 7.1.3 Time and Memory Complexity . 64 7.1.4 Advantages . 65 7.1.5 Weaknesses . 65 7.1.6 Implementation Details . 65 7.2 CURE . 68 7.2.1 Description . 68 7.2.2 Algorithm . 69 7.2.3 Time and Memory Complexity . 71 7.2.4 Advantages . 71 7.2.5 Weaknesses . 72 7.2.6 Implementation Details . 72 7.3 PDDP............................................... 73 viii CONTENTS 7.3.1 Description . 73 7.3.2 Algorithm . ..
Details
-
File Typepdf
-
Upload Time-
-
Content LanguagesEnglish
-
Upload UserAnonymous/Not logged-in
-
File Pages266 Page
-
File Size-