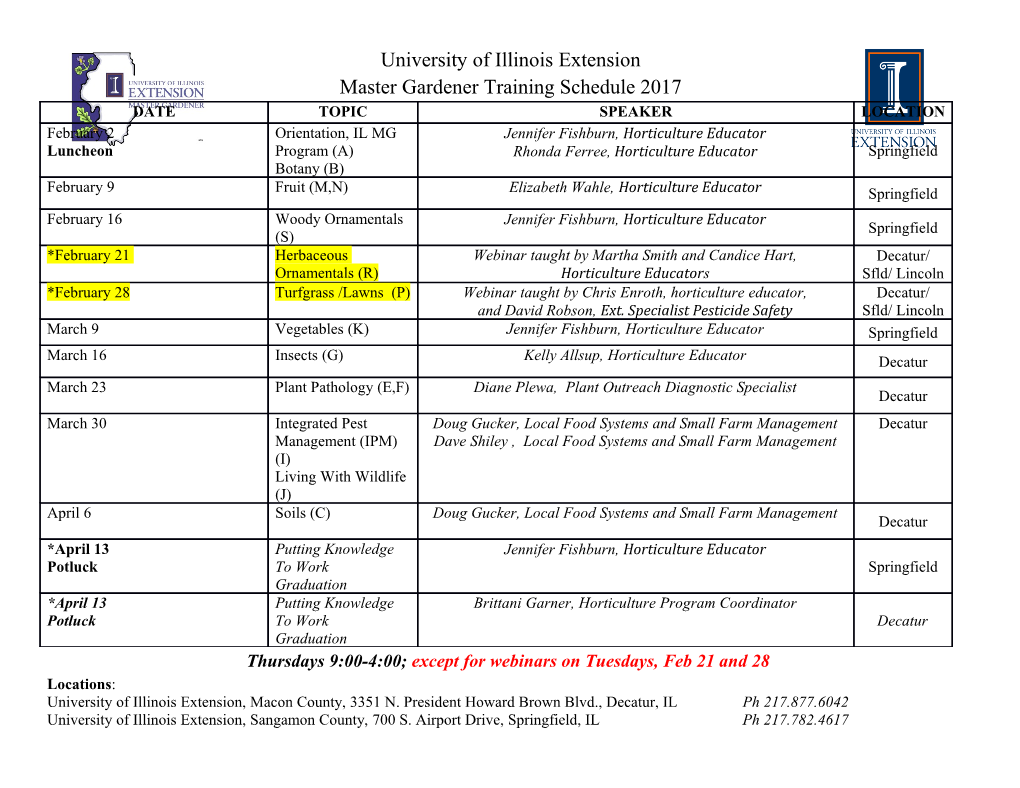
Letter of Notification for a Master of Science in Data Science Table of Contents ACADEMIC PROGRAM NAME .............................................................................................................3 DEGREE DESIGNATION .......................................................................................................................3 PROPOSED CIP CODES ........................................................................................................................3 PROPOSED CIP CODES TITLE ...............................................................................................................3 PROPOSED IMPLEMENTATION DATE ..................................................................................................3 ACADEMIC PROGRAM LIAISON (APL) NAME AND CONTACT INFORMATION ........................................3 BACKGROUND CONCERNING ACADEMIC PROGRAM DEVELOPMENT ...................................................3 PURPOSE AND NATURE OF ACADEMIC PROGRAM ..............................................................................4 ALIGNMENT WITH STATE MASTER PLAN AND INSTITUTIONAL MISSION ..............................................5 INSTITUTIONAL CAPACITY TO DELIVER THE PROPOSED ACADEMIC PROGRAM .....................................8 EXISTING PROGRAMS OFFERED AT PUBLIC AND PRIVATE TENNESSEE INSTITUTIONS ...........................8 FEASIBILITY STUDY .............................................................................................................................9 PROGRAM COSTS/REVENUES ........................................................................................................... 19 THEC FINANCIAL PROJECTION FORM ................................................................................................ 20 REFERENCES ....................................................................................................................................21 APPENDIX A: THEC Financial Projections Form .................................................................................. 22 APPENDIX B: Letters of Support ........................................................................................................ 28 2 ACADEMIC PROGRAM NAME Data Science DEGREE DESIGNATION Master of Science (MS) PROPOSED CIP CODES 11.0701 (Computer Science) PROPOSED CIP CODES TITLE Computer Science (11.0701) PROPOSED IMPLEMENTATION DATE Fall 2020 ACADEMIC PROGRAM LIAISON (APL) NAME AND CONTACT INFORMATION Dr. Robin Poston, [email protected], 901.678.5739 BACKGROUND CONCERNING ACADEMIC PROGRAM DEVELOPMENT “The coming century is surely the century of data” (Donoho, 2000). Data Science is emerging as a new, transformative paradigm in science and technology. With large volumes of data being generated every day from multiple sources (including business data, biomedical data, educational data, science data, engineering data, and personal data), the importance of systematic and rigorous approaches to understanding and putting these large volumes of data to good use is now well recognized. With this explosion of data, there is a significant demand for experts in industry, government, education, healthcare, etc., that have requisite skills to collect, process, and analyze data. Indeed, demand for Data Science master’s degrees has exploded in the last couple of years as indicated by the fact that the number of master’s degrees awarded in this area has quadrupled from around 5,000 to around 20,000 between 2016 and 2018 (see Figure 5). Our feasibility study, presented later, provides ample evidence on student interest and labor market needs in this area of Data Science including local and regional need, nationwide employer need, and future sustainable need and demand. Human expertise bottleneck. As noted, data is being generated at an unprecedented rate from varied sources such as social media, sensors, vehicles, smartphones, etc. We can collect a variety of data at high velocity and in large volumes using various data collection devices (sensors). For instance, we can deploy billions of sensors in cellphones and cars and have the information they collect sent to a digital storage server; similarly, millions of people observe and reported instantly traffic information using their cellphones – this information is then shared with all other drivers improving the overall efficiency of the 3 transportation system. Just to illustrate the volume of data being currently collected and available digitally, IBM estimates that 90 percent of the data in the world today has been created in the past two years. Exploiting the large and diverse data (big data) offers tremendous opportunities to improve processing, products, and services (Berman et al., 2018) as big data is assumed to carry insights and knowledge which would otherwise be inaccessible (Cao, 2017). For instance, in healthcare, big data holds the promise of reducing costs and increasing the quality of services (Brennan & Bakken, 2015; Frakt & Pizer, 2016). In other areas such as social and behavioral sciences (Lazer et al., 2009), agriculture (Kirkpatrick, 2019), law & justice (Quemy, 2017; also see the Data Driven Justice Initiative at https://www.naco.org/resources/signature-projects/data-driven-justice and the Big Data Social Justice Initiative at http://www.bigdatasocialjustice.com/social-justice-initiatives), or government (www.data.gov), there are similar opportunities. To deliver on those promises, we need to solve, above all, the human expertise bottleneck challenge by increasing the number of students trained in and knowledgeable of Data Science. Indeed, we can now scale data collection (by deploying at scale billions of data collection devices/sensors), storage (which is cheap and abundant), and processing (we can access millions of computers through cloud services). However, we cannot scale human expertise without a strategic, sustained, and concerted effort by all stakeholders. We face a human expertise bottleneck when it comes to big data. To meet this demand, universities around the country and the world have designed and been offering degrees in Data Science (for instance, there are more than 500 programs listed on the www.datascience.community) or establish entire institutes (see University of Rochester’s Goergen Data Science Institute and many others), departments, or schools (see University of Virginia’s School of Data Science - https://news.virginia.edu/content/uva-plans-new-school-data-science-120-million-gift-largest- university-history). The State of Tennessee and in particular the West Tennessee region will greatly benefit from a master’s level program in Data Science as indicated later by our feasibility study. The proposed Master’s in Data Science will address this acute need for Data Scientists in West Tennessee and beyond. PURPOSE AND NATURE OF ACADEMIC PROGRAM The purpose of the proposed Master of Science in Data Science (MS-DS) program is two-fold: 1) train students for positions in government and private agencies, industry, and research institutes, and 2) prepare students who plan to enter a doctoral program in Data Science and related fields. Among the outcomes of the MS-DS program we mention increase in State education attainment levels, meet the needs of economic, workforce, and research needs, and to increase degree production. The new MS-DS program will be hosted under the Dean of College of Arts and Sciences given its multi/inter disciplinary nature of its courses. 4 The nature of the program includes core courses in theoretical foundations of Data Science, i.e., a set of Computer Science and Statistics courses, and elective courses in discipline-specific quantitative analysis methods. The elective courses are clustered in specific disciplines such as Economics or Biomedical. This model has been proposed based on a market analysis of top programs (Columbia University, University of Michigan, University of Rochester) as well as programs from peer universities (University of Texas at El Paso, Southern Methodist University) around the country. The model offers foundational training as well as discipline-specific training in Data Science thus enabling students to become Data Scientists in specific areas such as Biology, Economics, Public Health, Government Policy, Logistics and Transportation, Inventory Management, Information Technology, inter alia, and a variety of other data- driven fields. This design should make the degree attractive to students who want to focus on Data Science methods in particular disciplines. It should be noted that one of the clusters is core Data Science which is meant to attract students who want to focus on core Data Science topics and possibly pursue a Ph.D. The target audience is college students who want to pursue Data Science careers with a focus on various disciplines ranging from political science to biology to public health to economics as well as professionals who want to acquire advanced Data Science skills in order to be able to advance their careers and competitiveness of their organizations by exploiting big data sets available nowadays with the goal of improving their organization’s processes, products, and services. Specifically, the MS-DS degree requires completion of 33 semester credit hours as follows: 15 credits from a set of core courses, 15 credits from a list of electives (with the recommendation that 9 credits must be from a concentration area), and 3 credits as master’s project. A Master’s Thesis option (6 credits) is also available in which case only
Details
-
File Typepdf
-
Upload Time-
-
Content LanguagesEnglish
-
Upload UserAnonymous/Not logged-in
-
File Pages46 Page
-
File Size-