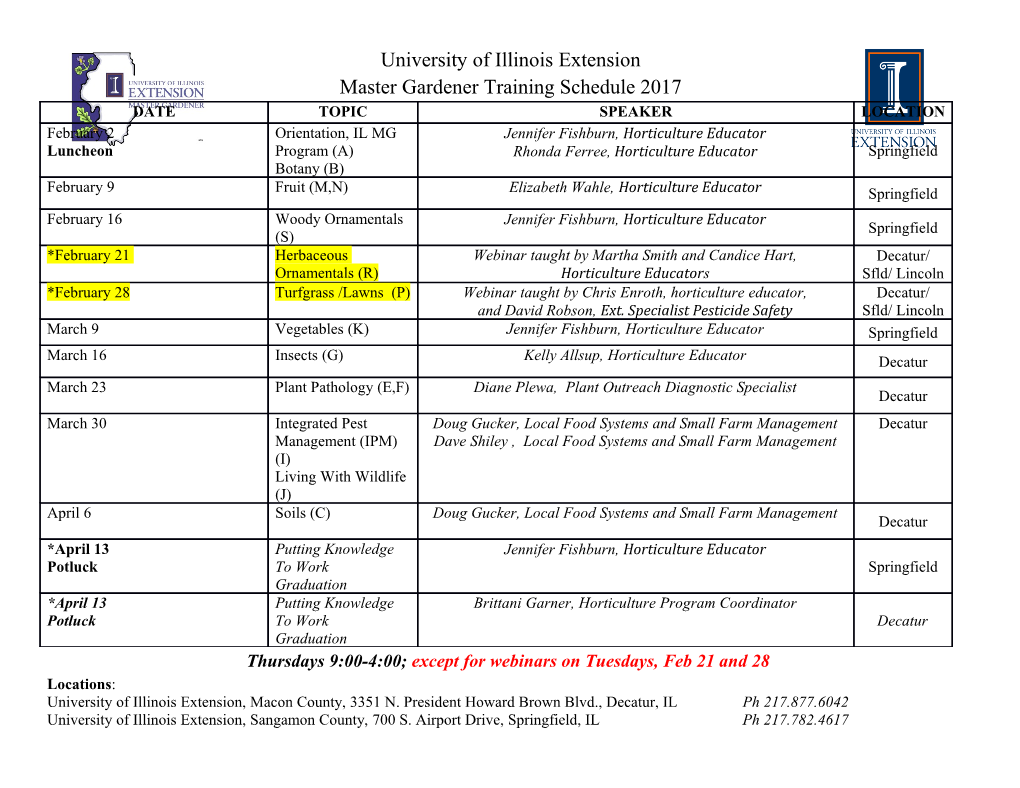
Pattern Recognition 46 (2013) 769–787 Contents lists available at SciVerse ScienceDirect Pattern Recognition journal homepage: www.elsevier.com/locate/pr Stratified sampling for feature subspace selection in random forests for high dimensional data Yunming Ye a,e,n, Qingyao Wu a,e, Joshua Zhexue Huang b,d, Michael K. Ng c, Xutao Li a,e a Department of Computer Science, Shenzhen Graduate School, Harbin Institute of Technology, China b Shenzhen Institutes of Advanced Technology, Chinese Academy of Sciences, China c Department of Mathematics, Hong Kong Baptist University, China d Shenzhen Key Laboratory of High Performance Data Mining, China e Shenzhen Key Laboratory of Internet Information Collaboration, Shenzhen, China article info abstract Article history: For high dimensional data a large portion of features are often not informative of the class of the objects. Received 3 November 2011 Random forest algorithms tend to use a simple random sampling of features in building their decision Received in revised form trees and consequently select many subspaces that contain few, if any, informative features. In this 1 September 2012 paper we propose a stratified sampling method to select the feature subspaces for random forests with Accepted 3 September 2012 high dimensional data. The key idea is to stratify features into two groups. One group will contain Available online 12 September 2012 strong informative features and the other weak informative features. Then, for feature subspace Keywords: selection, we randomly select features from each group proportionally. The advantage of stratified Stratified sampling sampling is that we can ensure that each subspace contains enough informative features for High-dimensional data classification in high dimensional data. Testing on both synthetic data and various real data sets in Classification gene classification, image categorization and face recognition data sets consistently demonstrates the Ensemble classifier Decision trees effectiveness of this new method. The performance is shown to better that of state-of-the-art Random forests algorithms including SVM, the four variants of random forests (RF, ERT, enrich-RF, and oblique-RF), and nearest neighbor (NN) algorithms. & 2012 Elsevier Ltd. All rights reserved. 1. Introduction bounded by the ratio of the average correlation between trees divided by the square of the average strength of the trees. Random forest (RF) builds a classification ensemble with a set For high dimensional data a large proportion of the features of decision trees that grow using randomly selected subspaces of may not be informative of the class of an object. The common data [1–5]. Experimental results have shown that random forest random sampling method may select many subspaces that do not classifiers can achieve a high accuracy in data classification include informative features. As a consequence, the decision trees [6,4,2]. Interest in random forests continues to grow with recent generated from these subspaces may suffer a reduction in their theoretical analyses [7–9] and applications research in bioinfor- average strength, thus increasing the error bounds for the RF. matics [10–14] and computer vision [15–19]. The main aim of this paper is to propose and develop a stratified A key step in building a RF is generating different subspaces of sampling method for feature subspace selection for generating the features at each node of each unpruned decision tree that makes decision trees of a RF. It is particularly relevant for high dimensional up the forest. Several subspace selection methods have been data. Our idea is to introduce a stratification variable to divide developed [5,1,4,2,3]. A simple random sampling of the available features into two groups: strong informative features and weak features is a common approach to selecting subspaces [2]. informative features. We then randomly select features from each The performance of a RF depends on the performance of each group, ensuring we have representative features from each group. decision tree, and the diversity of decision trees in the forest. This approach ensures that each subspace contains enough useful Breiman [2] formulated the overall performance of a set of trees features for classification purpose in high dimensional data. as the average strength and the average correlation between the In this paper we use both synthetic data sets and real world trees. He showed that the generalization error of a RF classifier is data sets from gene classification, image categorization and face recognition to demonstrate the proposed method’s effectiveness. The performance of the proposed method is shown to better that n Corresponding author. Tel./fax: þ86 755 2603 3008. of the current state-of-the-art algorithms including SVM, the four E-mail addresses: [email protected] (Y. Ye), [email protected] (Q. Wu), [email protected] (J. Zhexue Huang), variants of random forests (RF, ERT, enrich-RF, and oblique-RF), [email protected] (M.K. Ng), [email protected] (X. Li). and the kNN and naive Bayes algorithms. 0031-3203/$ - see front matter & 2012 Elsevier Ltd. All rights reserved. http://dx.doi.org/10.1016/j.patcog.2012.09.005 770 Y. Ye et al. / Pattern Recognition 46 (2013) 769–787 The remainder of the paper is organized as follows. In Section 2, random sampling the population are divided into smaller sub- we review random forests. In Section 3,wepresentthestratified groups called strata. Random sampling is then applied within each sampling method for feature subspace selection. In Section 4,we subgroup or stratum. present and discuss the experimental results on various data sets. One of the advantages of stratified sampling is that it can capture Conclusions and future work are presented in Section 5. key population characteristics. When we apply stratified sampling for feature subspace selection, a set of strong informative features forms a core stratum, and the weak informative features forms 2. Random forests another stratum. As discussed above it is advantageous to guarantee the inclusion of informative features in a subspace when construct- A RF model consists of an ensemble of decision tree models. The ing a decision tree. Adding weakly informative features will offer concept of building multiple decision trees was introduced by considerable diversity amongst the resulting decision trees. Williams [20].Ho[21] then developed the concept of taking a Based on these ideas, we introduce stratified random forests. The subspace of features when building each tree within the ensemble. method builds random decision trees using stratified feature sub- Breiman [2] then developed the RF algorithm as it is commonly space sampling for each node within the tree building process. This known today by introducing further randomness into the process. method is described in the following section where we also show that The common algorithm can be described as follows. Given a it is robust in subspace diversity and is computationally efficient. training data set X taken from a space S of N features: 3. Stratified feature subspace selection 1. Use bagging [22] to generate K subsets {X1,X2, ...,XK }by randomly sampling X with replacement. In this section, we first discuss the problem of a simple 2. For each data set Xk, use the CART [23] to build a decision tree. At each node in building a decision tree, randomly sample a random sampling method for feature subspace selection. Then subspace of p features (p5N) from S, and compute all possible we introduce a stratified sampling method. Finally, we develop a splits based on the p features. The best split (e.g., the largest new random forest algorithm, the stratified random forest (SRF). Gini measure) is used to continue with the divide and conquer process. Continue until a stopping criteria are met: i.e., all data 3.1. High dimensional data issue are pure with respect to the class, have identical values for each attribute, or the number of instances remaining in the The classification performance of a decision tree depends on data subset is less than nmin. the correlation of the selected features to the class feature. To 3. Combine the K unpruned trees h1ðX1Þ, h2ðX2Þ, y, hK ðXK Þ into a increase the strength of a tree we are required to select feature RF ensemble, and use a vote among the trees as the ensemble subspaces that contain features that are well correlated to classification decision. the class feature. However, for high dimensional data, there are usually relatively few features that have high a correlation to the An ensemble learner with excellent generalization accuracy has class feature. two properties: high accuracy of each component learner and high Suppose we have only H features that are informative (i.e., diversity in component learners. The theoretical and practical perfor- highly correlated to the class feature) for classification purposes. mance of ensemble classifiers is well documented [24,25]. The remaining NÀH features are then not informative. If a decision Breiman [2] employs two randomization procedures to tree is grown in a subspace of p features, often with p5N, then the achieve diversity. Sufficiently diverse trees can be constructed total number of possible subspaces is ! using randomly selected training samples for each of the indivi- N N! dual trees, and randomly selected subspaces of features for ¼ p ðNÀpÞ!p! splitting at each node within a tree. Geurts et al. [3] proposed to extremely randomize trees by randomizing the attribute The probability of selecting a subspace of p41 features without splitting threshold. Such randomization procedures were found informative features is given by to enhance the independence of individual classifiers in the ! NÀH ensemble and thus obtaining improved accuracy. H H p 1 1À ... 1À À À Random feature subspace sampling is the most common method p N N N N H p !¼ 1À to deliver diversity amongst decision trees due to its simplicity, N 1 p 1 N 1À ..
Details
-
File Typepdf
-
Upload Time-
-
Content LanguagesEnglish
-
Upload UserAnonymous/Not logged-in
-
File Pages19 Page
-
File Size-