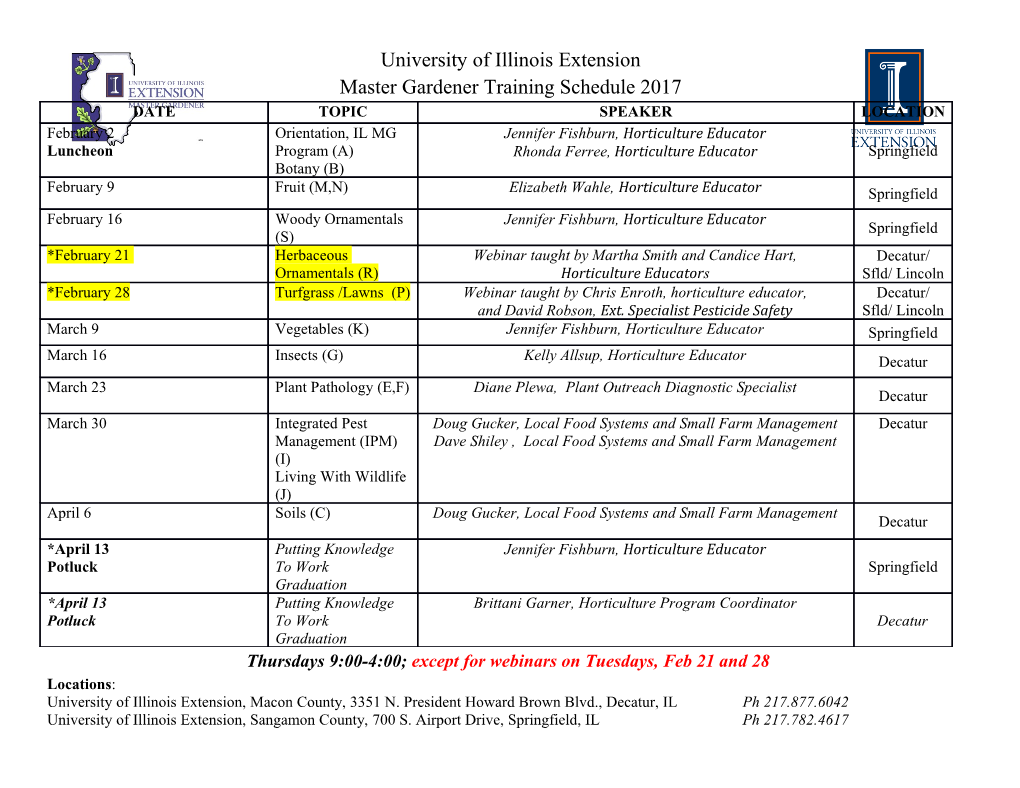
Guidelines for Assessment and Instruction in Statistics Education (GAISE) College Report 2016 Committee: Robert Carver (Stonehill College), Michelle Everson, co-chair (The Ohio State University), John Gabrosek (Grand Valley State University), Nicholas Horton (Amherst College), Robin Lock (St. Lawrence University), Megan Mocko, co-chair (University of Florida), Allan Rossman (Cal Poly – San Luis Obispo), Ginger Holmes Rowell (Middle Tennessee State University), Paul Velleman (Cornell University), Jeffrey Witmer (Oberlin College), and Beverly Wood (Embry-Riddle Aeronautical University) Citation: GAISE College Report ASA Revision Committee, “Guidelines for Assessment and Instruction in Statistics Education College Report 2016,” http://www.amstat.org/education/gaise. Endorsed by the American Statistical Association July 2016 1 Contents Committee: .......................................................................................................................................................... 1 Executive Summary ............................................................................................................................................ 3 Introduction ......................................................................................................................................................... 4 Goals for Students in Introductory Statistics Courses .................................................................................... 8 Recommendations ............................................................................................................................................. 12 Suggestions for Topics that Might be Omitted from Introductory Statistics Courses ............................... 23 References .......................................................................................................................................................... 25 APPENDIX A: Evolution of Introductory Statistics and Emergence of Statistics Education Resources . 28 APPENDIX B: Multivariable Thinking .......................................................................................................... 34 APPENDIX C: Activities, Projects, and Datasets .......................................................................................... 43 APPENDIX D: Examples of Using Technology ............................................................................................. 66 APPENDIX E: Examples of Assessment Items ............................................................................................ 104 APPENDIX F: Learning Environments ....................................................................................................... 131 2 EEExecutiveExecutive Summary In 2005 the American Statistical Association (ASA) endorsed the Guidelines for Assessment and Instruction in Statistics Education (GAISE) College Report. This report has had a profound impact on the teaching of introductory statistics in two- and four-year institutions, and the six recommendations put forward in the report have stood the test of time. Much has happened within the statistics education community and beyond in the intervening 10 years, making it critical to re-evaluate and update this important report. For readers who are unfamiliar with the original GAISE College Report or who are new to the statistics education community, the full version of the 2005 report can be found at http://www.amstat.org/education/gaise/GaiseCollege_full.pdf and a brief history of statistics education can be found in Appendix A of this new report. The revised GAISE College Report takes into account the many changes in the world of statistics education and statistical practice since 2005 and suggests a direction for the future of introductory statistics courses. Our work has been informed by outreach to the statistics education community and by reference to the statistics education literature. We continue to endorse the six recommendations outlined in the original GAISE College Report. We have simplified the language within some of these recommendations and re-ordered other recommendations so as to focus first on what to teach in introductory courses and then on how to teach those courses. We have also added two new emphases to the first recommendation. The revised recommendations are: 1. Teach statistical thinking. Teach statistics as an investigative process of problem-solving and decision- making. Give students experience with multivariable thinking. 2. Focus on conceptual understanding. 3. Integrate real data with a context and purpose. 4. Foster active learning. 5. Use technology to explore concepts and analyze data. 6. Use assessments to improve and evaluate student learning. This report includes an updated list of learning objectives for students in introductory courses, along with suggested topics that might be omitted from or de-emphasized in an introductory course. In response to feedback from statistics educators, we have substantially expanded and updated some appendices. We also created some new appendices to provide details about the evolution of introductory statistics courses; examples involving multivariable thinking; and ideas for implementing the GAISE recommendations in a variety of different learning environments. 3 Introduction Background Much has changed since the ASA endorsed the Guidelines for Assessment and Instruction in Statistics Education College Report (hereafter called the GAISE College Report) in 2005. Some highlights include: • More students are studying statistics. According to the Conference Board on Mathematical Sciences (CBMS) survey, 508,000 students took an introductory statistics course in a two- or four-year college/university in the fall of 2010, a 34.7% increase from 2005. More than a quarter (27.0%) of these enrollments were at two-year colleges 1. Nearly 200,000 students took the Advanced Placement (AP) Statistics exam in 2015, an increase of more than 150% over 2005. In addition, many high school students took the AP course without taking the exam or took a non-AP statistics course. At the undergraduate level, the number of students completing an undergraduate major in Statistics grew by more than 140% between 2003 and 2013 and continues to grow rapidly 2. • Many students are exposed to statistical thinking in grades 6 – 12 , because more state standards include a considerable number of statistical concepts and methods. Many of these standards have been influenced by the GAISE PreK – 12 report developed and endorsed by the ASA 3. In particular, the Common Core 4 includes standards on interpreting categorical and quantitative data and on making inferences and justifying conclusions. • The rapid increase in available data has made the field of statistics more salient. Many have heralded the flood of information now available. The Economist published a special report on the “data deluge” in 2010 5. Statisticians such as Hans Rosling and Nate Silver have achieved celebrity status by demonstrating how to garner insights from data 6. • The discipline of Data Science has emerged as a field that encompasses elements of statistics, computer science, and domain-specific knowledge 7. Data science has been described as the interplay between computational and inferential thinking 8. It includes the analysis of data types such as text, audio, and video, which are becoming more 1 http://www.ams.org/profession/data/cbms-survey/cbms2010-Report.pdf 2 http://www.amstat.org/education/curriculumguidelines.cfm 3 http://www.amstat.org/education/gaise/GAISEPreK-12_Full.pdf 4 http://www.corestandards.org/ 5 http://www.economist.com/printedition/2010-02-27 6 Silver, N. (2012) The Signal and the Noise: Why So Many Predictions Fail—but Some Don’t. New York: Penguin Books. Also see http://www.fivethirtyeight.com and http://www.gapminder.org. 7 http://magazine.amstat.org/blog/2015/10/01/asa-statement-on-the-role-of-statistics-in-data-science/ 8 Jordan, M. (2016) Computational Thinking and Inferential Thinking: Foundations of Data Science eCOTS 2016. Also see at https://www.causeweb.org/cause/ecots/ecots16/keynotes/jordan. 4 prevalent. There has been a parallel development of “analytics” as the study of extracting information from big data—particularly with business and governmental applications. • More and better technology options for education have become widely available . These include course management systems, automated homework systems, technology for facilitating discussion and engagement, audience response systems, and videos now used in many courses. Applets and other applications, such as Shiny apps coded in the R programming language, that are designed to explore statistical concepts have come into widespread use. Many general-purpose statistical packages have developed functions specifically for teaching and learning. • Alternative learning environments have become more popular. These include online courses, hybrid courses, flipped classrooms, and Massively Open Online Courses (MOOCs). Many of these environments may be particularly helpful for supporting faculty development. • Some have called for an update to the consensus introductory statistics curriculum to account for the rich data that are available to answer important statistical questions. • Innovative ways to teach the logic of statistical inference have received increasing attention. Among these are greater use of computer-based simulations and the use of resampling methods (randomization tests and bootstrapping) to teach concepts
Details
-
File Typepdf
-
Upload Time-
-
Content LanguagesEnglish
-
Upload UserAnonymous/Not logged-in
-
File Pages141 Page
-
File Size-