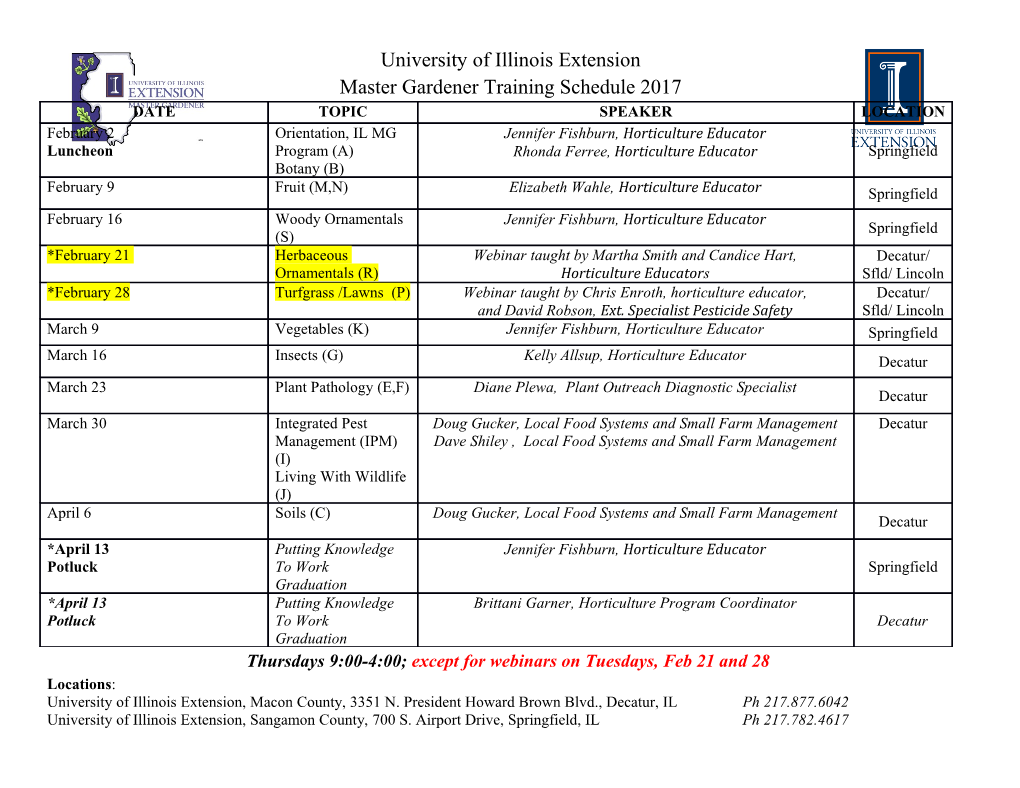
Rigorous Cluster Analyses For Prospective Player Evaluation In The National Football League Max Isaac Mulitz1 Brown University December 2015 I wish to express my appreciation to my thesis advisor, Professor Francesco Di Plinio, for his guidance and support throughout the research process and to Professor Mark Dean who served as my second reader. I would also like to thank Dr. Kevin Dayaratna for his voluntary guidance and insights in discussing the issues addressed in this thesis. I also wish to thank those coaches and advisors that directed and assisted me toward this interest including: Coach Nelson Burton, Coach Tom Green, Katherine Russell, Frank Costello, Coach Phil Estes and Brown University Football, and Coach Chip Kelly and the Philadelphia Eagles Organization. 1 Abstract This study uses principal component as well as k-means cluster analysis to evaluate prospects who enter the National Football League (NFL) draft. We also explore whether such analysis creates the opportunity to take advantage of inefficiencies in the NFL draft as a market. We find that for offensive tackles, both speed and explosiveness-based athleticism have a positive relationship with career performance, even after discounting for draft position. Our results have useful managerial implications, suggesting that many NFL managers currently undervalue athleticism in the offensive tackle position when selecting players in the draft. 2 1. Introduction The National Football League (NFL) is a multi-billion dollar business (Keenan, 2014). In principle, team quality influences the size of the teams fan base, which in turn sets the market for television rights, ticket sales and other moneys the team can make selling memorabilia, etc. Because of the hyper-competitive nature of the NFL, star players make close to 20 million per year and team salaries easily surpass $100 million per year. Throughout the year, NFL teams are constantly assessing potential as well as current players for their rosters. Scouts, coaches, statisticians, general managers, and, occasionally, owners participate in the assessment and acquisition of players. Although there are multiple routes to becoming a player for the NFL, the predominant path is from a National Collegiate Athletic Association (NCAA) Division I college team to the NFL Draft. Once drafted, the selectees must then make it through training camp without being cut from the roster and generally perform well during their initial game experiences. Each team organization has its own approaches for scouting and evaluate future players. However, included in the mix of assessment tools are: college records and statistics; results of personal discussions and interviews with candidates and their coaches, and information collected through exercises known as “combines.” During combines, players are interviewed, subjected to medical, psychological, and intelligence 3 testing and participate in a host of activities designed to assess their athleticism. One attribute of the combines is that they produce a single set of data and information on each participating player that is available to each NFL team. In addition, unlike the data from each candidate’s college experience, each player participating in the combine is subjected to the same battery of tests in the same environment, at the same time, which gives the data greater utility for comparing players with each other: “The combine requires the players to display their talents by performing standardized tests under controlled conditions, thereby creating fair and unbiased assessment conditions.” (Kuzmits, 2008). A detailed explanation of the Combine and the NFL Draft appear at Appendix I. 2. Previous Work The Harvard Sports Analytics Collective has written two papers evaluating the effect of Combine performance on NFL success and found very little correlation between the two (Meers, 2015). The Harvard study, however, only looked at raw Combine data. Many members of the sports analytics community believe that some adjustment to the raw Combine data yields a greater correlation between Combine results and NFL performance. One example of such a study is Shawn Siegele’s work on agility score and running back elusiveness, in which he finds a relationship between the agility metrics and rushing yards before contact (Siegele, 2012). Similarly, Lyndon Plothow finds that the Combine results measure “accurately skills and characteristics that are important for NFL football players,” yet, he recognizes that “[i]nterviews, position specific drills, and intelligence tests all inform a team’s decision to draft a player.” (Plothow, 2010). Another example is the analysts for Football Outsiders invented Speed Score in 2008, 4 which they used to demonstrate a stronger relationship between weight adjusted speed and running back performance than could be shown using a non-weight adjusted speed measurement. (Baier, 2015). Recently, certain teams, including the Seattle Seahawks, have been using SPARQ, which essentially takes raw combine variables as data and transforms it into a single final athleticism score. (Kelly, 2015). Similarly, the Philadelphia Eagles also employ SPARQ in their assessment efforts (Mengels, 2015). As discussed above, numerous studies suggest relationships between NFL combine scores and career performance. Each of these studies are based on some form of regression-based statistical analysis. It is this author’s view that regression analysis provides a reasonable measure of the correlation between combine and career performance. However, mathematical tools are available that present greater detail and precision in the analysis of correlations. Unlike regression analysis, Principal Component Analysis (PCA) and k-means Cluster Analysis not only measure correlation, but also explain the relevant factors and provide or suggest a causal correlation. Accordingly, this paper uses PCA and k-means, and therefore yields more precise analyses, which provide greater certainty to the overall conclusions drawn from the data. 3. Data NFL Combine Data is widely available going back to 1999 online. (NFL Combine Results, 2015). The sample data does not include information after 2011 because it is not possible to assess career performance of players that have not been in the league for more than three years. Accordingly, this paper used Combine data for the 1999 through 2011 seasons. 5 Our dependent measure is Career Approximate Value (AV) (Sports Reference, 2015). Although approximate value is not a perfect representation for career value, it is widely accepted as a reasonable proxy and, as a group, higher AV players playing a specific position have had better careers than lower AV players. 4. Methodology: The analysis employs multiple methodologies to determine optimal methods of evaluating positions. First, PCA is used to find which skills yield the most direct correlation to NFL performance measured according to AV. Then the application of cluster analysis is used to determine whether there are player types or athletic profiles at different positions that outperform the other positions. In addition to the combine numbers, we use speed score, which is a measurement of weight adjusted speed, and Explosion Score, which is the author’s own version of Weight Adjusted Jumping, which has been demonstrated to be important for at least some positions. (Waldo, 2011). As discussed above, Football Outsiders demonstrated the strong relationship between Speed Scores and running back performance in comparison to speed or weight alone. Waldo showed that the predictive ability of weight-adjusted jumping scores exceeded that of just considering jumping scores. Greater weight may impede both speed and jumping ability. Therefore, adjusting speed and jumping metrics for weight produces better indications of future performance. After performing the cluster analysis, we assess the performance of players, both before and after making an adjustment for draft position. The reason to adjust for draft position to some degree is because higher drafted players are given a greater opportunity to succeed and also because we are looking to see if players in a certain tier of athleticism 6 who are available later in the draft than their peers still actually outperform their expectation. To adjust for draft position, we compare the player’s data with the expected career AV of someone taken with at the same draft position. The expected Career AV will be tested using an online calculator (Rotoviz, Trade Calculator, 2015), which uses regression analysis. Because significant work has already been done on the quarterback, wide receiver, and running back positions, this work focuses on the offensive and defensive lines, which is somewhat uncharted territory. A better understanding of positions on the offensive and defensive lines should be of significant value to teams because the ultimate success of quarterbacks, wide receivers and running backs is largely dependent on the capabilities and performance of these supporting players. 5. Hypothesis We hypothesize that the relationship between Combine performance and NFL career performance will vary depending on offensive or defensive line position. For example, there is an expectation that basic athleticism is a measurable asset for offensive tackles. On the other hand, guards and centers, who require less athleticism, are expected to show greater explosive strength, but less agility than offensive tackles. For the defensive line, the success of interior players should, in principle, be
Details
-
File Typepdf
-
Upload Time-
-
Content LanguagesEnglish
-
Upload UserAnonymous/Not logged-in
-
File Pages48 Page
-
File Size-