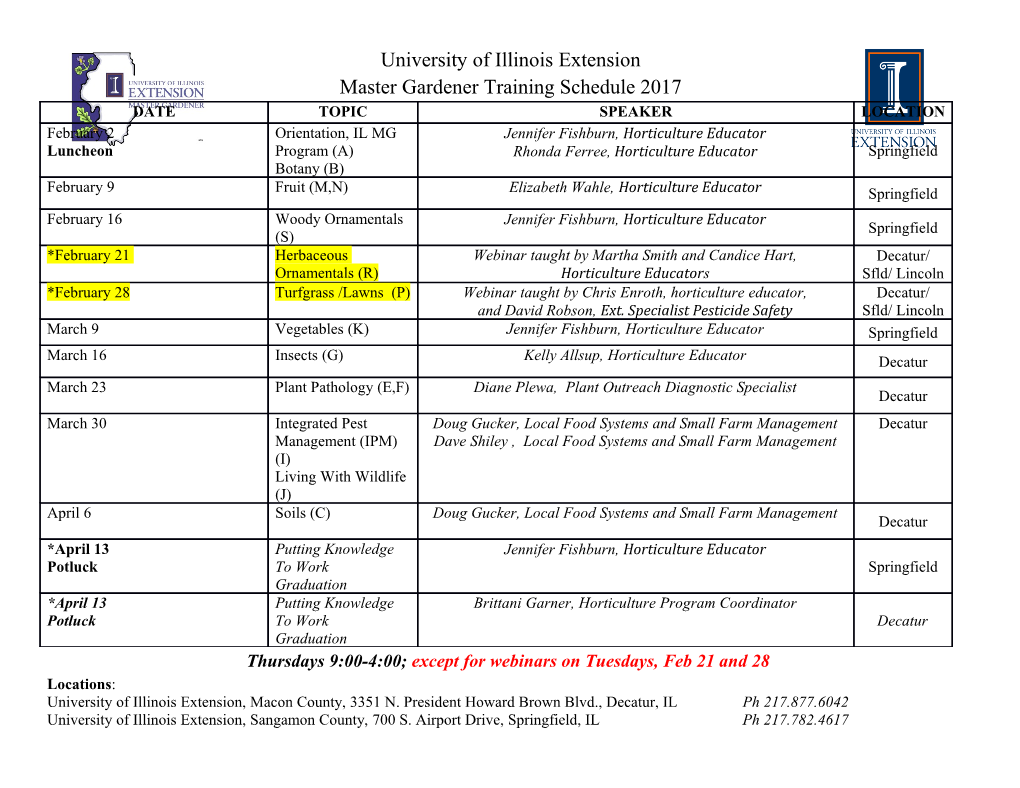
Evaluating Badland Susceptibility in Basilicata, Italy through the Integration of GIS and Multicriteria Decision Analysis Sean Leipe School of Geography and Earth Sciences, McMaster University 1. Introduction 2. Data and Methods (cont.) 3. Results (cont.) Three forms of MCDA were used as decision rules to combine The most visual similarity between maps was found between Badlands are spectacular forms of intense and accelerated gully the predisposing factor layers and create susceptibility maps. the AHP and Ideal Point Method models. Quantitative erosion that affect many parts of Italy [1, 2] and are common in differences in the maps are highlighted in Figure 4 by showing arid and semiarid areas such as the southern Basilicata region. The Analytical Hierarchy Process (AHP) uses a hierarchal which areas were classified as High or Very High for each map. These can be separated into two distinct erosional features: structure and pairwise comparison of criteria to establish their 23.7% of the total study area was included by at least 2 models, calanchi and biancane, where calanchi-type badlands are weights [14]. For this map, each criterion was ranked and while the Ideal Point Method gave the highest exclusive area of unvegetated, intensely dissected, knife-edged landforms that weighted through a pairwise comparison matrix, with a 343.2 km2. The Ideal Point model also gave the most highly or typically occur on 40-60° slopes made up of poorly consistency ratio of 0.057 showing that these judgements were very highly susceptible area, at 52.7% of the Basilicata region. consolidated/cemented materials [3, 4]. Though the calanchi reasonable. The weighted standardized layers were then added are visually spectacular, they are perceived as harsh and infertile using the Raster Calculator to create the susceptibility map. landscapes with limited to no agricultural potential and lead to The Ideal Point method ranks alternatives by their separation strong land degradation [4,5]. from an “ideal point”, which represents the most desirable level of each criterion under consideration [15]. For this map, the criteria were standardized and weighted through the same pairwise comparison matrix used for the AHP. The relative closeness between each pixel and its “ideal point” was then computed across the study area using the Raster Calculator and used to create the susceptibility map. Fuzzy Set Analysis involves standardizing each criterion using a function that determines how likely it is that a given pixel belongs to the set “suitable”, with a fully suitable pixel being given a value of 1 [16]. Using the Fuzzy Weighted Overlay (FWO) method [15], map layers were standardized through the Fuzzy Membership tools in ArcMap 10.4.1., weighted according to the pairwise comparison matrix, and added together in the Raster Calculator to create the susceptibility map. To validate the predictive capabilities of a susceptibility model, the maps created by it must be compared with known badland locations [7]. This badland inventory was created by identifying calanchi landscapes through Landsat 8 pan-sharpened imagery [17], and was used to extract the values overlaying it from each Figure 1: Location of the study area along with a susceptibility map. Areas used to evaluate contributing factors Figure 4: Differences between maps in areas calanchi landscape within the Aliano municipality [6] were used as a training set to determine the success rates of the identified as being highly or very highly susceptible models, while landscapes found after completing the models Prediction rate and success rate curves were plotted using The purpose of this study is to create 3 maps that evaluate were used as a validation set to determine their prediction rates. susceptibility values extracted from the badland inventory, and susceptibility of calanchi erosion in the Basilicata region using were used for ROC analysis. An area under curve (AUC) value of different forms of multicriteria decision analysis (MCDA), and 1 indicates a perfect model with ideal fit, while a value of 0.5 assess and compare their results and effectiveness using Training Validation would indicate an inaccurate model with random fit [7]. prediction rate and success rate curves along with Receiver Set Set Operating Characteristics (ROC) analysis. 2. Data and Methods Through identification of calanchi landscapes using satellite Prediction Rate Prediction Rate Success Rate Success Rate imagery and previous works [1, 3, 6, 7, 8, 9, 10], eight causal Figure 2: Landsat 8 imagery of the badland inventory, split factors were identified as contributing to calanchi into the training set (left) and validation set (right) encroachment and converted to operational data layers in Analytical Hierarchy Process ArcGIS 10.4.1. These factors – listed in order of their 3. Results Prediction AUC = 0.8135 importance to calanchi erosion – are shown in Table 1. Success AUC = 0.8484 Once the factor layers were combined using their respective Ideal Point Method Table 1: Predisposing factor layers and decision rules, they were then classified into 5 levels of Prediction AUC = 0.7894 rationale behind their inclusion in the model susceptibility using the Equal Interval method so that values of Success AUC =0.8149 0 – 0.2 were considered very low, 0.2 – 0.4 as low, 0.4 – 0.6 as Prediction Rate Data Layer Rationale Success Rate Fuzzy Weighted Overlay moderate, 0.6 – 0.8 as high, and 0.8 – 1.0 as very high. On Prediction AUC = 0.6879 Lithology Calanchi are typically found in average, 43% of the area was found to be high or very high. Success AUC = 0.7058 unconsolidated or poorly Figure 5: Prediction rate and success rate curves consolidated fine sediments [3, 7] generated for each model, used for ROC analysis Land Cover Human activities are a key factor in calanchi development, and can be 4. Conclusion determined through the land use A combination of GIS and MCDA was used to create 3 classification of the area [2] susceptibility maps of calanchi erosion in Basilicata, Italy. These Soil Type Calanchi mainly develop in fine- were validated through ROC analysis, which showed that the grained, highly dispersive soils [3, AHP model had the best predictive capability. On average, 43% of the study area was found to be highly or very highly 8] susceptible to badland encroachment, which should be Slope Gradient Several studies have found that considered in any future prevention or remediation strategies. slope gradient is an important control on calanchi erosion [2, 3, 7, 5. Literature Cited 8, 9] 1. Alexander, D. E. (1980). Calanchi-Accélerated erosion in Italy. Geography, 65(2), 95–100. 2. Buccolini, M., & Coco, L. (2013). MSI (morphometric slope index) for analyzing activation and evolution of calanchi in Italy. Geomorphology, 191, 142–149. Aspect Calanchi landforms tend to develop 3. Clarke, M. L., & Rendell, H. M. (2006). Process-form relationships in Southern Italian badlands: Erosion rates and implications for landform evolution. Earth Surface Processes and Landforms, 31(1), 15–29. on S to SW-facing scarps [3, 7] 4. Phillips, C. P. (1998). The badlands of Italy: a vanishing landscape? Applied Geography, 18(3), 243–257. 5. Valentin, C., Poesen, J., & Li, Y. (2005). Gully erosion: Impacts, factors and control. Catena, 63(2–3), 132–153. 6. Svelto, Anna. Basilicata – I calanchi di Aliano (Matera). Fame di Sud, 2016. Length Slope The LS Factor considers the effect 7. Bianchini, S., Del Soldato, M., Solari, L., Nolesini, T., Pratesi, F., & Moretti, S. (2016). Badland susceptibility assessment in Volterra municipality (Tuscany, Italy) by means of GIS and statistical analysis. Environmental Earth Sciences, 75(10), 889. 8. Conforti, M., Buttafuoco, G., Rago, V., Aucelli, P. P. C., Robustelli, G., & Scarciglia, F. (2016). Soil loss assessment in the Turbolo Factor of topography on erosion [11] catchment (Calabria, Italy). Journal of Maps, 12(5), 815–825. 8. Farifteh, J., & Soeters, R. (2006). Origin of biancane and calanchi in East Aliano, southern Italy. Geomorphology, 77(1–2), 142–152. 9. Moretti, S., & Rodolfi, G. (2000). A typical “calanchi” landscape on the Eastern Apennine margin (Atri, Central Italy): Stream Power The SPI is a measure of the erosive Geomorphological features and evolution. Catena, 40(2), 217–228. 10. Pulice, I., Cappadonia, C., Scarciglia, F., Robustelli, G., Conoscenti, C., De Rose, R., … Agnesi, V. (2012). Geomorphological, chemical Index power of flowing water [12] and physical study of “calanchi” landforms in NW Sicily (southern Italy). Soil Sci SSoc Am J, 50, 1294-1298 11. Moore, I. D., Burch G.J. (1986). Physical basis of the length-slope factor in the universal soil loss equation.. Hydrological Processes, 5(1), 3–30 Curvature Plan curvature is perpendicular to 12. Moore, I. D., Grayson, R. B., & Ladson, a R. (1991). Digital Terrain Modeling : A Review of Hydrological Geomorphological and Biological Applications. Hydrological Processes, 5(1), 3–30. (Planform) the erosional slope and emphasizes 13. Pourghasemi, H. R., Pradhan, B., & Gokceoglu, C. (2012). Application of fuzzy logic and analytical hierarchy process (AHP) to landslide susceptibility mapping at Haraz watershed, Iran. Natural Hazards, 63(2), 965–996. http 14. Jankowski, P. (2007). Integrating geographical information systems and multiple criteria decision-making methods. International the convergence and divergence of Journal of Geographical Information Systems, 9(3), 252–273 Figure 3: Susceptibility maps generated using the 15. Malczewski, J. (1999). GIS and Multicriteria Decision Analysis. John Wiley, New York flow along it [13] 16. Jiang, H., & Eastman, J. R. (2000). Application of fuzzy measures in multi-criteria evaluation in GIS. International Journal of Geographical Information Science, 14(2), 173–184. different MCDA methods, classified using Equal Interval 17.NASA Landsat Program (2016). Landsat 8 OLI, 15m resolution. United States Geological Survey, Sioux Falls, captured August 8th, 2016. .
Details
-
File Typepdf
-
Upload Time-
-
Content LanguagesEnglish
-
Upload UserAnonymous/Not logged-in
-
File Pages1 Page
-
File Size-