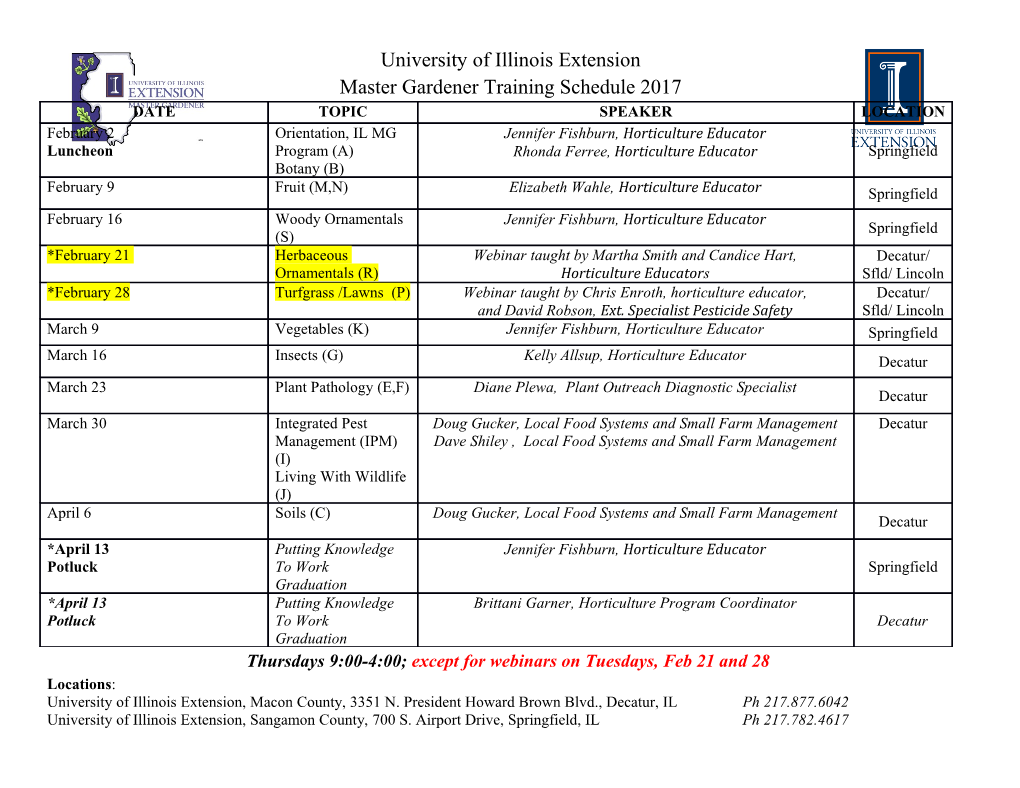
Overview Estimating Phylogenetic Trees • Introduction • Definitions Inge Jonassen, • Tree building methods Dept. of Informatics – Clustering (UPGMA) University of Bergen – Neighbour Joining • Evaluating trees • Practical usage Darwin: “Origin of the species” Tree • Tree – nodes – edges – Exactly one path from each node to every other node (no cycles) 1 Rooted/unrooted Tree Node degrees • Rooted tree – there is a special node called a root from which there is Degree of a node: number of edges coming in/going out a unique path leading to every other node • Unrooted 1 – no such node 2 Rooted tree Un-rooted tree 1 2 1 4 1 Taxonomic Unit • Taxonomic Unit - gene/species/.. represented by a node in the tree • Operational Taxonomic Unit - gene/species represented by a leaf in the tree - these are the genes/species under comparison A-I: Taxonomic Units A-E: Operational Taxonomic Units Bifurcating tree Rooted tree Un-rooted tree • A node is bifurcating if it has only two immediate descendant lineages – in a rooted tree;L an internal node has exactly two children – in an unrooted tree; an internal node has degree 3. A and B are children of H, H and G are children of I, C and F are children of G, D and E are children of F. 2 Estimate tree Brute force impossible • Goal: • For n OTUs, the number of different topologies is – Find tree which shows the history of evolution of a set of genes/species/… • Not possible to observe • Can estimate based on today’s species/genes • For n=20, there are 221,643,095,476,699,771,875 – Need model of evolution different topologies – Find tree that is likely to have produced today’s species/genes under the model • Cannot look at all! Tree Building Methods Distance based Distance matrix • Distance based Alignment 1 1 2 3 4 5 6 7 – calculate measure of distance between each pair of 2 genes 3 1 4 2 5 – use distances to find tree 6 3 • Character based 7 4 5 – use characters (bases/amino acids) when building the 6 tree 7 7 2 1 65 3 4 Tree Agglomerative UPGMA Clustering Method • Distance based • Different clustering methods differ in how they • Outline: define the distance between two clusters. – Let each unit be a cluster • UPGMA uses – Join the two clusters u,v closest together - • Let them be a new cluster (u,v) • Build tree for (u,v) by letting the trees corresponding to u and v be subtrees in a new tree for (u,v). – Keep going until only one cluster remains where nu (nv) is the number of input sequences (leaves in the tree rooted by u (v) 3 WPGMA One problem with XPGMA • Assumes that evolution happens with constant rate • WPGMA uses (molecular clock) • UPGMA assigns equal weight to each original sequence-sequence distance. • WPGMA does not - therefore it is called weighted Neighbour Joining (NJ) • Does not assume a constant molecular clock • Starts with a star tree where all OTUs are linked to a central node: • Each pair of OTUs are evaluated for being clustered together, for example 1 and 2: N i=3 • For each pair the sum of all lengths in the resulting tree is calculated • The pair giving the lowest sum is chosen - in the continuation the pair is considered as one OTU • This is repeated. 4 NJ vs UPGMA Minimum Evolution • Note that in NJ the pair of OTUs is chosen that • The tree with minimum sum of branch lengths is the gives the lowest sum of branch lengths in the minimum evolution tree. resulting tree. • Note that NJ “takes one step at a time” and need not produce a tree which gives minimum evolution • In UPGMA the pair of closest OTUs are chosen • Cannot look at all trees. not taking into account the rest of the tree. • One way: • UPGMA does not allow for rate variation among – make tree using NJ branches. – calculate sum of branch lengths of NJ tree and for topologically similar trees Character based methods Character based methods Alignment • Maximum Parsimony 1 2 – find evolutionary tree requiring the minimum number 3 of evolutionary changes to explain the differences in 4 5 the OTUs 6 7 • Maximum Likelihood – find model (including tree) that gives the highest likelihood of producing the observed sequences - under the defined model 7 2 1 6 5 3 4 • Both are very time consuming, but accurate Tree Statistical Testing: Example result Bootstrapping • Test the reliability of a tree T produced from an alignment A (with n columns). • Repeat x (e.g., 100) times – make pseudo-alignment A’ by picking (with replacement) n arbitrary columns from A – Estimate tree for A’: T’ – For each subtree in T: check if it is found in T’ – Record for each subtree in T for how many pseudo- In 90% of the trees produced from pseudo-alignments, the sub-tree (1,2) alignments the resulting trees contained the same was found subtree. 5 Example Practical use > HBA_HORSE VLSAADKTNV KAAWSKVGGH AGEYGAEALE RMFLGFPTTK TYFPHFDLSH GSAQVKAHGK KVGDALTLAV GHLDDLPGAL SNLSDLHAHK LRVDPVNFKL LSHCLLSTLA VHLPNDFTPA VHASLDKFLS SVSTVLTSKY R • Include as many sequences as possible • ClustalX was >HBB_HORSE VQLSGEEKAA VLALWDKVNE EEVGGEALGR LLVVYPWTQR FFDSFGDLSN PGAVMGNPK used to align KAHGKKVLHS FGEGVHHLDN LKGTFAALSE LHCDKLHVDP ENFRLLGNVL VVVLARHFGK – make sure they are all homologous DFTPELQASY QKVVAGVANA LAHKYH >MYG_PHYCA 7 globin VLSEGEWQLV LHVWAKVEAD VAGHGQDILI RLFKSHPETL EKFDRFKHLK TEAEMKASED • Make an accurate alignment LKKHGVTVLT ALGAILKKKG HHEAELKPLA QSHATKHKIP IKYLEFISEA IIHVLHSRHP sequences GDFGADAQGA MNKALELFRK DIAAKYKELG YQG – accurate - aligned residues/bases have evolved from >GLB5_PETMA PIVDTGSVAP LSAAEKTKIR SAWAPVYSTY ETSGVDILVK FFTSTPAAQE FFPKFKGLTT same residue/base in common ancestor ADQLKKSADV RWHAERIINA VNDAVASMDD TEKMSMKLRD LSGKHAKSFQ VDPQYFKVLA AVIADTVAAG DAGFEKLMSM ICILLRSAY – can be hand-edited >LGB2_LUPLU GALTESQAAL VKSSWEEFNA NIPKHTHRFF ILVLEIAPAA KDLFSFLKGT SEVPQNNPEL QAHAGKVFKL VYEAAIQLQV TGVVVTDATL KNLGSVHVSK GVADAHFPVV KEAILKTIKE • Select the part of the alignment to be used as input VVGAKWSEEL NSAWTIAYDE LAIVIKKEMN DAA >HBA_HUMAN to the phylogeny program - remove VLSPADKTNV KAAWGKVGAH AGEYGAEALE RMFLSFPTTK TYFPHFDLSH GSAQVKGHGK KVADALTNAV AHVDDMPNAL SALSDLHAHK LRVDPVNFKL LSHCLLVTLA AHLPAEFTPA VHASLDKFLA SVSTVLTSKY R – gappy regions >HBB_HUMAN VHLTPEEKSA VTALWGKVNV DEVGGEALGR LLVVYPWTQR FFESFGDLST PDAVMGNPKV – unreliably aligned regions KAHGKKVLGA FSDGLAHLDN LKGTFATLSE LHCDKLHVDP ENFRLLGNVL VCVLAHHFGK EFTPPVQAAY QKVVAGVANA LAHKYH Clustal X guide tree Resulting alignment NJ tree from alignment The two together Guide Tree From Alignment 6 How to Build Good Trees Programs used • Large number of OTUs • ClustalX • Large number of characters • Avoid characters prone to convergence • Drawtree from the phylip package – GC, codon usage, dinucleotides • Avoid rapidly evolving characters – GC, third positions, variable a.a.’s • Analyze only homologous characters in different OTUs – alignments must be good • For gene trees, identify orthologs and paralogs From Jonathan Eisen, TIGR Evolutionary Functional Prediction How to Build Good Gene Trees EXAMPLE A METHOD EXAMPLE B 2A CHOOSE GENE(S) OF INTEREST 5 1 3 4 3A 2 2B 5 1A 2A 1B 6 • Identify all homologs of gene of interest 3B IDENTIFY HOMOLOGS • Align carefully ALIGN SEQUENCES • EXCLUDE regions of ambiguous alignment 1A 2A 3A 1B 2B 3B 1 2 3 4 5 6 • EXCLUDE hypervariable regions CALCULATE GENE TREE • EXCLUDE gaps Duplication? 1 2 3 4 5 6 • Use multiple phlyogenetic methods 1A 2A 3A 1B 2B 3B OVERLAY KNOWN • Use methods that allow for rate variation among branches FUNCTIONS ONTO TREE – neighbor-joining not UPGMA Duplication? 1 2 3 4 5 6 • Use methods that incorporate mutation/substitution biases 1A 2A 3A 1B 2B 3B INFER LIKELY FUNCTION – ts-tv, PAM OF GENE(S) OF INTEREST Ambiguous • Estimate statistical support for patterns Duplication? Species 1 Species 2 Species 3 – likelihood, bootstrapping 1A1B 2A 2B 3A 3B 1 2 3 4 5 6 ACTUAL EVOLUTION (ASSUMED TO BE UNKNOWN) From Jonathan Eisen, TIGR Duplication From Jonathan Eisen, TIGR 7.
Details
-
File Typepdf
-
Upload Time-
-
Content LanguagesEnglish
-
Upload UserAnonymous/Not logged-in
-
File Pages7 Page
-
File Size-