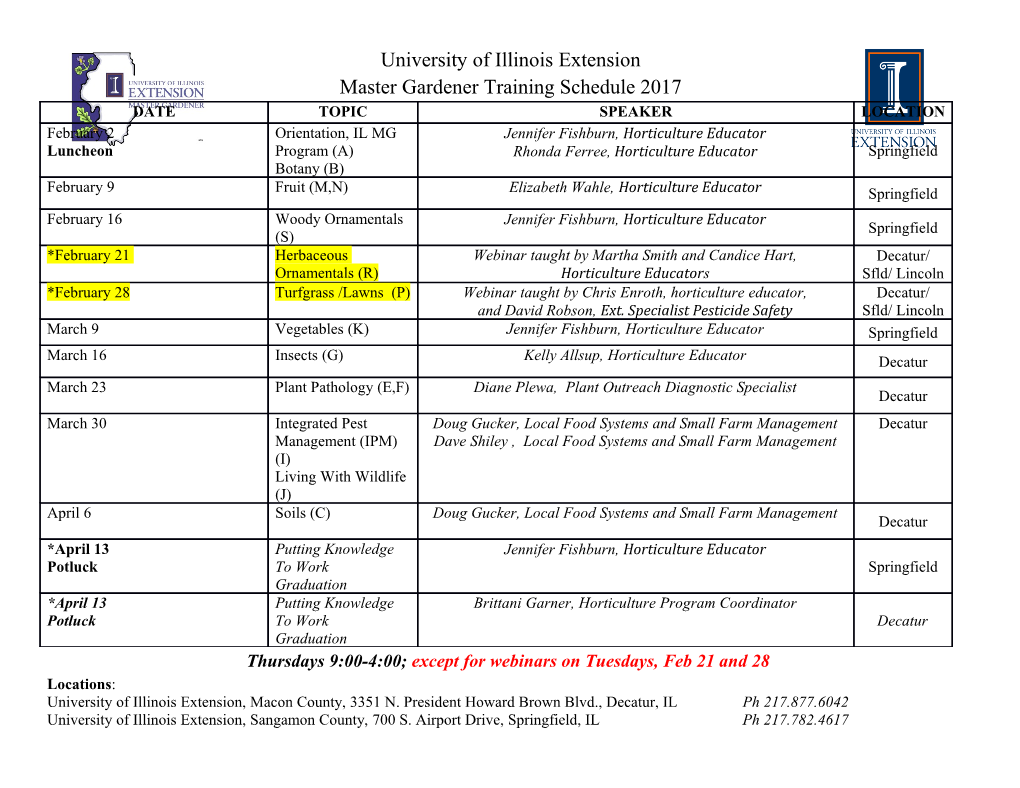
A Tutorial on Convex Optimization II: Duality and Interior Point Methods Haitham Hindi Palo Alto Research Center (PARC), Palo Alto, California 94304 email: [email protected] Abstract— In recent years, convex optimization has become a and concepts. For detailed examples and applications, the computational tool of central importance in engineering, thanks reader is refered to [8], [2], [6], [5], [7], [10], [12], [17], to its ability to solve very large, practical engineering problems [9], [25], [16], [31], and the references therein. reliably and efficiently. The goal of this tutorial is to continue the overview of modern convex optimization from where our We now briefly outline the paper. There are two main ACC2004 Tutorial on Convex Optimization left off, to cover sections after this one. Section II is on duality, where we important topics that were omitted there due to lack of space summarize the key ideas the general theory, illustrating and time, and highlight the intimate connections between them. the four main practical applications of duality with simple The topics of duality and interior point algorithms will be our examples. Section III is on interior point algorithms, where focus, along with simple examples. The material in this tutorial is excerpted from the recent book on convex optimization, by the focus is on barrier methods, which can be implemented Boyd and Vandenberghe, who have made available a large easily using only a few key technical components, and yet amount of free course material and freely available software. are highly effective both in theory and in practice. All of the These can be downloaded and used immediately by the reader theory we cover can be readily extended to general conic both for self-study and to solve real problems. programs, such as second order cone programs (SOCP) and semidefinite programs (SDP); see [8] for details. I. INTRODUCTION Notation Our notation is standard [8]. For example, we will The objectives are to continue the overview of modern use dom to denote the domain of a function, int denotes the convex optimization from where our ACC2004 Tutorial on interior of a set, relint denotes the interior of a set relative Convex Optimization [18] left off. Specifically, we review to the smallest affine set containing it (its affine hull), and the role of duality and demonstrate both its practical and () to denote componentwise inequality when applied to theoretical value in convex optimization; describe interior vectors, and semidefinite ordering, when applied to matrices. n point methods for solving convex optimization problems; and S+ denotes the set of symmetric n × n positive semidefinite highlight the intimate connections between duality and the matrices. solution methods. We aim to give an overview of the essential ideas mainly II. DUALITY defining concepts and listing properties without proof. With In this section, we present the basic ideas of duality the exception of minimal narrative comments by the present theory, illustrating along the way its four main practical author, all of the material in this tutorial is excerpted from uses: bounding nonconvex problems; stopping criteria in chapters 5, 9, 10 and 11 of the book Convex Optimization algorithms; decomposition of large problems; and sensitivity by Boyd and Vandenberghe [8], where complete details analysis. with lucid explanations can be found. This will be our main reference in this tutorial. I am deeply indebted to the A. The Lagrange dual function authors, for generously allowing me to use their material in preparing this tutorial. The authors have also made available 1) The Lagrangian: We consider an optimization problem on the internet a large amount of free course material and in the standard form: software [14], [22]. minimize f0(x) The reader is assumed to have a working knowledge of subject to fi(x) ≤ 0; i = 1; : : : ; m (1) linear algebra and basic vector calculus, and some (minimal) hi(x) = 0; i = 1; : : : ; p; exposure to optimization. However, due to its different focus, this paper can be read quite independently of our Part I with variable x 2 Rn, and where the functions from Rn ! R paper [18]. The following references also cover the topics f0, f1; : : : ; fm, and h1; : : : ; hp are the objective, inequality of optimization [26], [24], [1], [3], [4], convex analysis [23], constraints and equality constraints, respectively. We assume m dom p dom [28], [30], [19], [15], and numerical computation [29], [13], its domain D = i=0 fi \ i=1 hi is nonempty, [11], [20]. and denote the optimal value of (1) by p?. For now, we do T T Also, in order keep the paper quite general, we have not assume the problem (1) is convex. tried to not to bias our presentation toward any particular The basic idea in Lagrangian duality is to take the con- audience. Hence, the examples used in the paper are very straints in (1) into account by augmenting the objective simple and intended merely to clarify the optimization ideas function with a weighted sum of the constraint functions. We n m p define the Lagrangian L : R × R × R ! R associated which has inequality constraint functions fi(x) = −xi, i = with the problem (1) as 1; : : : ; n. To form the Lagrangian we introduce multipliers λi for the n inequality constraints and multipliers νi for the equality m p constraints, and obtain L(x; λ, ν) = f0(x) + λifi(x) + νihi(x); T n T L(x; λ, ν) = c x − λixi + ν (Ax − b) i=1 i=1 i=1 X X = −bT ν + (c + AT ν − λ)T x: m p with dom L = D × R × R . We refer to λi as the La- P The dual function is grange multiplier associated with the ith inequality constraint T T T fi(x) ≤ 0; similarly we refer to νi as the Lagrange multiplier g(λ, ν) = inf L(x; λ, ν) = −b ν + inf(c + A ν − λ) x; x x associated with the ith equality constraint hi(x) = 0. The vectors λ and ν are called the dual variables or Lagrange which is easily determined analytically, since a linear function is bounded below only when it is identically zero. Thus, g(λ, ν) = multiplier vectors associated with the problem (1). −∞ except when c + AT ν − λ = 0, in which case it is −bT ν: 2) The Lagrange dual function: We define the Lagrange m p −bT ν AT ν − λ + c = 0 dual function (or just dual function) g : R × R ! R as g(λ, ν) = m −∞ otherwise. the minimum value of the Lagrangian over x: for λ 2 R , ν 2 Rp, Note that the dual function g is finite only on a proper affine m p m p subset of R × R . We will see that this is a common occurrence. The lower bound property (2) is nontrivial only g(λ, ν) = inf f0(x) + λifi(x) + νihi(x) : T x2D when λ and ν satisfy λ 0 and A ν − λ + c = 0. When i=1 i=1 ! T X X this occurs, −b ν is a lower bound on the optimal value of the When the Lagrangian is unbounded below in x, the dual LP (3). function takes on the value −∞. Since the dual function is the pointwise infimum of a family of affine functions B. The Lagrange dual problem of (λ, ν), it is concave, even when the problem (1) is not For each pair (λ, ν) with λ 0, the Lagrange dual convex. function gives us a lower bound on the optimal value p? of 3) Lower bounds on optimal value: It is easy to show [8] the optimization problem (1). Thus we have a lower bound that the dual function yields lower bounds on the optimal that depends on some parameters λ, ν. A natural question value p? of the problem (1): For any λ 0 and any ν we is: What is the best lower bound that can be obtained from have the Lagrange dual function? g(λ, ν) ≤ p?: (2) This leads to the optimization problem maximize g(λ, ν) This important property is easily verified. Suppose x~ is a (4) feasible point for the problem (1), i.e., fi(x~) ≤ 0 and subject to λ 0: hi(x~) = 0, and λ 0. Then we have This problem is called the Lagrange dual problem associated m p with the problem (1). In this context the original problem (1) λifi(x~) + νihi(x~) ≤ 0; is sometimes called the primal problem. The term dual i=1 i=1 feasible, to describe a pair (λ, ν) with λ 0 and g(λ, ν) > X X since each term in the first sum is nonpositive, and each term −∞, now makes sense. It means, as the name implies, that in the second sum is zero, and therefore (λ, ν) is feasible for the dual problem (4). We refer to (λ?; ν?) as dual optimal or optimal Lagrange multipliers if m p L(x;~ λ, ν) = f (x~) + λ f (x~) + ν h (x~) ≤ f (x~): they are optimal for the problem (4). 0 i i i i 0 The Lagrange dual problem (4) is a convex optimization i=1 i=1 X X problem, since the objective to be maximized is concave and Hence the constraint is convex. This is the case whether or not the primal problem (1) is convex. g(λ, ν) = inf L(x; λ, ν) ≤ L(x;~ λ, ν) ≤ f0(x~): x2D 1) Making dual constraints explicit: The example above Since g(λ, ν) ≤ f0(x~) holds for every feasible point x~, the shows that it can happen (and often does) that the domain inequality (2) follows.
Details
-
File Typepdf
-
Upload Time-
-
Content LanguagesEnglish
-
Upload UserAnonymous/Not logged-in
-
File Pages11 Page
-
File Size-