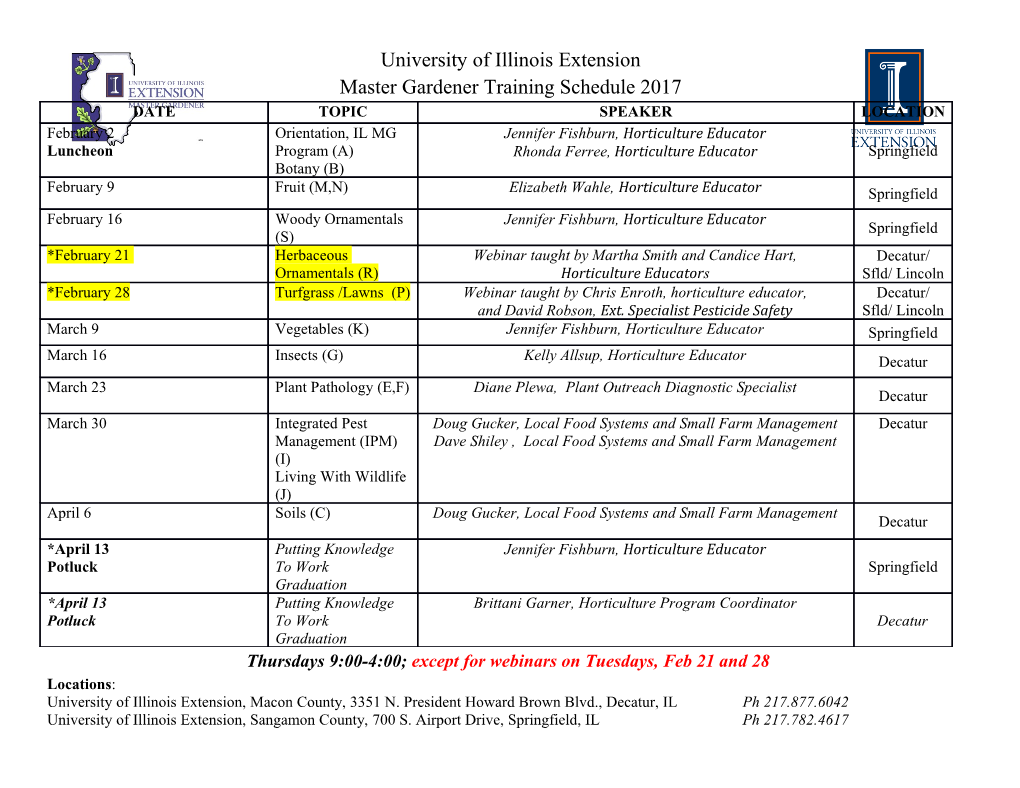
proceedings Proceedings On a General Definition of Conditional Rényi Entropies † Velimir M. Ili´c 1,*, Ivan B. Djordjevi´c 2 and Miomir Stankovi´c 3 1 Mathematical Institute of the Serbian Academy of Sciences and Arts, Kneza Mihaila 36, 11000 Beograd, Serbia 2 Department of Electrical and Computer Engineering, University of Arizona, 1230 E. Speedway Blvd, Tucson, AZ 85721, USA; [email protected] 3 Faculty of Occupational Safety, University of Niš, Carnojevi´ca10a,ˇ 18000 Niš, Serbia; [email protected] * Correspondence: [email protected] † Presented at the 4th International Electronic Conference on Entropy and Its Applications, 21 November–1 December 2017; Available online: http://sciforum.net/conference/ecea-4. Published: 21 November 2017 Abstract: In recent decades, different definitions of conditional Rényi entropy (CRE) have been introduced. Thus, Arimoto proposed a definition that found an application in information theory, Jizba and Arimitsu proposed a definition that found an application in time series analysis and Renner-Wolf, Hayashi and Cachin proposed definitions that are suitable for cryptographic applications. However, there is still no a commonly accepted definition, nor a general treatment of the CRE-s, which can essentially and intuitively be represented as an average uncertainty about a random variable X if a random variable Y is given. In this paper we fill the gap and propose a three-parameter CRE, which contains all of the previous definitions as special cases that can be obtained by a proper choice of the parameters. Moreover, it satisfies all of the properties that are simultaneously satisfied by the previous definitions, so that it can successfully be used in aforementioned applications. Thus, we show that the proposed CRE is positive, continuous, symmetric, permutation invariant, equal to Rényi entropy for independent X and Y, equal to zero for X = Y and monotonic. In addition, as an example for the further usage, we discuss the properties of generalized mutual information, which is defined using proposed CRE. Keywords: Rényi entropy; conditional entropy; mutual information PACS: 89.70.Cf, 87.19.lo 1. Introduction Rényi entropy (RE) is a well known one parameter generalization of Shannon entropy. It has successfully been used in a number of different fields, such as statistical physics, quantum mechanics, communication theory and data processing [1,2]. On the other hand, the generalization of conditional Shannon entropy to Rényi entropy case is not uniquely defined. Thus, different definitions of conditional Rényi entropy has been proposed in the context of channel coding [3], secure communication [4–6] and multifractal analysis [1]. However, no one of the generalization satisfies a set of basic properties which are satisfied by the Shannon conditional entropy and there is no general agreement about the proper definition, so the choice of the definition depends on application purpose. Essentially, in all of the previous discussions, CRE can be represented as an average uncertainty about a random variable X if a random variable Y is given. In this paper, we introduce three-parameter CRE which contains previously defined conditional entropies as special cases that can be obtained by a proper choice of the parameters. Moreover, it satisfies Proceedings 2018, 2, 166; doi:10.3390/ecea-4-05030 www.mdpi.com/journal/proceedings Proceedings 2018, 2, 166 2 of 6 all of the properties that are simultaneously satisfied by the previous definitions, so that it can successfully be used in aforementioned applications. Thus, we show that the proposed CRE is positive, continuous, symmetric, permutation invariant, equal to Rényi entropy for independent X and Y, equal to zero for X = Y and monotonic. One of the most frequent usages of conditional entropies is for the definition of mutual information (MI). The MI represents information transfer between the channel input and output which can be defined as the input uncertainty reduction if the output symbols are known. Thus, the MI which corresponds to the a-b-g entropy measured as a difference between the input RE and the input a-b-g CRE when the output is given. We analyze the properties of a-b-g MI and show that the basic properties of Shannon MI, such as continuity, annulation for independent input and output and reducing to the output entropy for independent events, are also satisfied in the case of a-b-g MI, which further validates the usage of a-b-g CRE. The paper is organized as follows. In Section2 we review basic notions about Rényi entropy. The definition of a-b-g CRE is introduced in Section3 and its properties are considered in Section4. The a-b-g MI is considered in Section5. 2. Rényi Entropy Let X be a discrete random variable taking values from a sample space fx1, ... , xng and distributed according to PX = (p1,..., pn). The Rényi entropy of X of order a (a > 0) is defined as ! 1 a Ra(X) = log2 ∑ PX(x) . (1) 1 − a x For two discrete random variables X and Y, with joint density PXY, the joint Rényi entropy is defined with ! 1 a Ra(X, Y) = log2 ∑ PXY(x, y) . (2) 1 − a x,y The following properties hold [1]: (A1) Ra(X) is continuous with respect to PX; (A2) Adding a zero probability event to the sample space of X does not change Ra(X) (A3) Ra(X) takes its largest value for the uniformly distributed random variable i.e., Ra(X) ≤ log2 n, (3) with equality iff PX = (1/n, . , 1/n). (A4) If PX = (1, 0, . , 0), then Ra(X) = 0; (A5) Ra(X) is symmetric with respect to PX, i.e., if X ∼ (p1, ... , pn) and Y ∼ ((pp(1), ... , pp(n))), where p is any permutation of f1, . , ng, than Ra(X) = Ra(Y) for all PX; (A6) Ra(X) is continuous with respect to a and in the limit case reduces to Shannon entropy S(X) [7]: S(X) = lim Ra(X) = − ∑ PX(x) log2 PX(x); (4) a!1 x (A7) Rényi entropy can be represented as quasi-linear mean of Hartley information content H = − log2 PX as: ! −1 Ra(X) = ga ∑ P(x)ga(H(x)) (5) x where the function g is defined with a 8 2(1−a)x−1 <> , for a 6= 1; ga(x) = 1 − a (6) :> x, for a = 1. Proceedings 2018, 2, 166 3 of 6 or any linear function of Equation (6) (it follows from a well known result from mean value theory: if one function is a linear function of another one, they generate the same quasi-linear mean). 3. a-b-g Conditional Rényi Entropy Previously, several definitions of the CRE has been proposed [8] as a measure of average uncertainty about random variable Y when X is known. In this section, we unify all of this definitions and we define the CRE as three parameter function which can access all of the previous definitions by a special choice of the parameters. Let (X, Y) ∼ PX,Y, X ∼ PX. The Rényi entropy of conditional random variables YjX = x distributed according to PYjX=x is denoted with Ra(YjX = x). The conditional Rényi entropy is defined with ! b,g −1 (b) Ra (YjX) = gg ∑ PX (x)gg(Ra(YjX = x)) (7) x where gg is given with Equation (6) and escort distribution of PX is defined with: P (x)b (b)( ) = X PX x b . (8) ∑x PX(x) The definition can straightforwardly be extended to the joint conditional entropy. For random variables X,Y,Z, we define joint conditional entropy as: ! b,g −1 (b) Ra (Y, ZjX) = gg ∑ PX (x)gg(Ra(Z, YjX = x)) (9) x (b) The definitions extends to the case b = ¥, by taking a limit b ! ¥, and by using limb!¥ PX (x) = maxx PX(x). In the case of a = b = 1, the definitions reduces to the Shannon case. By choosing appropriate values for b and g we get the previously considered definitions as follows: [C-CRE] b = g = 1, Cachin [4] C Ra (YjX) = ∑ PX(x) Ra(YjX = x) (10) x [JA-CRE] b = g = a, Jizba and Arimitsu [1] JA 1 (a) (1−a)Ra(YjX=x) Ra (YjX) = log2 ∑ PX (x)2 (11) 1 − a x [RW-CRE] b = ¥ Renner and Wolf [5] (RW-CRE) ( RW minx Ra(YjX = x) if a > 1 Ra (YjX) = (12) maxx Ra(YjX = x) if a < 1 [A-CRE] b = 1, g = 2 − a−1 Arimoto [3] (A-CRE) 1 1−a A a Ra(YjX=x) Ra (YjX) = log2 ∑ PX(x)2 (13) 1 − a x [H-CRE] a = g, b = 1 Hayashi [6] (H-CRE). H 1 (1−a)Ra(YjX=x) Ra (YjX) = log2 ∑ PX(x)2 (14) 1 − a x Proceedings 2018, 2, 166 4 of 6 4. Properties of a-b-g CRE The a-b-g CRE satisfies the set of important properties for all a, b, g: b,g (B1) Ra (YjX) ≥ 0 b,g (B2) Ra (YjX) is continuous with respect to PX,Y; b,g (B3) Ra (YjX) is symmetric with respect to PYjX=x for all PX 2 Dn, i = 1, . , n and PYjX=x; P P x Rb,g(YjX) = R(YjX = x ) (B4) If YjX=x is a permutation of YjX=x1 , for all , then, and a 1 b,g (B5) If X and Y are independent, then Ra (YjX) = R(Y) b,g (B6) If X = Y, then Ra (YjX) = 0 (B7) In the case of a = b = 1, the definitions reduces to the Shannon case. (B8) Let X, Y, Z be random variables distributed according with joint distribution PX,Y,Z and corresponding marginal distributions PX, PY, PY,Z b,g b,g Ra (Y, ZjX) ≤ Ra (YjX) (15) The proofs for the properties B1–B6 straightforwardly follows from the definition Equation (7) of the conditional Rényi entropy and from the properties A1–A5 of Rényi entropy.
Details
-
File Typepdf
-
Upload Time-
-
Content LanguagesEnglish
-
Upload UserAnonymous/Not logged-in
-
File Pages6 Page
-
File Size-