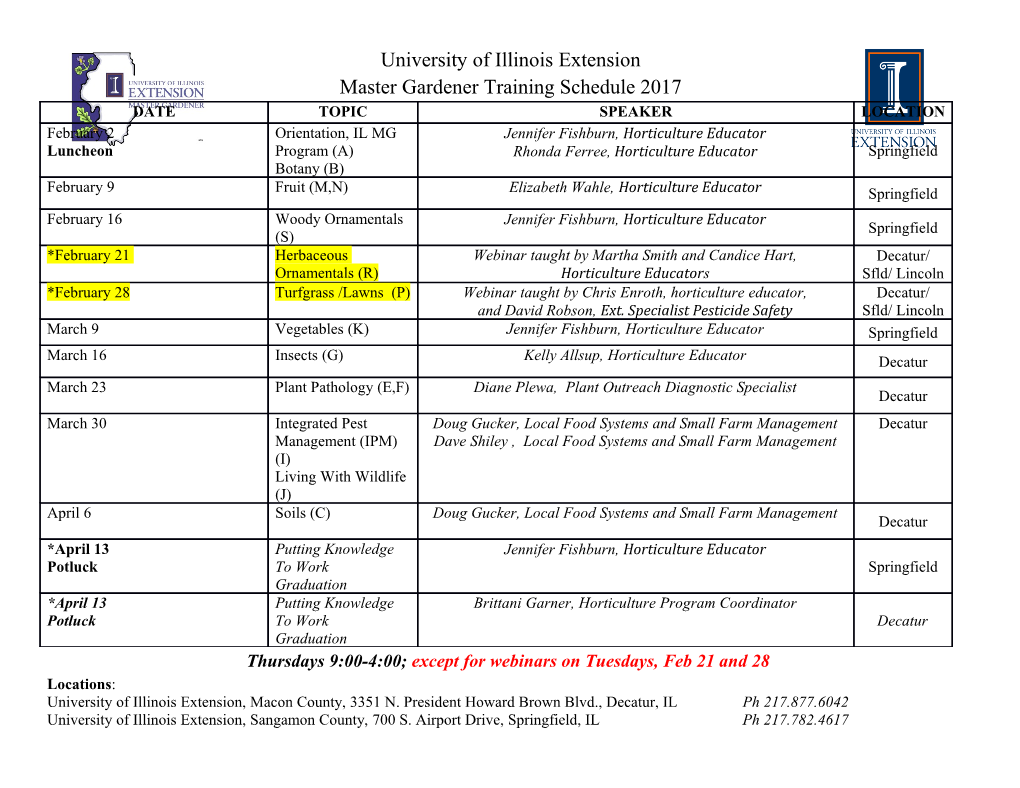
Bioinformatic analysis of the interface between mitochondrial biogenesis and apoptotic cell death signaling pathways in Parkinson's disease. Robert Bentham Supervised by Dr G. Szabadkai and Dr K. Bryson March 3, 2012 Contents 1 Introduction 1 2 Microarray Analysis 2 2.1 Data acquisition . 3 2.2 Quality Control . 3 2.2.1 Normalisation . 5 2.3 LIMMA . 5 2.3.1 Results . 7 2.4 Gene Set Analysis . 7 2.4.1 GSEA . 8 2.4.2 GAGE . 8 2.4.3 Results . 9 3 Conclusion 9 References 14 A Tables 16 B R code 17 1 Introduction Mitochondria are subcellular organelles present in most eukaryotic cells. They have a complex evolutionary history, endosymbiotic theory saying that they evolved from free living bacteria which became incorporated within a cell. They have their own DNA (known as mtDNA) which is inherited from the mother only. Mitochondria primarily function being to provide ATP to the rest of the cell which is used as a source of energy means that they are essential for the healthy function of a cell Cell survival is dependent on the maintenance of a healthy cellular mitochondrial pool which is in turn dependent on two processes. The degradation of damaged mitochondria by autophagy and the process of mitochondrial renewal, mitochondrial biogenesis. This project will chiefly concern the latter of these processes, mitochondrial biogenesis. This biogenesis is simply the process of which new mitochondria are formed, however, the precise biological machinery controlling this process however is highly complex. Despite this complexity the PGC-1 family of transcriptional coactivators have been identifies as the master regulators of mitochondrial biogenesis[14]. 1 Robert Bentham Cancer, cardiovascular disease and neurodegenerative diseases such as Parkinson's have all been associated with dysfunction of the mitochondria [14] [5]. In a recent review on the overlapping pathways involved in Parkinson's and cancer,[3] the role of mitochondria in both is stressed. It has previously been shown that PGC-1α down regulation occurs in Parkinson's disease [19], this could lead to the pathogenesis of Parkinson's disease due to mitochondrial dysfunction, possibly meaning that PGC-1α is a potential therapeutic target. Additionally, in previous bioinformatic analysis of the role of PGC-1 in cancer[1], PGC-1 also was found to down regulate stress pathways involved in DNA damage. Interestingly DNA damage has also been suggested to be associated with Parkinson's disease [12]. The aim of this work is to test the hypothesis that in clinical samples of Parkinson's disease besides downregulation of mitochondrial pathways, there are alterations in pathways involving DNA damage. To do this previously published microarray data will be studied, and significantly expressed genes and gene pathways identified. 2 Microarray Analysis A microarray is a device for measuring the expression levels of large numbers of genes. It does this via utilising the process of DNA hybridisation, which is illustrated in Figure 1. The expression level of each gene is detected by hybridisning with a number of oligonucleotide fragments on the chip acting as probes. For a single gene there are 11 perfect match (PM) probes and 11 mismatched probes (MM), in which the sequence differs by a single base. These MM probes are important for quality control, they measure the specificity of the hybridisation by giving an indication of any cross-hybridisation that has occurred. Thus the chip is covered with large number of probes of DNA both of type PM and MM. The target RNA from the experimental sample is manipulated and fluorescently labelled. So when hybridisation occurs with the probes, there is a measure of gene expression obtained from the intensity of the fluorescence at each spot on the microarray. For Affymetrix chips two microarrays from different experimental conditions, one being from a control sample can then be compared, and differences arising from the experimental condition inferred. Figure 1: Image from Affymetrix illustrating the construction and workings of an affymetrix microarray. There are numerous issues in the use of microarrays or any other high-throughput technique, firstly there is a huge amount of data that must be analysed in a statistically robust manner. To maintain this robustness quality control is a essential part of any analysis, these issues and others are discussed in [20], 2 Robert Bentham unfortunately with microarrays different statistical techniques can lead to quite different results, so one must proceed with care. There are also many things beyond our control, there is technical variability in the actual experiment. This comes from differences in the temperature and pH values which affecting hybridisation on the microarray. Additionally each probe can not be optimised for hybridation equally, adding the stochastic nature of biological systems this leads to very noisy results with large systematic bias. Any statistical analysis must deal with these levels of noise and judge when to reject a microarray from the analysis if any systematic bias can no longer be tolerated. 2.1 Data acquisition For the aims of this report four datasets were identified for analysis involving microarrays from patients with Parkinson's disease. The first dataset, which will be referred to as the Zheng dataset (available from GEO series accession number GSE24378 [9]) and is part of the meta study that identified PGC1-α as a potential target for parkinson's disease [19]. This particular study is made of 17 samples with 8 replicates for parkinson's disease and 9 replicates for the controls, the RNA used on the microarray is from 500 dopamine (DA) neurons from the pars compacta (SNc) of the substantia nigra. Another three data sets were furthermore selected for analysis, these included another dataset, which will be called the Middleton dataset (available through GEO Series accession number GSE20292[8]) which was also used in the meta study [19] [28]. Middleton has 18 control replicates and 11 replicates with parkinson's disease. The next data set chosen, named Mullen (available through GEO Series accession number GSE7621[6]) has 16 replicates for Parkinson's disease and 9 replicase for the controls [17]. The final data set, will be referred to as Moran (available through GEO Series accession number GSE8397[7] [21]). The Moran dataset, had microarrays from the Affymetrix U133A and U133B chip, of these only the U133A chip were used, as well as this microarrays taken from the substantia nigra with no distinction between the lateral and medial parts. After this the Moran dataset contained 24 replicates for Parkinson's disease and 15 replicates for the controls. All of the data sets chosen were from microarrays using Affymetrix chips , Middleton and Moran used U133A chips while Mullen uses the more recent U133 plus 2.0, these two differ in the number of genes they detect the plus 2.0 having probes for an additional 6500 genes. In contrast to this the Zheng study uses the U133 X3P chip which uses probes designed to examine sequences closer to the 3' end of transcripts, which is useful in cases of bad RNA degradation which happens from the 5' end of transcripts. 2.2 Quality Control The purpose of quality control is to identify arrays which are not possible to correct and use in the analysis. Problems may include mistakes in the experimental procedure or a very high signal to noise ratio. For a comprehensive look at array quality a variety of measures should be examined, this can be quite time consuming, however it is possible to automate this process somewhat with R package arrayQualityMetrics [15]. A few of the main methods of quality control used will be discussed here, though there are many different techniques many of which are generated automatically in the arrayQualityMetrics package. The first thing to check for is array defects by looking at a spatial plot of intensitied, areas such as high intensity could indicate uneven hybridisation, while patterns in the spatial plot could indicate a particle being loose in the chip and scratching the surface while hybridisation occurs in a centrifuge. Figure ??a shows the spatial plots for all chips in the Middleton dataset, in this case and all other datasets examined there were no problems with either array defects or hybridisation effects. The next quantity to check for is RNA degradation or poor labeling. It is well known that RNA degra- dation starts from the 5' end of a molecule and finishes at the 3' end, a feature that the chip U133 plus 2.0 makes use of. For this reason if RNA degradation has occurred the mean intensity of the probes at the 3' end should be much higher, this can easily be checked and plotted in R. Figure 2b shows an increase in the intensities of probes at the 3' end in the Zheng dataset. Indeed all the other datasets showed similar results, this result could also be due to inefficient labeling as the labeling reaction used in preparing the 3 Robert Bentham RNA degradation plot C1 C2 C3 80 C4 PD1 PD2 PD3 C5 60 PD4 PD5 PD6 PD7 C6 C7 40 PD8 C8 C9 Mean Intensity : shifted and scaled 20 0 0 2 4 6 8 10 5' <−−−−−> 3' Probe Number (a) Spatial plot showing probe intensities of microarrays (b) RNA degradation plot showing severe degradation for the Middleton dataset, all microarrays here are nor- for samples in the Zheng dataset despite the special mal. This plot was generated with the arrayQualityMet- U133 X3P chip here designed for cases with bad RNA rics package degradation. (c) PM and MM log2 intensity graph for data in the Middleton study generated with the arrayQualityMetrics package. Figure 2: Quality Control measures used in analysis RNA to sample occurs from the 3' end, however due to all samples being taken from postmortems it is very likely that the cause of this result is RNA degradation.
Details
-
File Typepdf
-
Upload Time-
-
Content LanguagesEnglish
-
Upload UserAnonymous/Not logged-in
-
File Pages20 Page
-
File Size-