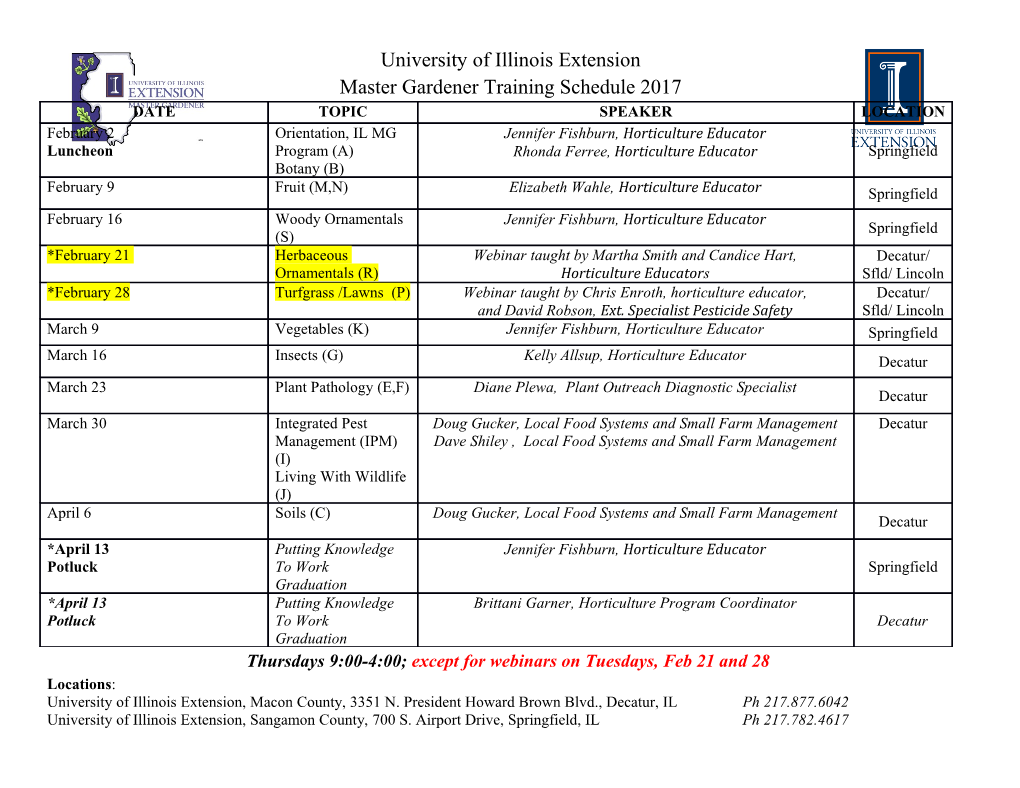
AAAI Technical Report SS-12-02 Designing Intelligent Robots: Reintegrating AI Scaling-Up Knowledge for a Cognizant Robot Thomas Degris∗, Joseph Modayily ∗Flowers, INRIA, 351 cours de la libration, 33405 Talence Cedex, France yRLAI, University of Alberta, Edmonton, AB, Canada, T6G 2E8 Abstract cations and navigate between them. Fourth, some animals use a large number of diverse sensorimotor signals from the This paper takes a new approach to the old adage that knowl- world. Mammals, for example, are able to take advantage edge is the key for artificial intelligence. A cognizant robot is a robot with a deep and immediately accessible understand- of smell, sight, hearing, taste and touch, along with temper- ing of its interaction with the environment—an understand- ature, acceleration, hair movements and also their internal ing the robot can use to flexibly adapt to novel situations. senses such as pain, hunger, fatigue, guts, and propriocep- Such a robot will need a vast amount of situated, revisable, tion. In addition, they interact with their environment using and expressive knowledge to display flexible intelligent be- a large number and variety of muscles and actuators. haviors. Instead of relying on human-provided knowledge, To demonstrate comparable awareness of their environ- we propose that an arbitrary robot can autonomously acquire ment, a cognizant robot would need to have lots of immedi- pertinent knowledge directly from everyday interaction with ate knowledge. For instance, a reaction as basic as flinching the environment. We show how existing ideas in reinforce- in response to a moving object involves knowledge in the ment learning can enable a robot to maintain and improve its knowledge. The robot performs a continual learning process form of a ready to use flinching skill, and also, perhaps, a that scales-up knowledge acquisition to cover a large number prediction of the trajectory of the object. Competent naviga- of facts, skills and predictions. This knowledge has seman- tion involves a wide-range of knowledge about the topology tics that are grounded in sensorimotor experience. We see the and characteristics of the environment, as well as skills rang- approach of developing more cognizant robots as a necessary ing from obstacle avoidance to planning a new route when a key step towards broadly competent robots. path is unexpectedly blocked. Detecting novelty and exhibit- ing curiosity-driven behaviour also involves knowledge: us- Knowledge: a Bottleneck for Cognizant Robots ing existing knowledge to contextualize newly observed sen- sorimotor data, and a repertoire of behaviors to assess the Any robot with a closed-loop control has some awareness nature of the novelty. The more knowledge to which a robot of its environment. In 1948, Walter’s robot tortoises Elmer has immediate access, the more the robot can be cognizant. and Elsie could respond to their environment by avoiding The bottleneck in developing such robots is the lack of obstacles or moving towards a light (Walter, 1950). More methods that are able to acquire and maintain a large quan- recently, robot vacuum cleaners detect dirty areas and spend tity of immediately usable knowledge. A traditional ap- more time cleaning them. Some are able to build maps of proach is to manually provide the robot with knowledge their local space. Autonomous cars are able to take appro- about its environment in the form of models, skills, and fea- priate action to react to changing traffic conditions (Urmson tures. But such methods are problematic for at least two et al., 2008). reasons. One, scaling-up knowledge is difficult if it re- In comparison, most animals can be considered more cog- quires manual interventions for every modification. Two, nizant than modern robots in many ways. First, even simple such knowledge will be necessarily incomplete or inaccu- animals such as fruit flies, cockroaches and spiders are able rate in an open environment. Moreover, knowledge is often to adapt their behavior to their environment through learning encoded in a form that cannot be autonomously updated by from everyday experience (Greenspan and van Swinderen, the robot (e.g. hand-coded or learned-but-fixed features and 2004). Second, animals can learn to anticipate what is go- skills). ing to happen next, as it has been demonstrated with dogs, As a solution to this bottleneck, we consider Horde: a pigeons, and insects (Pearce, 1997). Third, animals demon- conceptually simple architecture that was introduced re- strate a deep understanding of their sensorimotor experience cently (Sutton et al., 2011). Horde proposes a theoretically by exhibiting complex behaviors. Cats can detect what is un- sound formalism for representing knowledge. Horde allows usual in their environment and exhibit curiosity-driven be- a robot to learn autonomously from its everyday interaction havior. Crows, bees, and rats can successfully remember lo- with its environment. Horde is able to maintain and acquire Copyright c 2012, Association for the Advancement of Artificial a large number of situated, revisable, and expressive pieces Intelligence (www.aaai.org). All rights reserved. of knowledge that are exploitable by a cognizant robot. To Consequently, the goal of developing a cognizant robot Environment requires the definition of only these two elements of knowl- edge: an internal state update function and a behavior pol- icy. A robot will demonstrate a deep understanding of its environment by extracting information from observations to Observation Action adequately update its internal state, so that the behavior pol- Robot icy will efficiently output adapted actions. Other elements of knowledge support the construction of these two functions. Another important element of knowledge are skills. In- dependently of the robot’s current behavior, we would like to represent the knowledge of being able to achieve some Figure 1: The robot is a situated agent that can only learn goals. We can formalize skills as policies π(ajx). Similarly from its sensorimotor interaction stream of observations and to the option framework, we can also define a termination actions. function C : X ! [0; 1] that, given the state of the agent, defines the probability of terminating a policy, maybe when reap these benefits, the robot should have an abundance of a goal is achieved (Sutton et al., 1999). For a mobile robot, experience and the ability to autonomously explore its envi- a useful skill such as “going back to the docking station” ronment. would be represented by a policy that the robot could fol- low to go back to its docking station and the skill would Defining Knowledge for a Cognizant Robot terminate either when the robot observes it has successfully connected or after a time out. To make our discussion about knowledge more concrete, Given a policy and a termination function, another ele- we start by defining the interaction between the robot and ment of knowledge are the predictions about observable sig- its environment. We assume that this interaction is divided nals. An observable signal z is any function of the observa- into discrete time steps. Despite the fact that this assump- tions or the state of the agent. If the current time is t, we are tion does not characterize robots using analog electronics interested in predicting the value z at the time T (where for their behavior (e.g. Walter’s robot tortoises), it is a re- T T > t) given the current state x of the agent. These pre- quirement for working with digital computers. Ideally, the t dictions can be conditional on a skill, such as a prediction of length of these time steps should be as small as possible so the value z if a policy π is started from the current time t that the robot has a short reaction time. Note that time steps T and terminated at time T . An example of such prediction for do not need to be of constant length. a mobile robot would be knowledge such as: if I go back to The environment for robots is the physical world, a large the docking station starting from now and until termination, space with essentially unlimited forms of variation. At every will I observe being connected to it? time t, the robot receives a new observation o 2 O from its t Another form of predictions frequently used for knowl- environment (where O is a real vector space). The observa- edge, most notably in reinforcement learning, is the empiri- tion o represents the latest information available on all the t cal return g , that is the prediction of the sum of an observ- sensors of the robot. Not all elements of o may have been t t able signal z all along the way while executing a skill: updated because sensors can have different update times. In response, the robot sends back an action a 2 A where A is T t X also a real vector space. An action includes all the parame- gt = zk: (1) ters for the different actuators available to the robot, includ- k=t ing voltage on motors, parameters for pattern generators or colors on LEDs. Observations and actions are the only in- Such knowledge can represent predictions such as: what is formation exchanged between a robot and its environment, the number of time steps required to go back to the docking as illustrated in Figure 1; they do not constitute knowledge. station? What is the total amount of energy required to reach The first element of knowledge that we formalize is an the docking station? update function U : X × A × O ! X defined as A last element of knowledge commonly used is features. Part of the state of the agent can be a feature vector φt that xt U(xt−1; at−1; ot); is updated every time step. φt can include elements from where X is the space of state of the agent.
Details
-
File Typepdf
-
Upload Time-
-
Content LanguagesEnglish
-
Upload UserAnonymous/Not logged-in
-
File Pages6 Page
-
File Size-