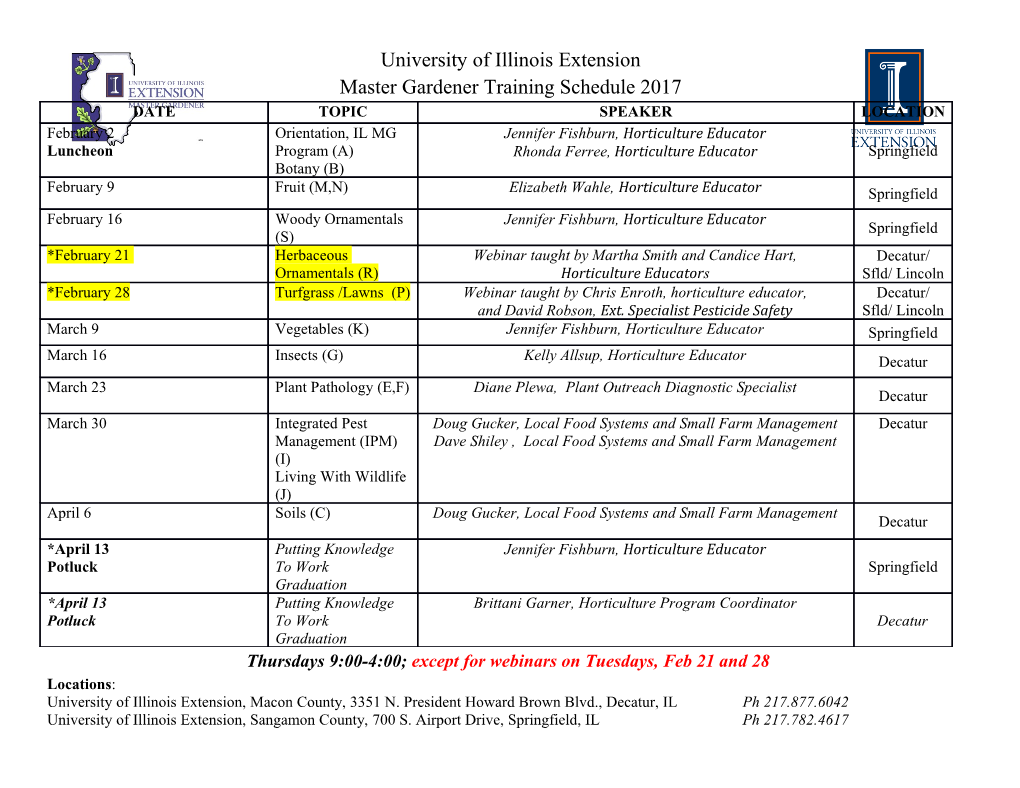
Stat 5120 – Categorical Data Analysis Stat 5120 – Categorical Data Analysis Dr. Corcoran, Fall 2004 Dr. Corcoran, Fall 2004 IV. Comparing Two Proportions Example IV.A Readings for Section IV: Agresti, Chapter 2 Introduction, In an experiment involving 427 genetically engineered mice, 77 Sections 2.2 and 2.3. mice were exposed to the synthetic form of the investigative fiber, and 350 were exposed to the natural form of the fiber. After some We now consider the problem of deciding whether two binomial time, the mice were examined for the presence of tumors. Do the results contained in the table below indicate that exposed mice proportions π1 and π2 are the same, when both π1 and π2 are unknown. have a higher probability of tumor? Tumor Fiber Type Yes No Total Synthetic 40 37 77 Natural 138 212 350 Total 178 249 427 Stat 5120 – Categorical Data Analysis Stat 5120 – Categorical Data Analysis Dr. Corcoran, Fall 2004 Dr. Corcoran, Fall 2004 Example IV.A (cont’d) Measuring the Difference Between Proportions The number of tumors in each of the fiber categories can be Given two population proportions π1 and π2, how do we quantify thought of as a binomial random variable: the number of tumors in the disparity between them? There are three common metrics for accomplishing this: the synthetic group is Binomial(77, πS) and the number of tumors in the natural fiber group is Binomial(350, πN), where πS is the probability that a mouse exposed to synthetic fiber develops a 1. The risk difference, given by π1 – π2. tumor, and πN is the tumor probability for a mouse exposed to natural fiber. 2. The relative risk, given by π1/π2. 3. The odds ratio, given by Odds(π )/Odds(π ), where Formally speaking, we are interested in a test of H0: πS = πN versus 1 2 Odds(π) = π/(1 – π). HA: πS <> πN. Of these three measures, the odds ratio is by far the most widely used in practice. 1 Stat 5120 – Categorical Data Analysis Stat 5120 – Categorical Data Analysis Dr. Corcoran, Fall 2004 Dr. Corcoran, Fall 2004 Inference for the Risk Difference Inference for the Risk Difference Given two population proportions π1 and π2, the MLE of the risk Note that difference is given by RD = p – p , where p and p are the 1 2 1 2 H : π = π H : Risk Difference = 0 observed proportions in each of the two groups. The variance of 0 1 2 ⇔ 0 . the RD is given by Var(p1) + Var(p2), where Var(pi) = πi(1 – πi)/ni, H A : π1 ≠ π 2 H A : Risk Difference ≠ 0 for i = 1, 2, and ni is the sample size in group i. Since π1 and π2 are unknown, we estimate the variance of the RD by substituting p1 Hence, under the null, the statistic Z = (RD – 0)/s.e.(RD) ~ N(0,1). and p2. Moreover, a (1 – α)100% confidence interval for the true risk Provided that n1 and n2 are sufficiently large, by the CLT the difference is given by approximate distribution of RD is N(π1 – π2, s.e.[RD]), where RD ± z1−α / 2s.e.(RD). p (1− p ) p (1− p ) s.e.(RD) = 1 1 + 2 2 . n1 n2 Stat 5120 – Categorical Data Analysis Stat 5120 – Categorical Data Analysis Dr. Corcoran, Fall 2004 Dr. Corcoran, Fall 2004 Example IV.B Inference for the Relative Risk For the data in Example IV.A, the observed proportions of mice The MLE of the relative risk is given by RR = p1/p2, where p1 and p are the observed proportions in each of the two groups. with tumors are pS = 0.5195 for the synthetic treatment group and 2 pN = 0.3943 for the natural treatment group. For the purpose of inference, we normally focus on the log(RR) What is the RD? What is s.e.(RD)? (by convention, “log” in this class refers to the natural log, or log with base e). This is because the distribution of the log(RR) is Give the value of a statistic to test the null hypothesis in IV.A. approximately normal, provided that the sample is sufficiently What is the P-value of this test? large. The MLE of the log relative risk is given by log(RR) = Give a 95% CI for π1 – π2. log(p1/p2) = log(p1) – log(p2). Negative values of log(RR) indicate Is there evidence that exposure to the synthetic fiber leads to that p1 < p2, positive values indicate the reverse. When greater tumor risk? log(RR) = 0, this indicates that p1 = p2. 2 Stat 5120 – Categorical Data Analysis Stat 5120 – Categorical Data Analysis Dr. Corcoran, Fall 2004 Dr. Corcoran, Fall 2004 Inference for the Relative Risk Example IV.C The large-sample standard error of the log(RR) is given by For the data in Example IV.A, what is the RR? What is the n − x n − x s.e.(log[RR]) = 1 1 + 2 2 , log(RR)? What is s.e.(log[RR])? x1n1 x2n2 th where ni and xi are respectively the sample size and number of responses in the i group, for i = 1, 2. Using the RR, give the value of a statistic to test the null hypothesis in IV.A. What is the P-value of this test? Note that H : π = π H : log(π /π ) = 0 0 1 2 ⇔ 0 1 2 . Give a 95% CI for π1/π2. H A : π1 ≠ π 2 H A : log(π1 /π 2 ) ≠ 0 Is this evidence that exposure to the synthetic fiber leads to greater Hence, under the null, the statistic Z = (log[RR] – 0)/s.e.(log[RR]) ~ N(0,1). tumor risk? Moreover, a (1 – α)100% confidence interval for the true risk ratio is given by exp{log(RR) ± z1−α / 2s.e.[log(RR)]}. Stat 5120 – Categorical Data Analysis Stat 5120 – Categorical Data Analysis Dr. Corcoran, Fall 2004 Dr. Corcoran, Fall 2004 Inference for the Odds Ratio Inference for the Odds Ratio The MLE of the odds ratio is given by OR = Odds(p1)/Odds(p2) = The large-sample standard error of the log(OR) is given by {p /(1 – p )}/{p /(1 – p )} = p (1 – p )/p (1 – p ) = 1 1 1 1 1 1 2 2 1 2 2 1 s.e.(log[OR]) = + + + . x1(n2 – x2)/ x2(n1 – x1), where p1 and p2 are the observed proportions x1 n1 − x1 x2 n2 − x2 in each of the two groups, and ni and xi are respectively the sample Note that th size and number of responses in the i group, for i = 1, 2. H : π = π H : log[Odds(π ) / Odds(π )] = 0 0 1 2 ⇔ 0 1 2 . H : π ≠ π H : log[Odds(π ) / Odds(π )] ≠ 0 As with the relative risk, we carry out inference for the odds ratio A 1 2 A 1 2 via the log(OR), due to the more desirable distributional properties Hence, under the null, the statistic Z = (log[OR] – 0)/s.e.(log[OR]) ~ N(0,1). of the log(OR). Moreover, a (1 – α)100% confidence interval for the true odds ratio is given by The MLE of the log odds ratio is given by log(OR) = exp{log(OR) ± z1−α / 2s.e.[log(OR)]}. log[x1(n2 – x2)/ x2(n1 – x1)]. Negative values of log(OR) indicate that p1 < p2, positive values indicate the reverse. When log(OR) = 0, this indicates that p1 = p2. 3 Stat 5120 – Categorical Data Analysis Stat 5120 – Categorical Data Analysis Dr. Corcoran, Fall 2004 Dr. Corcoran, Fall 2004 Example IV.D Practical Advantages of the Odds Ratio Of the three measures discussed, the odds ratio is the most widely used, mainly For the data in Example IV.A, what is the OR? What is the for the following reasons: log(OR)? What is s.e.(log[OR])? 1. The odds ratio is invariant with respect to whether a study is prospective Using the OR, give the value of a statistic to test the null or retrospective. hypothesis in IV.A. What is the P-value of this test? A prospective study is one in which sample subjects are grouped in some way, and then followed forward in time to determine whether the subjects experience Give a 95% CI for Odds(π1)/Odds(π2). the outcome of interest. Is this evidence that exposure to the synthetic fiber leads to greater A retrospective study is one in which subjects are sampled on the basis of the tumor risk? outcome, and then grouped according to their history. This is an efficient design when the outcome is relatively rare (i.e., you would not observe a sufficient number of outcomes if you followed the subjects prospectively). How do the results based on the three measures compare? Stat 5120 – Categorical Data Analysis Stat 5120 – Categorical Data Analysis Dr. Corcoran, Fall 2004 Dr. Corcoran, Fall 2004 Invariance of the Odds Ratio Practical Advantages of the Odds Ratio, continued Whether the design is prospective or retrospective, we want to know how the probability of experiencing the outcome differs by group. Suppose for instance, that S represents the 2. We interpret the parameters of the logistic regression model in terms of event that an individual smokes, and D represents that the individual has a disease. Note the odds ratio. that under the prospective design, we can determine P(D|S). Under the retrospective design, however, we have sampled according to disease status, so we can compute 3. When the outcome is relatively rare, the odds ratio and relative risk are P(S|D), but not P(D|S).
Details
-
File Typepdf
-
Upload Time-
-
Content LanguagesEnglish
-
Upload UserAnonymous/Not logged-in
-
File Pages6 Page
-
File Size-