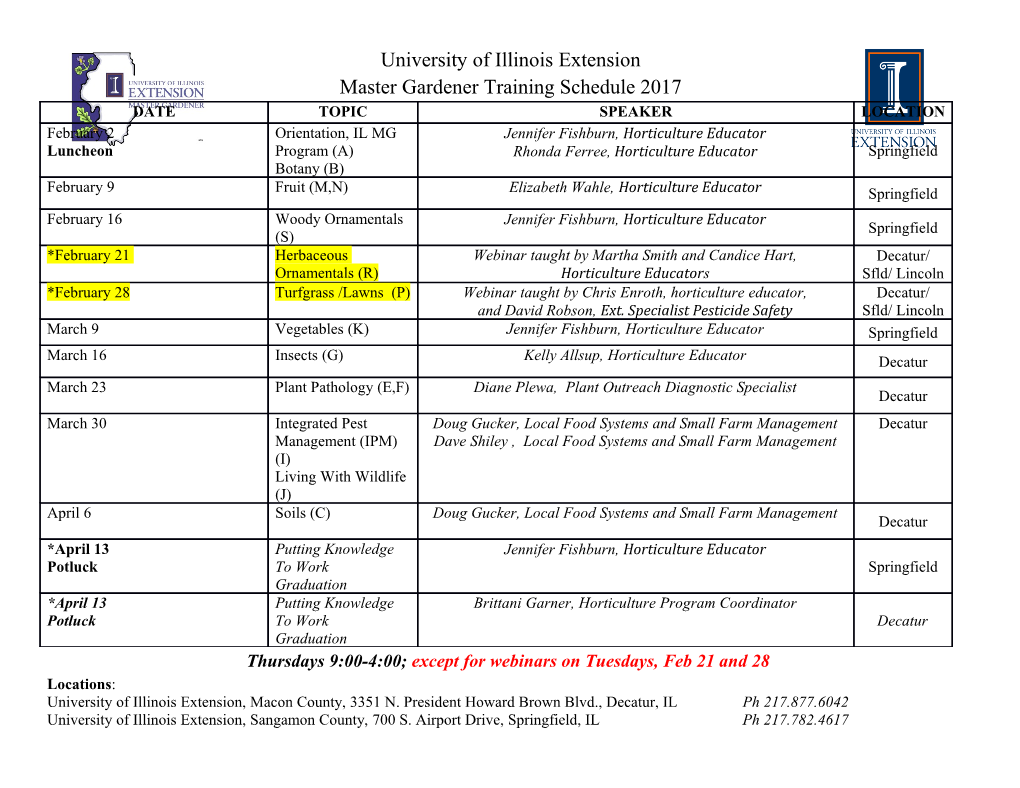
ME-C3100 Computer Graphics, Fall 2019 Jaakko Lehtinen Many slides from Frédo Durand Sampling II, Anti-aliasing, Texture Filtering CS-C3100 Fall 2019 – Lehtinen 1 Admin and Other Things • Give class feedback – please! – You will get an automated email with link – I take feedback seriously – One additional assignment point for submitting • 2nd midterm / exam on Wed Dec 18 – Two sheets of two-sided A4 papers allowed – Those who took 1st midterm: only complete 1st half – Those who didn’t: answer all questions • Last lecture today Aalto CS-C3100 Fall 2019 – Lehtinen 2 Sampling • The process of mapping a function defined on a continuous domain to a discrete one is called sampling • The process of mapping a continuous variable to a discrete one is called quantization • To represent or render an image using a computer, we must both sample and quantize – Today we focus on the effects of sampling continuous discrete value value continuous position discrete position CS-C3100 Fall 2019 – Lehtinen 3 Sampling & Reconstruction The visual array of light is a continuous function 1/ We sample it... – with a digital camera, or with our ray tracer – This gives us a finite set of numbers (the samples) – We are now in a discrete world 2/ We need to get this back to the physical world: we reconstruct a continuous function from the samples – For example, the point spread of a pixel on a CRT or LCD – Reconstruction also happens inside the rendering process (e.g. texture mapping) • Both steps can create problems – Insufficient sampling or pre-aliasing (our focus today) – Poor reconstruction or post-aliasing CS-C3100 Fall 2019 – Lehtinen 4 Sampling Example 1.4 1.2 1 0.8 0.6 0.4 0.2 0 0 1 2 3 4 5 6 7 8 9 10 Continuous function CS-C3100 Fall 2019 – Lehtinen 5 Sampling Example 1.4 1.2 1 0.8 0.6 0.4 0.2 0 0 1 2 3 4 5 6 7 8 9 10 Sampled discrete function CS-C3100 Fall 2019 – Lehtinen 6 Reconstruction Example 1.4 1.2 1 0.8 0.6 0.4 0.2 0 0 1 2 3 4 5 6 7 8 9 10 Nearest neighbor reconstruction CS-C3100 Fall 2019 – Lehtinen 7 Reconstruction Example 1.4 1.2 1 0.8 0.6 0.4 0.2 0 0 1 2 3 4 5 6 7 8 9 10 Piecewise linear reconstruction CS-C3100 Fall 2019 – Lehtinen 8 Reconstruction Example 1.4 As you can see, there are many 1.2 ways of 1 reconstructing a function from the 0.8 samples. 0.6 Some are more 0.4 accurate than others. 0.2 0 0 1 2 3 4 5 6 7 8 9 10 Piecewise cubic reconstruction CS-C3100 Fall 2019 – Lehtinen 9 Wiggliness vs. Sampling Density 1.4 1.2 1 0.8 0.6 0.4 0.2 0 0 1 2 3 4 5 6 7 8 9 10 CS-C3100 Fall 2019 – Lehtinen 10 Wiggliness vs. Sampling Density 2x sampling density 1.4 1.2 1 0.8 0.6 0.4 0.2 0 0 1 2 3 4 5 6 7 8 9 10 CS-C3100 Fall 2019 – Lehtinen 11 Sampling Density • If we sample too coarsely, the samples can be mistaken for something simpler during reconstruction • This is why it’s called aliasing – The new (erroneous) low-frequency sine wave is an alias/ghost of the high-frequency one Input Reconstructed CS-C3100 Fall 2019 – Lehtinen 12 Pre- vs. Post-Aliasing • If the sampling density is too low, no reconstruction method can recover the original function – This is pre-aliasing, information was lost in sampling • Poor reconstruction is post-aliasing 1.4 1.4 1.2 1.2 1 1 0.8 0.8 0.6 0.6 0.4 0.4 0.2 0.2 0 0 0 1 2 3 4 5 6 7 8 9 10 0 1 2 3 4 5 6 7 8 9 10 CS-C3100 Fall 2019 – Lehtinen 13 Recap: Texture Aliasing CS-C3100 Fall 2019 – Lehtinen 14 Aliasing – What Does it Look Like? Nasty mess “Nearest neighbor” Mess gets blurred out “Tri-linear Mip-mapping” CS-C3100 Fall 2019 – Lehtinen 15 Questions? CS-C3100 Fall 2019 – Lehtinen 16 Sampling Density Revisited • If we sample too coarsely, the samples can be mistaken for something simpler during reconstruction • When does this happen? – “The signal must not contain frequencies higher than half the sampling density” (Nyquist, Shannon, and others) Input Reconstructed CS-C3100 Fall 2019 – Lehtinen 17 Sampling Density Revisited • So, in order to prevent aliasing, we should either.. – Filter the high frequencies out from the signal before sampling (this is called “low-pass filtering”) – Sample at a higher rate Input Reconstructed CS-C3100 Fall 2019 – Lehtinen 18 Solution? • Removing high freq’s = low-pass filtering = blur • In practice, we blur before sampling in order to stop high frequencies from messing our image up – In audio, use an analog low-pass filter before sampling – For ray tracing/rasterization: compute image at higher resolution, blur, then resample at lower resolution • Not exactly a proper low-pass prefilter, because aliasing still happens at the higher resolution, but it’s all we can do – For textures, we blur the texture before doing the lookup (not necessary to compute in higher resolution first) • To understand what really happens, we need semi-serious math (Fourier transforms) CS-C3100 Fall 2019 – Lehtinen 19 Digital Cameras • Most digital cameras have an optical low-pass filter in front of the sensor – Yes, it’s essentially a piece of frosted glass (maitolasi) • Some cameras don’t – See comparison between Nikon D800 and D800e CS-C3100 Fall 2019 – Lehtinen 20 Blurring Removes High Frequencies Original image High frequencies removed by blur CS-C3100 Fall 2019 – Lehtinen 21 Types of Blur • Blur = local weighted average • Different set of weights => different blur – e.g.: box, Gaussian, bilinear, bicubic Box filter: Equally-weighted average of N samples CS-C3100 Fall 2019 – Lehtinen 22 Types of Blur • Blur = local weighted average • Different set of weights => different blur – e.g.: box, Gaussian, bilinear, bicubic Bicubic filter: A wider filter with small negative lobes CS-C3100 Fall 2019 – Lehtinen 23 Filter Examples Notice that there are still sharp features in the result Original Box filter CS-C3100 Fall 2019 – Lehtinen 24 Filter Examples Notice that there are still sharp features in the result Gaussian blur Box filter CS-C3100 Fall 2019 – Lehtinen 25 Filter Examples No sharp edges like box, but overall sharper (good) Gaussian blur Bicubic filter CS-C3100 Fall 2019 – Lehtinen 26 Blurring: (Discrete) Convolution Convolution Input sign Kernel Convolution of a function and a kernel gives another function. All values in the output function are locally weighted averages of the input function. CS-C3100 Fall 2019 – Lehtinen 27 Blurring: (Discrete) Convolution Convolution Input sign Kernel Same shape, just reduced contrast!!! The sine wave is an eigenvector (output is the input multiplied by a constant) This means sines and cosines are particularly simple for convolution CS-C3100 Fall 2019 – Lehtinen 28 Animated Convolution • See Wikipedia CS-C3100 Fall 2019 – Lehtinen 29 (Convolutional Neural Nets) • Yes, the CNNs everyone is talking about now are based on precisely this operation. – Many layers of convolutions where the kernels are learned – Layers separated by point-wise nonlinearities (eg. ReLU) • See wikipedia or Goodfellow’s Deep Learning book CS-C3100 Fall 2019 – Lehtinen 30 Types of Convolution • That was discrete; the functions and the kernel were sampled representations defined on a grid • There is also a continuous convolution – input and output functions and the kernel are defined on a continuous domain – weighted sum is replaced by an integral • And actually, a semi-discrete one as well! – Reconstructing a continuous signal from samples is a convolution as well: you convolve the “spiky” samples with a continuous reconstruction kernel CS-C3100 Fall 2019 – Lehtinen 31 Aalto CS-E5520 Spring 2019 – Lehtinen 32 Filter f(x-xj,y-yj) centered at (xj, yj) Aalto CS-E5520 Spring 2019 – Lehtinen 33 Filter f(x-xj,y-yj) centered at (xj, yj) times the underlying signal Aalto CS-E5520 Spring 2019 – Lehtinen 34 dxdy Z value of convolution at (xj,yj) is the integral of the filter times the underlying signal Aalto CS-E5520 Spring 2019 – Lehtinen 35 Low-pass filtered continuous image (convolution of f and input image) = previous integral evaluated at all points Aalto CS-E5520 Spring 2019 – Lehtinen 36 Samples at pixel centers Aalto CS-E5520 Spring 2019 – Lehtinen 37 Samples evaluate convolution result at pixel centers Aalto CS-E5520 Spring 2019 – Lehtinen 38 Example: Samples CS-C3100 Fall 2019 – Lehtinen 39 Example: Reconstruction Kernel CS-C3100 Fall 2019 – Lehtinen 40 1 Sample * Translated Reconstruction Kernel CS-C3100 Fall 2019 – Lehtinen 41 All Samples * Translated Kernels CS-C3100 Fall 2019 – Lehtinen 42 Final Reconstruction = sum of samples * translated kernels CS-C3100 Fall 2019 – Lehtinen 43 Questions? CS-C3100 Fall 2019 – Lehtinen 44 Signal Processing 101 • Sampling and filtering are best understood in terms of Fourier analysis • We already saw aliasing for sine waves: a high frequency sine wave turns into a low frequency one when undersampled CS-C3100 Fall 2019 – Lehtinen 45 Remember Fourier Analysis? • A signal in the spatial domain has a dual representation in the frequency domain spatial domain frequency domain • This particular signal is band-limited, meaning it has no frequencies above some threshold CS-C3100 Fall 2019 – Lehtinen 46 Remember Fourier Analysis? • We can transform from one domain to the other using the Fourier Transform – Just a change of basis: Represent the image in terms of sines and cosines of different frequencies and orientations – An invertible transform frequency domain spatial domain Fourier Transform Inverse Fourier Transform CS-C3100 Fall 2019 – Lehtinen 47 The Fourier basis functions are sines and cosines each with a certain orientation and frequency v u CS-C3100 Fall 2019 – Lehtinen The Fourier basis functions are sines and cosines each with a certain orientation
Details
-
File Typepdf
-
Upload Time-
-
Content LanguagesEnglish
-
Upload UserAnonymous/Not logged-in
-
File Pages111 Page
-
File Size-